Beyond Sliding Windows: Object Localization By Efficient Subwindow Search
CVPR(2008)
摘要
Most successful object recognition systems rely on binary classification, deciding only if an object is present or not, but not providing information on the actual object location. To perform localization, one can take a sliding window approach, but this strongly increases the computational cost, because the classifier function has to be evaluated over a large set of candidate subwindows. In this paper, we propose a simple yet powerful branch-and-bound scheme that allows efficient maximization of a large class of classifier functions over all possible subimages. It converges to a globally optimal solution typically in sublinear time. We show how our method is applicable to different object detection and retrieval scenarios. The achieved speedup allows the use of classifiers for localization that formerly were considered too slow for this task, such as SVMs with a spatial pyramid kernel or nearest neighbor classifiers based on the chi(2)-distance. We demonstrate state-of-the-art performance of the resulting systems on the UIUC Cars dataset, the PASCAL VOC 2006 dataset and in the PASCAL VOC 2007 competition.
更多查看译文
关键词
image classification,image retrieval,object detection,tree searching,ESS method,branch-and-bound scheme,efficient subwindow search,object detection,object localization,object recognition systems,object retrieval,sliding windows,subimage classifier functions,
AI 理解论文
溯源树
样例
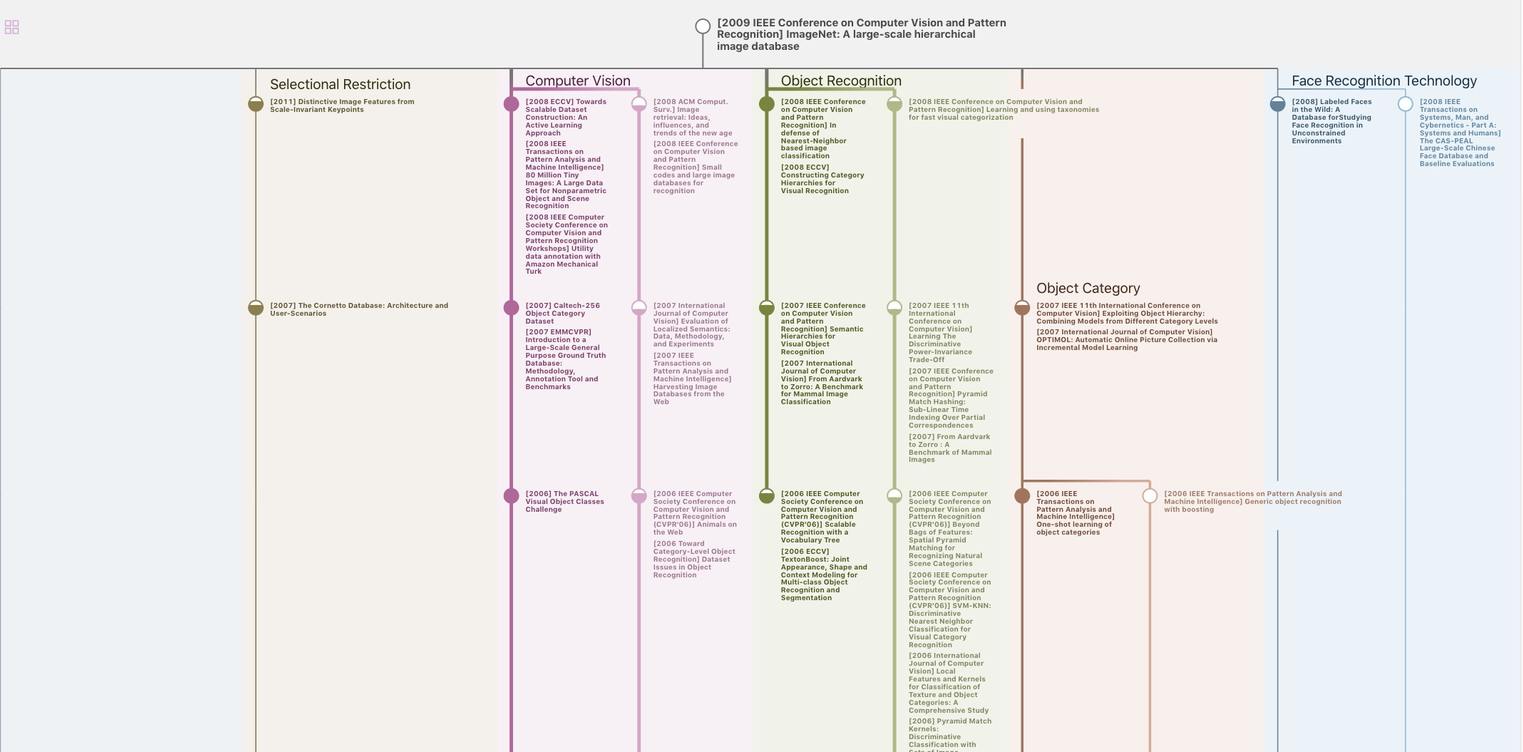
生成溯源树,研究论文发展脉络
Chat Paper
正在生成论文摘要