Topics Over Nonparametric Time: A Supervised Topic Model Using Bayesian Nonparametric Density Estimation.
BMAW'12: Proceedings of the Ninth UAI Conference on Bayesian Modeling Applications Workshop - Volume 962(2012)
摘要
We propose a new supervised topic model that uses a nonparametric density estimator to model the distribution of real-valued metadata given a topic. The model is similar to Topics Over Time, but replaces the beta distributions used in that model with a Dirichlet process mixture of normals. The use of a nonparametric density estimator allows for the fitting of a greater class of metadata densities. We compare our model with existing supervised topic models in terms of prediction and show that it is capable of discovering complex metadata distributions in both synthetic and real data.
更多查看译文
AI 理解论文
溯源树
样例
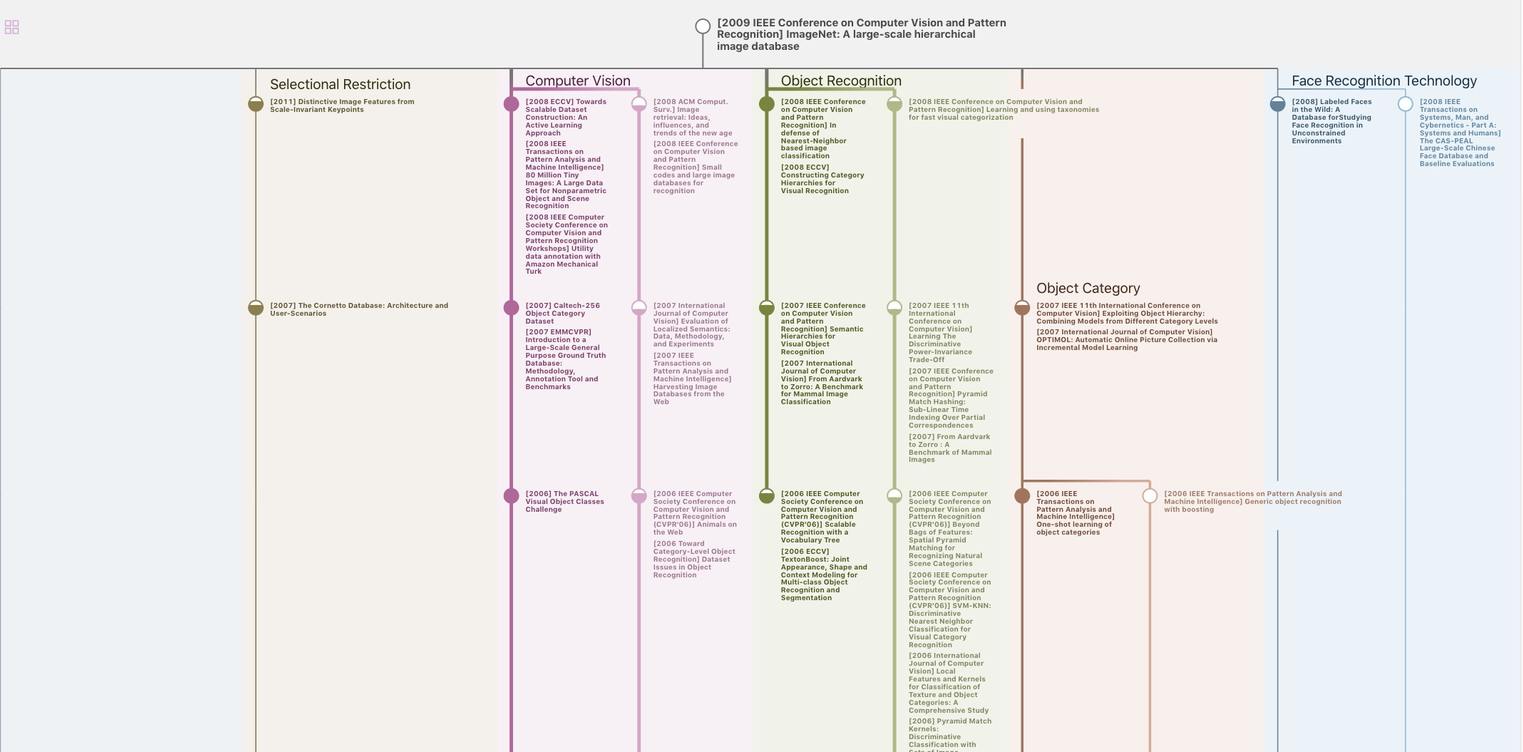
生成溯源树,研究论文发展脉络
Chat Paper
正在生成论文摘要