How to put it into words - using random forests to extract symbol level descriptions from audio content for concept detection
ICASSP(2012)
摘要
This paper presents a system that uses symbolic representations of audio concepts as words for the descriptions of audio tracks, that enable it to go beyond the state of the art, which is audio event classification of a small number of audio classes in constrained settings, to large-scale classification in the wild. These audio words might be less meaningful for an annotator but they are descriptive for computer algorithms. We devise a random-forest vocabulary learning method with an audio word weighting scheme based on TF-IDF and TD-IDD, so as to combine the computational simplicity and accurate multi-class classification of the random forest with the data-driven discriminative power of the TF-IDF/TD-IDD methods. The proposed random forest clustering with text-retrieval methods significantly outperforms two state-of-the-art methods on the dry-run set and the full set of the TRECVID MED 2010 dataset.
更多查看译文
关键词
accurate multiclass classification,audio classification,pattern clustering,multimedia event detection,random forest clustering,inverse document,computational simplicity,audio concepts,vocabulary,audio track descriptions,extract symbol level descriptions,text detection,term frequency,audio word weighting,annotator,symbolic representations,random-forest vocabulary learning,random forests,text-retrieval,audio signal processing,audio content,audio event classification,trecvid med 2010 dataset,frequency,large-scale classification,concept detection,radio frequency,vegetation,support vector machines
AI 理解论文
溯源树
样例
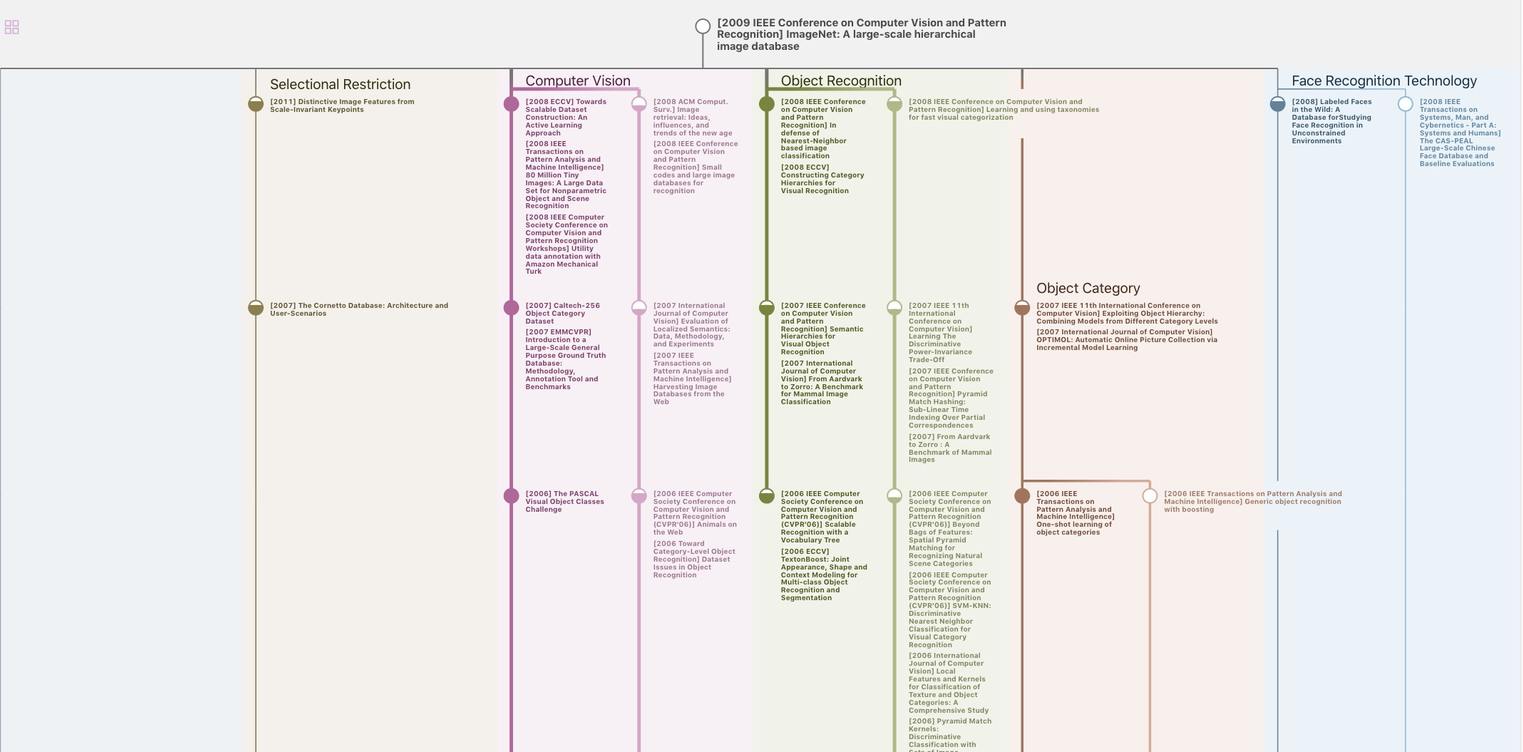
生成溯源树,研究论文发展脉络
Chat Paper
正在生成论文摘要