Support Vector Machine Approximation using Kernel PCA
msra(2009)
摘要
Support Vector Machine is a very important tech- nique used for classification and regression. Although very accurate, the speed of SVM classification de- creases with increase in the number of support vec- tors. This paper describes one method of reducing the number of support vectors through the application of Kernel PCA. This method is different from other pro- posed methods as we show that the exact choice of the reduced support vectors is not important as long as the vectors span a fixed subspace. This method reduces the number of support vectors by upto 90% without any significant degradation in performance. We also propose a heuristic to determine the reducibility of an SVM.
更多查看译文
关键词
support vector,kernel pca,support vector machine
AI 理解论文
溯源树
样例
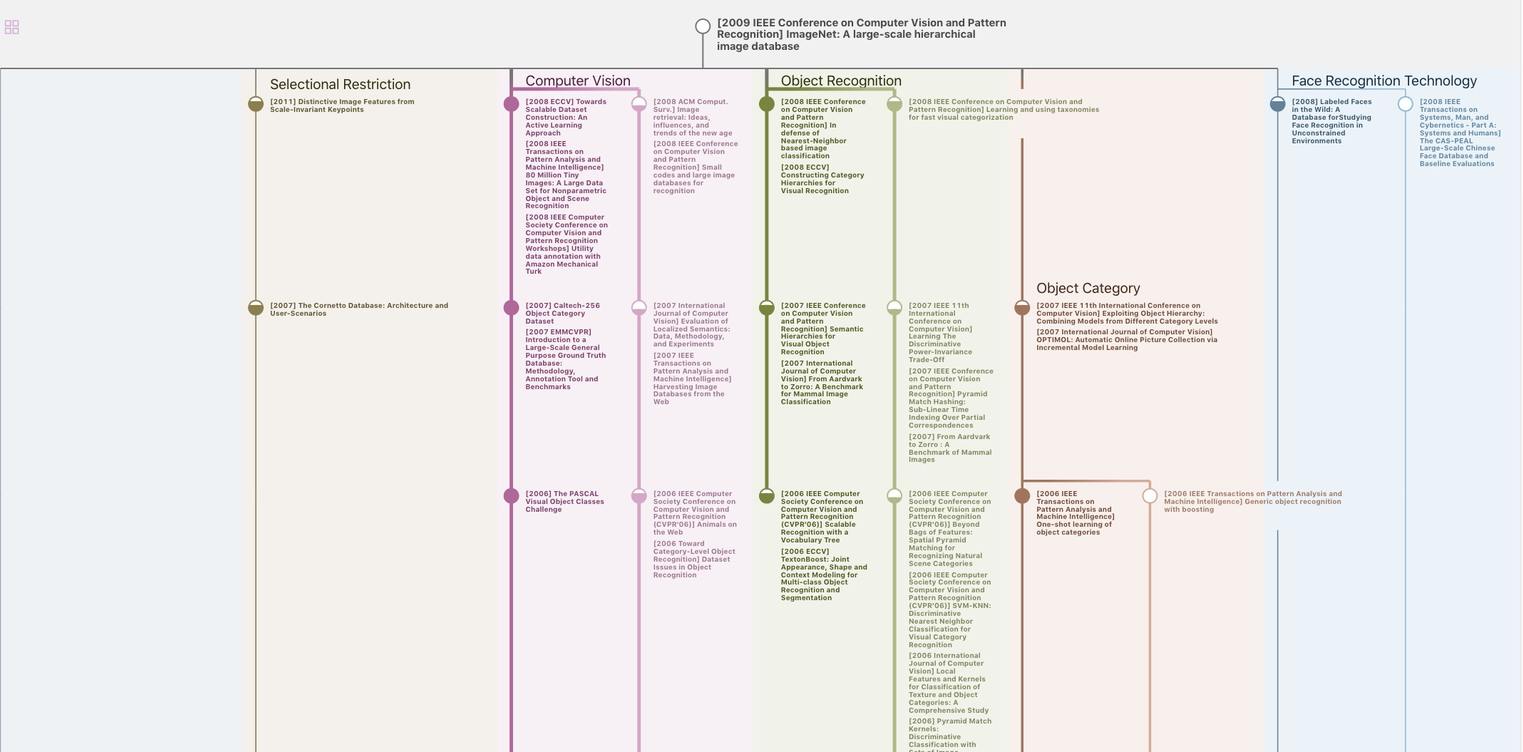
生成溯源树,研究论文发展脉络
Chat Paper
正在生成论文摘要