Robust Keyword Spotting With Rapidly Adapting Point Process Models
INTERSPEECH 2009: 10TH ANNUAL CONFERENCE OF THE INTERNATIONAL SPEECH COMMUNICATION ASSOCIATION 2009, VOLS 1-5(2009)
摘要
In this paper, we investigate the noise robustness properties of frame-based and sparse point process-based models for spotting keywords in continuous speech. We introduce a new strategy to improve point process model (PPM) robustness by adapting low-level feature detector thresholds to preserve background firing rates in the presence of noise. We find that this unsupervised approach can significantly outperform fully supervised maximum likelihood linear regression (MLLR) adaptation of an equivalent keyword-filler HMM system in the presence of additive white and pink noise. Moreover, we find that the sparsity of PPMs introduces an inherent resilience to non-stationary babble noise not exhibited by the frame-based HMM system. Finally, we demonstrate that our approach requires less adaptation data than MLLR, permitting rapid online adaptation.
更多查看译文
关键词
keyword spotting, point process model, noise adaptation
AI 理解论文
溯源树
样例
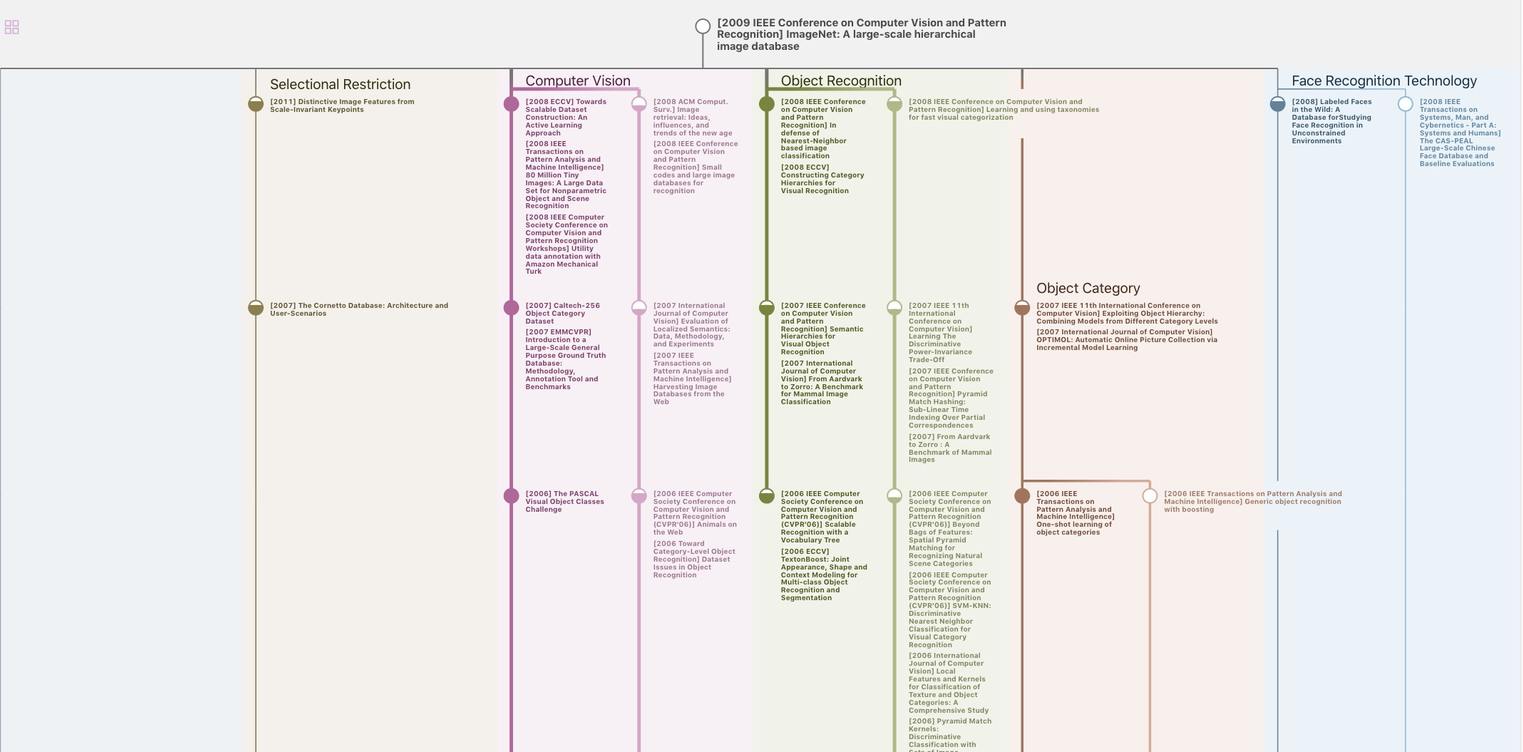
生成溯源树,研究论文发展脉络
Chat Paper
正在生成论文摘要