Understanding large text corpora via sparse machine learning
Periodicals(2013)
摘要
AbstractSparse machine learning has recently emerged as powerful tool to obtain models of high-dimensional data with high degree of interpretability, at low computational cost. The approach has been successfully used in many areas, such as signal and image processing. This article posits that these methods can be extremely useful in the analysis of large collections of text documents, without requiring user expertise in machine learning. Our approach relies on three main ingredients: i multidocument text summarization; ii comparative summarization of two corpora, both using sparse regression or classification; iii sparse principal components and sparse graphical models for unsupervised analysis and visualization of large text corpora. We validate our methods using a corpus of Aviation Safety Reporting System ASRS reports and demonstrate that the methods can reveal causal and contributing factors in runway incursions. Furthermore, we show that the methods automatically discover four main tasks that pilots perform during flight, which can aid in further understanding the causal and contributing factors to runway incursions and other drivers for aviation safety incidents. We also provide a comparative study involving other commonly used datasets, and report on the competitiveness of sparse machine learning compared to state-of-the-art methods such as latent Dirichlet allocation LDA. © 2013 Wiley Periodicals, Inc. Statistical Analysis and Data Mining 6: 221-242, 2013
更多查看译文
关键词
data mining,intelligent text understanding,sparse machine learning,optimization
AI 理解论文
溯源树
样例
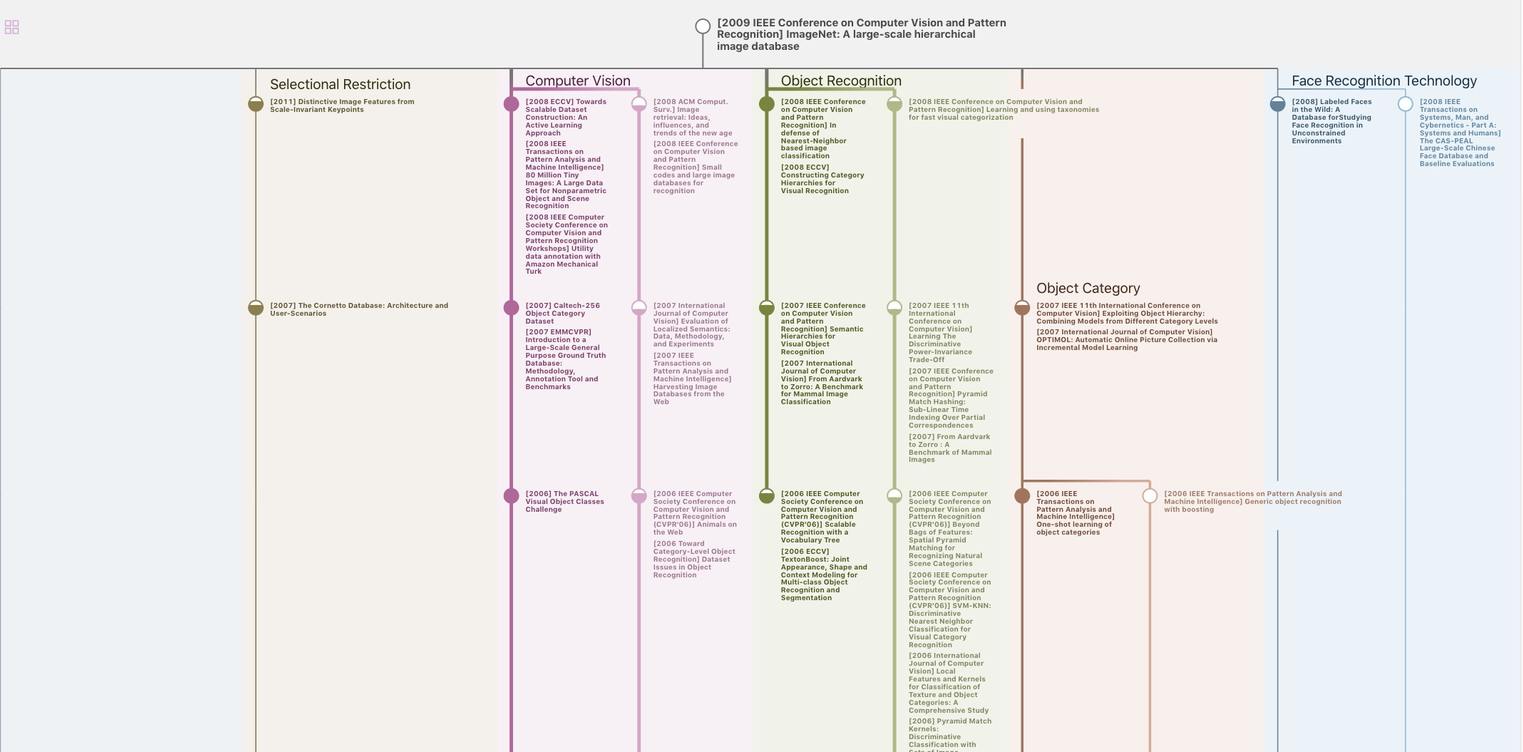
生成溯源树,研究论文发展脉络
Chat Paper
正在生成论文摘要