Learning Mixtures of Localized Rules by Maximizing the Area Under the ROC Curve
ROCAI(2004)
摘要
We introduce a model class for statistical learning which is based on mixtures of propositional rules. In our mixture model, the weight of a rule is not uniform over the entire instance space. Rather, it depends on the instance at hand. This is motivated by applications in molecular biology, where it is frequently observed that the effect of a particular mutational pattern depends on the genetic background in which it occurs. We assume in our model that the effect of a given pattern of mutations will be very similar only among sequences that are also highly similar to each other. On the other hand, a pattern might have very different effects in different genetic backgrounds. Model inference consists of repeated iteration through a sequence of three steps: First, a new rule is mined from a resampled data set using the apriori algorithm. Next, the localization information for the rule is computed. Finally, the weights of all rules in the mixture model are re-optimized simultaneously. This weight optimization is done using the area under the ROC curve rather than the error rate as the objective function. Correspondingly, the weight of a sample in the resampling procedure is based on the rank of the sample relative to the other samples rather than directly on the score itself (such as in boosting). This strategy can be seen as an adaptation of boosting to the case of AUC optimization. Finally, we apply our method to the problem of predicting HIV-1 coreceptor usage from the amino acid sequence of the viral surface protein.
更多查看译文
关键词
molecular biology,amino acid sequence,error rate,roc curve,mixture model,objective function
AI 理解论文
溯源树
样例
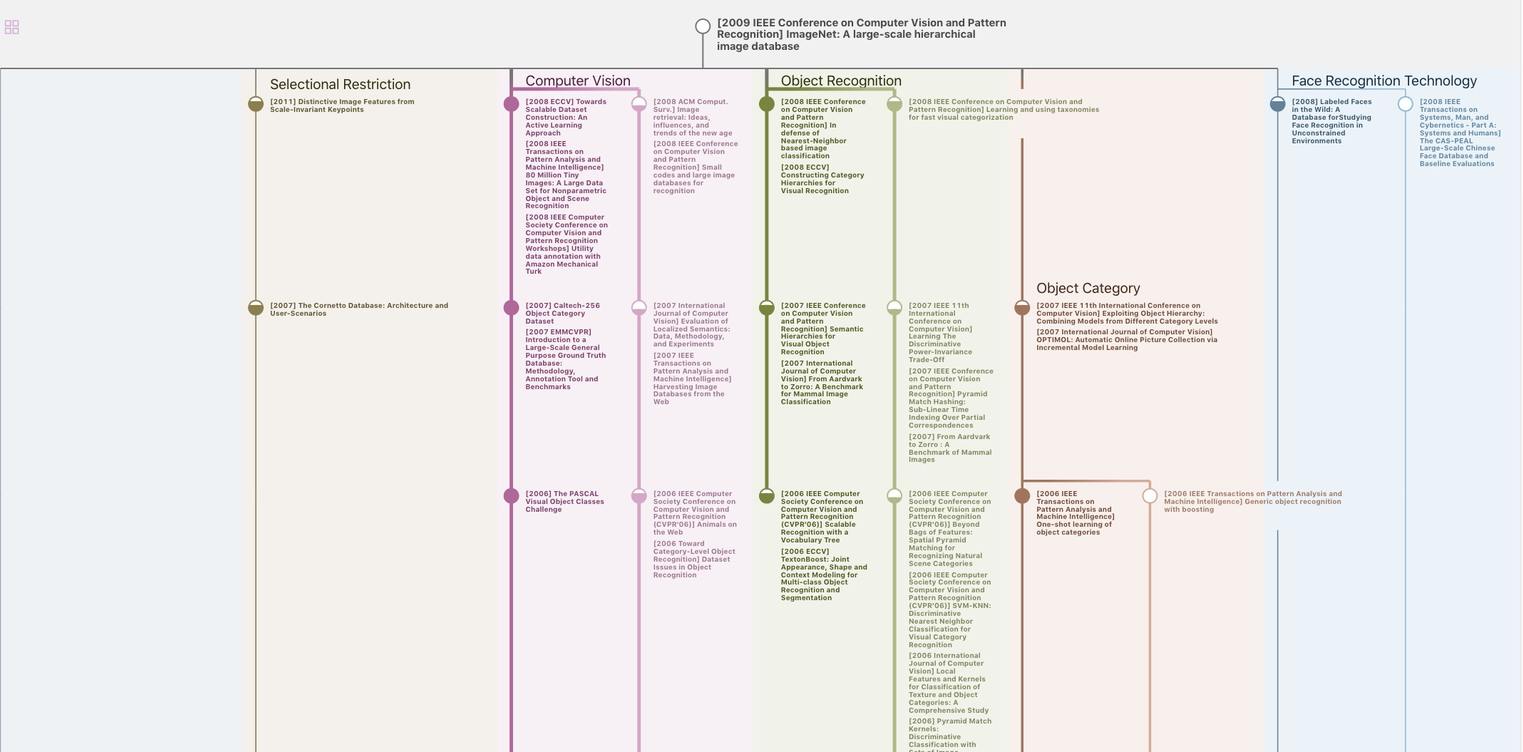
生成溯源树,研究论文发展脉络
Chat Paper
正在生成论文摘要