Discriminative Graph Training For Ultra-Fast Low-Footprint Speech Indexing
INTERSPEECH 2008: 9TH ANNUAL CONFERENCE OF THE INTERNATIONAL SPEECH COMMUNICATION ASSOCIATION 2008, VOLS 1-5(2008)
摘要
We study low complexity models for audio search. The indexing and retrieval system consists of Automatic Speech Recognition (ASR), phone expansion, N-gram indexing and approximate match. In particular, the ASR system can vary tremendously in complexity ranging from a simple speaker-independent system to a fully speaker-adapted system. In this paper, we focus on a speaker-independent system with a small number of Gaussians. Such a system, with ASR followed by phone expansion, provides a good balance between speed and accuracy, allowing for the processing of large volumes of data and better retrieval performance than systems relying solely on phone recognition. Here we describe the use of discriminative training of a finite-state decoding graph for improving system accuracy while preserving speed of operation.
更多查看译文
关键词
speech indexing, discriminative training, finite-state decoding graph
AI 理解论文
溯源树
样例
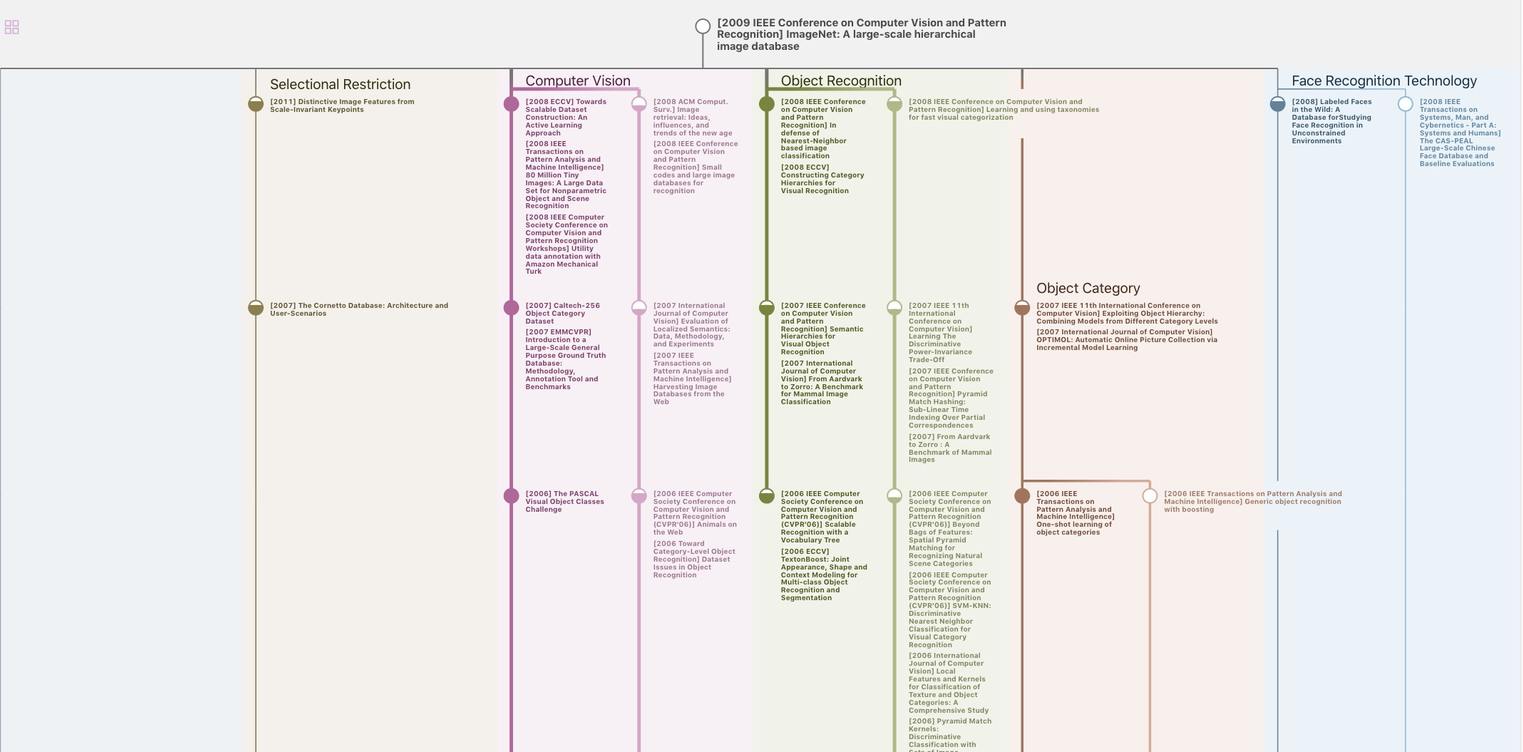
生成溯源树,研究论文发展脉络
Chat Paper
正在生成论文摘要