Multi-Activity Tracking In Lle Body Pose Space
Proceedings of the 2nd conference on Human motion: understanding, modeling, capture and animation(2007)
摘要
We present a method to simultaneously estimate 3d body pose and action categories from monocular video sequences. Our approach learns a low-dimensional embedding of the pose manifolds using Locally Linear Embedding (LLE), as well as the statistical relationship between body poses and their image appearance. In addition, the dynamics in these pose manifolds are modelled. Sparse kernel regressors capture the nonlinearities of these mappings efficiently. Body poses are inferred by a recursive Bayesian sampling algorithm with an activity-switching mechanism based on learned transfer functions. Using a rough foreground segmentation, we compare Binary PCA and distance transforms to encode the appearance. As a postprocessing step, the globally optimal trajectory through the entire sequence is estimated, yielding a single pose estimate per frame that is consistent throughout the sequence. We evaluate the algorithm on challenging sequences with subjects that are alternating between running and walking movements. Our experiments show how the dynamical model helps to track through poorly segmented low-resolution image sequences where tracking otherwise fails, while at the same time reliably classifying the activity type.
更多查看译文
关键词
Image Descriptor, Locally Linear Embedding, Relevance Vector Machine, Nonlinear Dimensionality Reduction, Appearance Descriptor
AI 理解论文
溯源树
样例
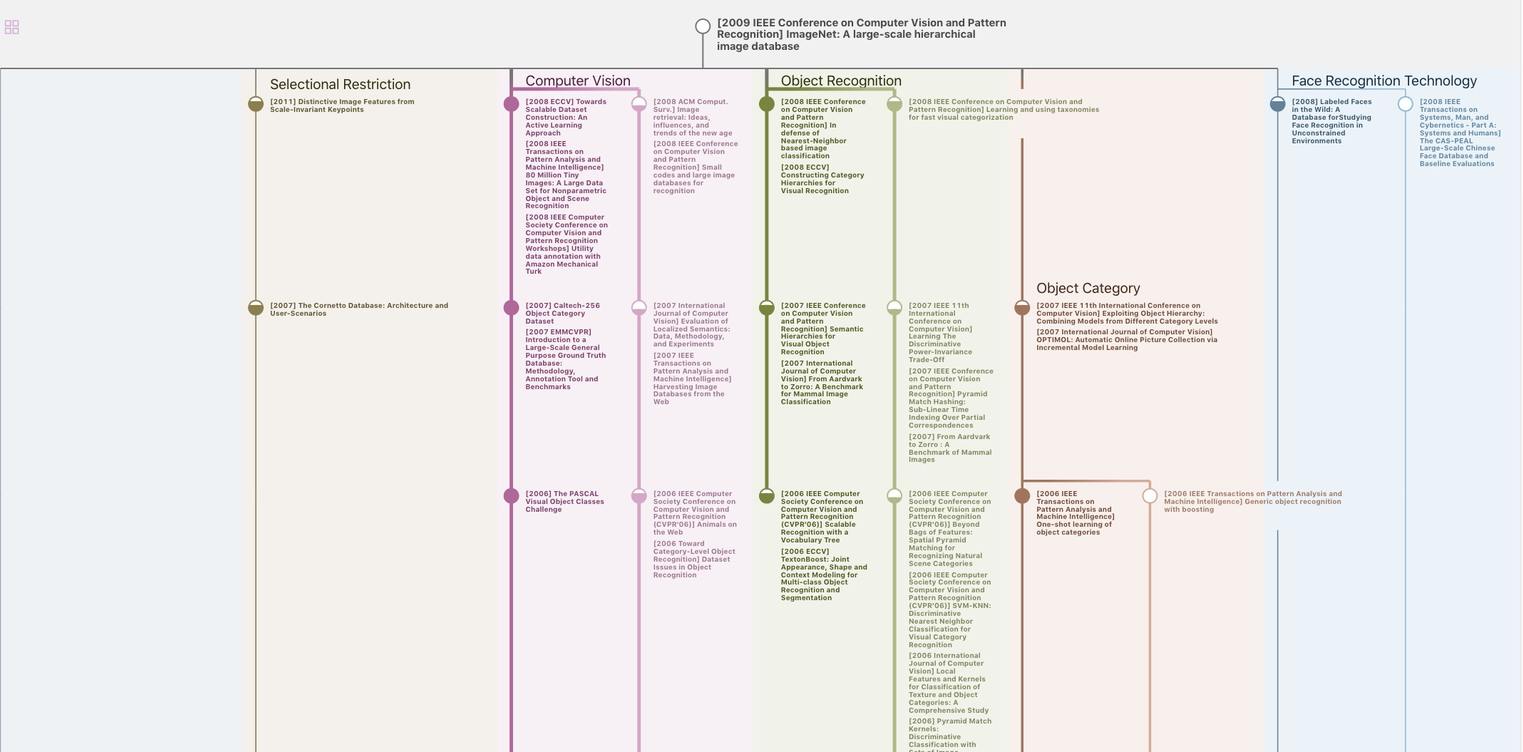
生成溯源树,研究论文发展脉络
Chat Paper
正在生成论文摘要