ALOHA: a novel probability fusion approach for scoring multi-parameter drug-likeness during the lead optimization stage of drug discovery
Journal of Computer-Aided Molecular Design(2013)
摘要
Automated lead optimization helper application (ALOHA) is a novel fitness scoring approach for small molecule lead optimization. ALOHA employs a series of generalized Bayesian models trained from public and proprietary pharmacokinetic, absorption, distribution, metabolism, and excretion, and toxicology data to determine regions of chemical space that are likely to have excellent drug-like properties. The input to ALOHA is a list of molecules, and the output is a set of individual probabilities as well as an overall probability that each of the molecules will pass a panel of user selected assays. In addition to providing a summary of how and when to apply ALOHA, this paper will discuss the validation of ALOHA’s Bayesian models and probability fusion approach. Most notably, ALOHA is demonstrated to discriminate between members of the same chemical series with strong statistical significance, suggesting that ALOHA can be used effectively to select compound candidates for synthesis and progression at the lead optimization stage of drug discovery.
更多查看译文
关键词
Domain of applicability,Model strength,Multi-parameter optimization,Model confidence,Compound fitness,Drug-likeness prediction,Lead optimization
AI 理解论文
溯源树
样例
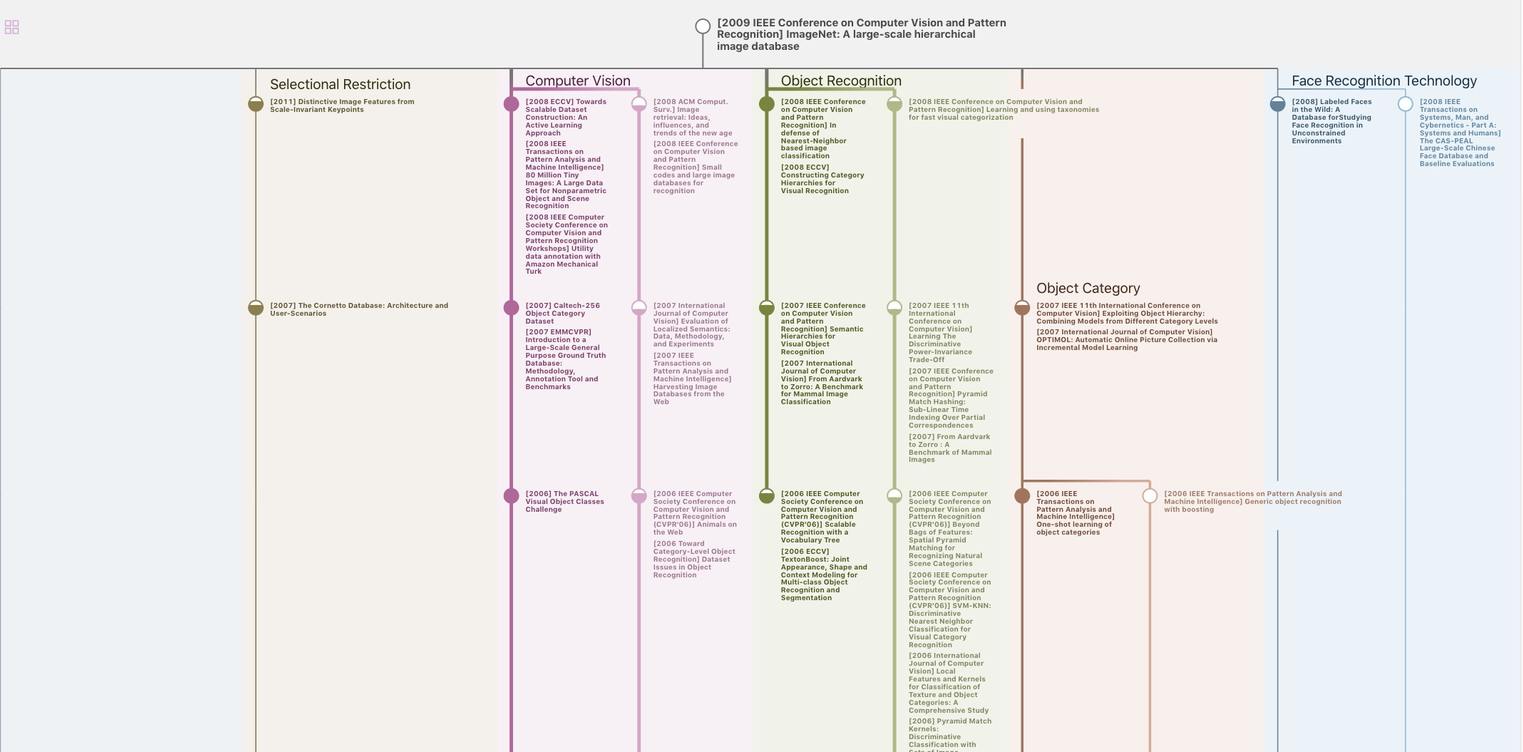
生成溯源树,研究论文发展脉络
Chat Paper
正在生成论文摘要