Aftershock ground motion prediction model based on conditional convolutional generative adversarial networks
Engineering Applications of Artificial Intelligence(2024)
摘要
Strong mainshocks are typically accompanied by numerous aftershocks, and the investigation of the structural failure mechanisms under the mainshock-aftershock sequence becomes particularly crucial. However, the number of recorded mainshock-aftershock sequences is limited. Therefore, the purpose of this article is to provide a reasonable method for directly generating the aftershock time histories from mainshock time histories. Using convolutional network as the basic network layer and conditional generative adversarial network as the structure, two models, one-dimensional convolution (1D-C-DCGAN) and two-dimensional convolution (2D-C-DCGAN) are established respectively by utilizing the deep convolutional generative adversarial network to learn the relationship between the mainshock-aftershock time histories. Then, they are trained with 972 pairs of the selected mainshock-aftershock time histories, and prediction results are discussed in comparison. The results show that the two models are proficient in generating AS acceleration time histories that are closely related to the sample trend, in which the 2D-C-DCGAN model performing better in overall waveform prediction, but with local spikes. In the comparison of intensity measures and response spectra, by examining coefficients such as R2, RMSE, MAPE, the two models outperformed the mainstream model (ASK14) on the dataset, and the 2D-C-DCGAN model is more accurate than the 1D-C-DCGAN model. The distributions of intensity measure predicted by 2D-C-DCGAN model are closer to the measured intensity measures, and its predicted response spectra are smoother and better matched to the measured response spectra. This advantage can be attributed to the effectiveness of convolution operations on two-dimensional data, allowing the convolutional capabilities to be fully utilized.
更多查看译文
关键词
Aftershocks,Seismic time history,Intensity measures,Conditional generative adversarial network,Convolutional neural network
AI 理解论文
溯源树
样例
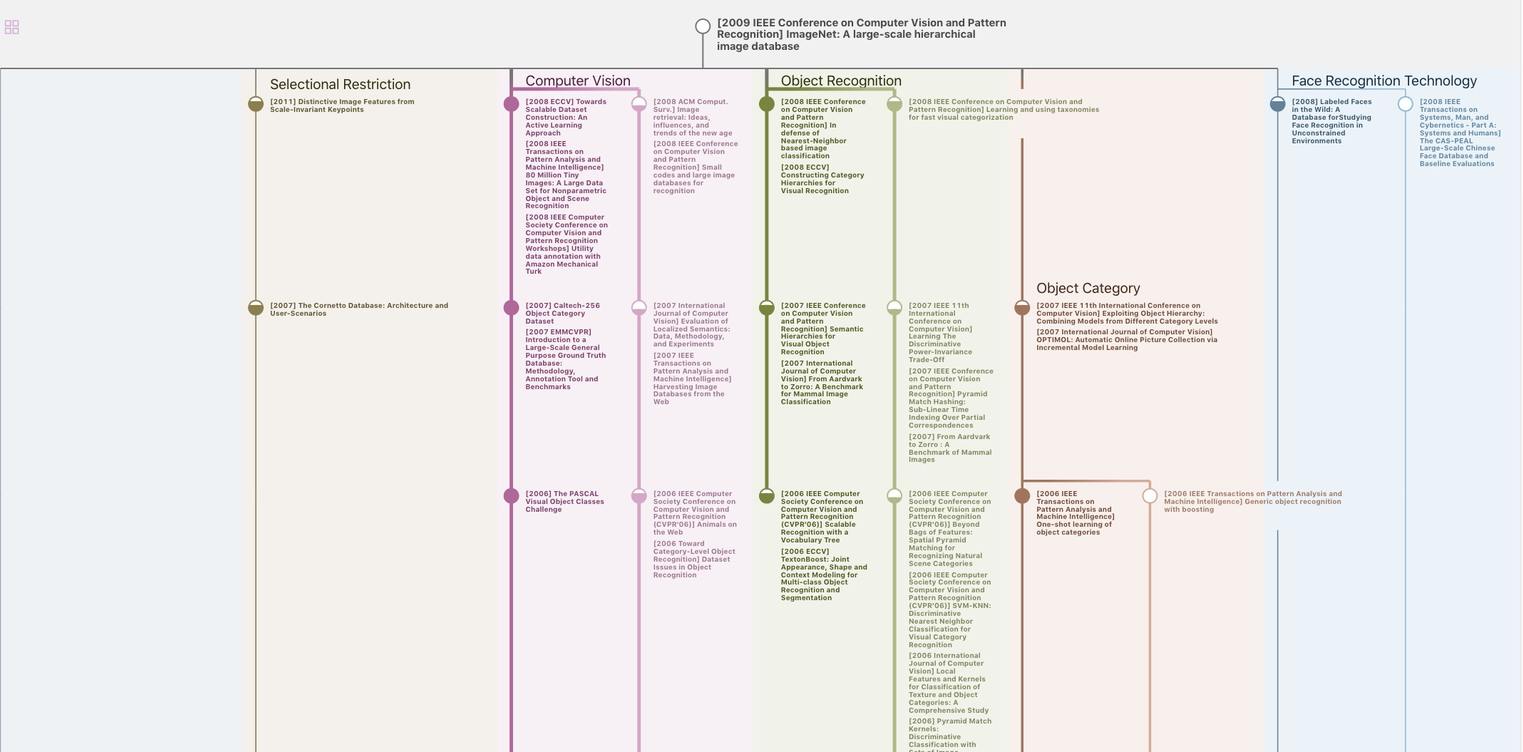
生成溯源树,研究论文发展脉络
Chat Paper
正在生成论文摘要