Probabilistic models for concurrent chatting activity recognition
IJCAI(2011)
摘要
Recognition of chatting activities in social interactions is useful for constructing human social networks. However, the existence of multiple people involved in multiple dialogues presents special challenges. To model the conversational dynamics of concurrent chatting behaviors, this article advocates Factorial Conditional Random Fields (FCRFs) as a model to accommodate co-temporal relationships among multiple activity states. In addition, to avoid the use of inefficient Loopy Belief Propagation (LBP) algorithm, we propose using Iterative Classification Algorithm (ICA) as the inference method for FCRFs. We designed experiments to compare our FCRFs model with two dynamic probabilistic models, Parallel Condition Random Fields (PCRFs) and Hidden Markov Models (HMMs), in learning and decoding based on auditory data. The experimental results show that FCRFs outperform PCRFs and HMMs-like models. We also discover that FCRFs using the ICA inference approach not only improves the recognition accuracy but also takes significantly less time than the LBP inference method.
更多查看译文
关键词
factorial conditional random fields,multiple dialogue,fcrfs model,lbp inference method,activity recognition,multiple activity state,hmms-like model,dynamic probabilistic model,multiple people,ica inference approach,inference method,loopy belief propagation,iterative classification,chatting activity recognition,hidden markov model,conditional random field,social network,social interaction,probabilistic model
AI 理解论文
溯源树
样例
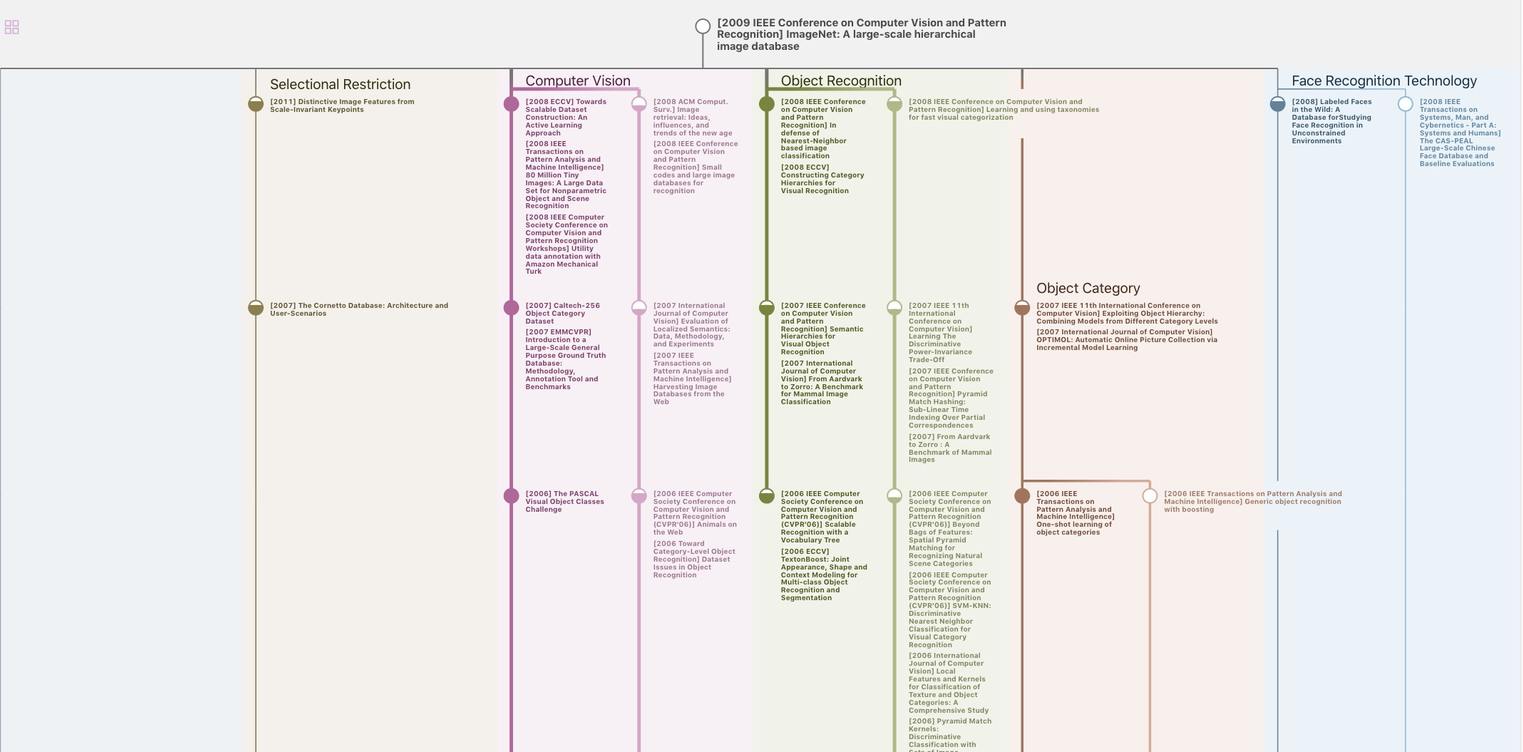
生成溯源树,研究论文发展脉络
Chat Paper
正在生成论文摘要