Machine Learning Way for Boosting Accuracy in Canonical Correlation Analysis based Frequency Recognition
IJCNN(2006)
摘要
Canonical Correlation Analysis (CCA) is used to frequency recognition of multichannel signals. The unknown signals are compared against known templates and their frequencies are recognized by simply comparing the biggest coefficients of their CCA coefficient vectors. This strategy is straightforward but may not give optimal results. To boost the accuracy of recognition we reformulate the approach in views of machine learning. In this paper, we propose a new strategy based on supervised learning. We also employ feature selection within this framework to adopt efficient coefficients which may not be the largest coefficients for the features vectors. The recognition method is validated by results with real world data.
更多查看译文
关键词
supervised learning,signal processing,learning (artificial intelligence),frequency recognition,multichannel signal,canonical correlation analysis,machine learning,correlation methods,learning artificial intelligence,feature vector,feature selection
AI 理解论文
溯源树
样例
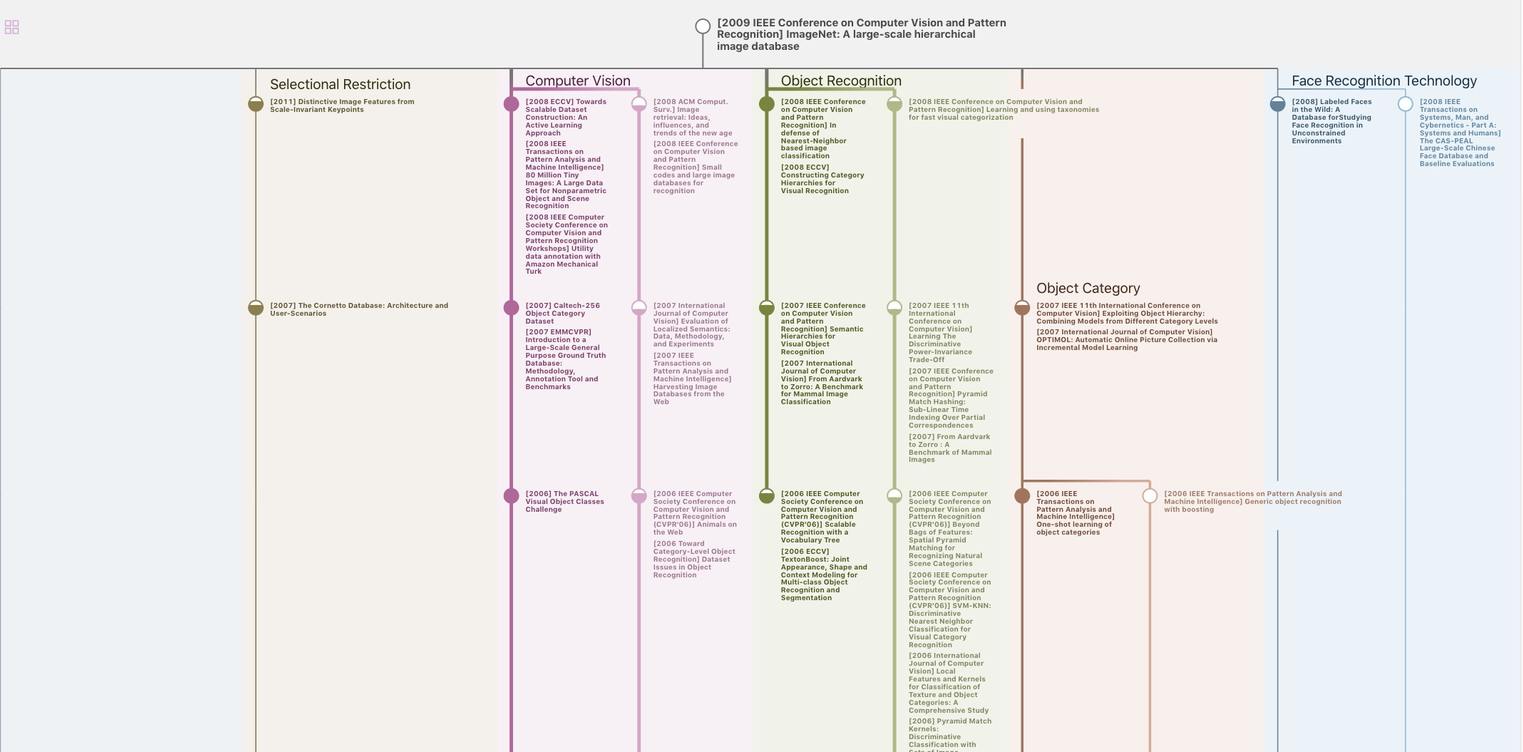
生成溯源树,研究论文发展脉络
Chat Paper
正在生成论文摘要