Modular Combination Of Deep Neural Networks For Acoustic Modeling
14TH ANNUAL CONFERENCE OF THE INTERNATIONAL SPEECH COMMUNICATION ASSOCIATION (INTERSPEECH 2013), VOLS 1-5(2013)
摘要
In this work, we propose a modular combination of two popular applications of neural networks to large-vocabulary continuous speech recognition. First, a deep neural network is trained to extract bottleneck features from frames of mel scale filterbank coefficients. In a similar way as is usually done for GMM/HMM systems, this network is then applied as a nonlinear discriminative feature-space transformation for a hybrid setup where acoustic modeling is performed by a deep belief network. This effectively results in a very large network, where the layers of the bottleneck network are fixed and applied to successive windows of feature frames in a time-delay fashion. We show that bottleneck features improve the recognition performance of DBN/HMM hybrids, and that the modular combination enables the acoustic model to benefit from a larger temporal context. Our architecture is evaluated on a recently released and challenging Tagalog corpus containing conversational telephone speech.
更多查看译文
关键词
Acoustic Modeling, Deep Belief Networks, Deep Bottleneck Features, Large Vocabulary Speech Recognition
AI 理解论文
溯源树
样例
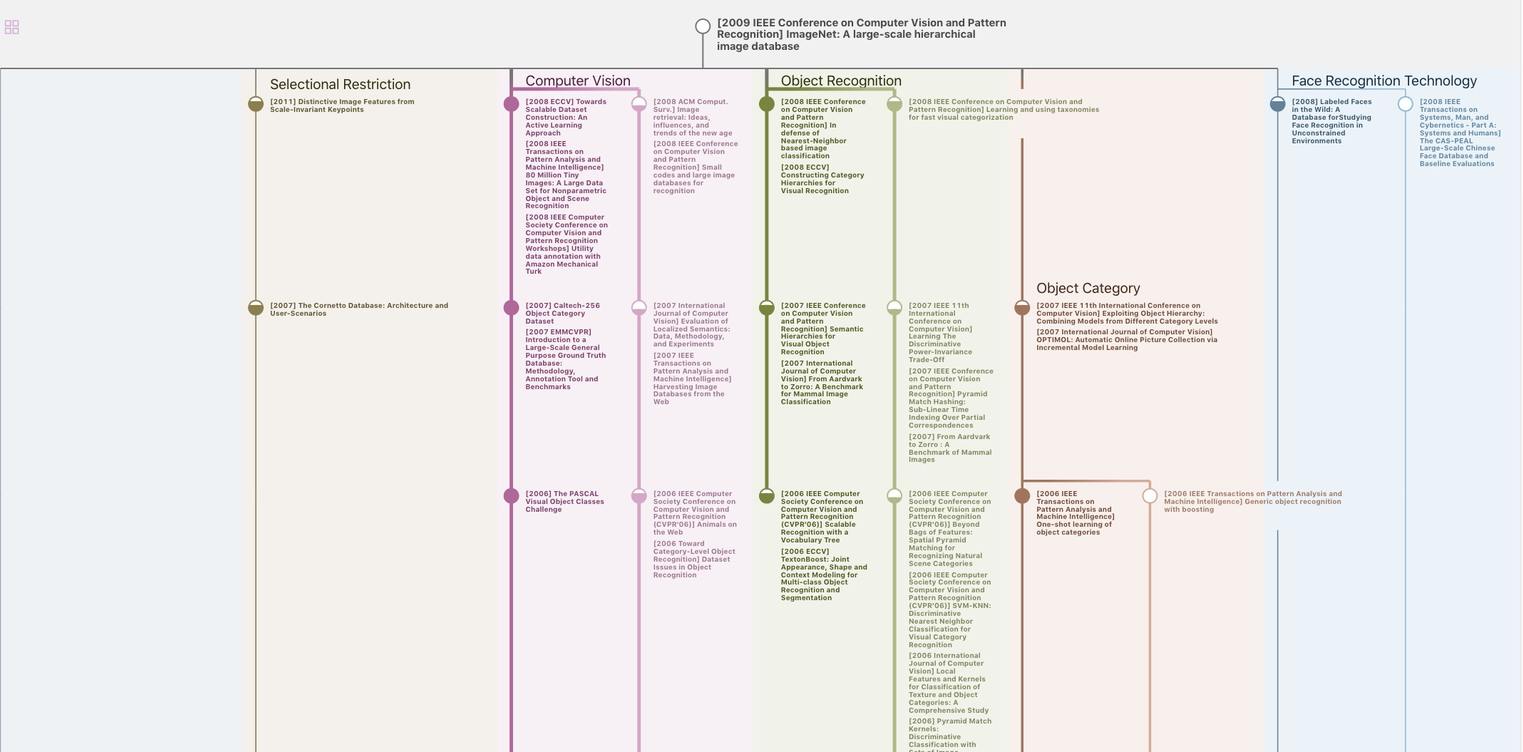
生成溯源树,研究论文发展脉络
Chat Paper
正在生成论文摘要