Hourly Traffic Prediction of News Stories
arXiv (Cornell University)(2013)
摘要
The process of predicting news stories popularity from several news sources has become a challenge of great importance for both news producers and readers. In this paper, we investigate methods for automatically predicting the number of clicks on a news story during one hour. Our approach is a combination of additive regression and bagging applied over a M5P regression tree using a logarithmic scale (log10). The features included are social-based (social network metadata from Facebook), content-based (automatically extracted keyphrases, and stylometric statistics from news titles), and time-based. In 1st Sapo Data Challenge we obtained 11.99% as mean relative error value which put us in the 4th place out of 26 participants.
更多查看译文
关键词
prediction,news stories
AI 理解论文
溯源树
样例
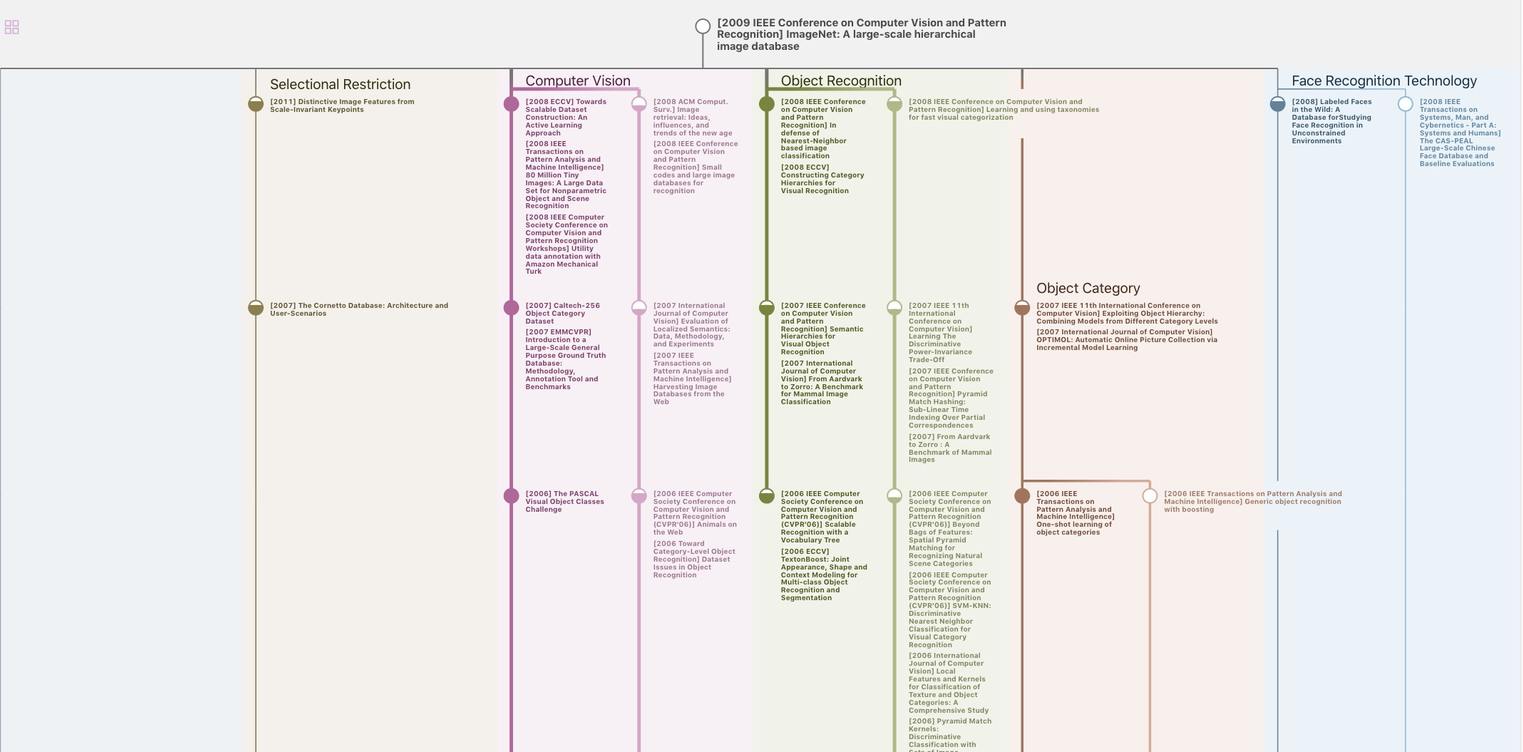
生成溯源树,研究论文发展脉络
Chat Paper
正在生成论文摘要