Training Factored PCFGs with Expectation Propagation
EMNLP-CoNLL '12: Proceedings of the 2012 Joint Conference on Empirical Methods in Natural Language Processing and Computational Natural Language Learning(2012)
摘要
PCFGs can grow exponentially as additional annotations are added to an initially simple base grammar. We present an approach where multiple annotations coexist, but in a factored manner that avoids this combinatorial explosion. Our method works with linguistically-motivated annotations, induced latent structure, lexicalization, or any mix of the three. We use a structured expectation propagation algorithm that makes use of the factored structure in two ways. First, by partitioning the factors, it speeds up parsing exponentially over the unfactored approach. Second, it minimizes the redundancy of the factors during training, improving accuracy over an independent approach. Using purely latent variable annotations, we can efficiently train and parse with up to 8 latent bits per symbol, achieving F1 scores up to 88.4 on the Penn Treebank while using two orders of magnitudes fewer parameters compared to the naïve approach. Combining latent, lexicalized, and unlexicalized annotations, our best parser gets 89.4 F1 on all sentences from section 23 of the Penn Treebank.
更多查看译文
关键词
Penn Treebank,multiple annotations coexist,Combining latent,independent approach,induced latent structure,latent bit,latent variable annotation,unfactored approach,F1 score,factored manner,expectation propagation,factored PCFGs
AI 理解论文
溯源树
样例
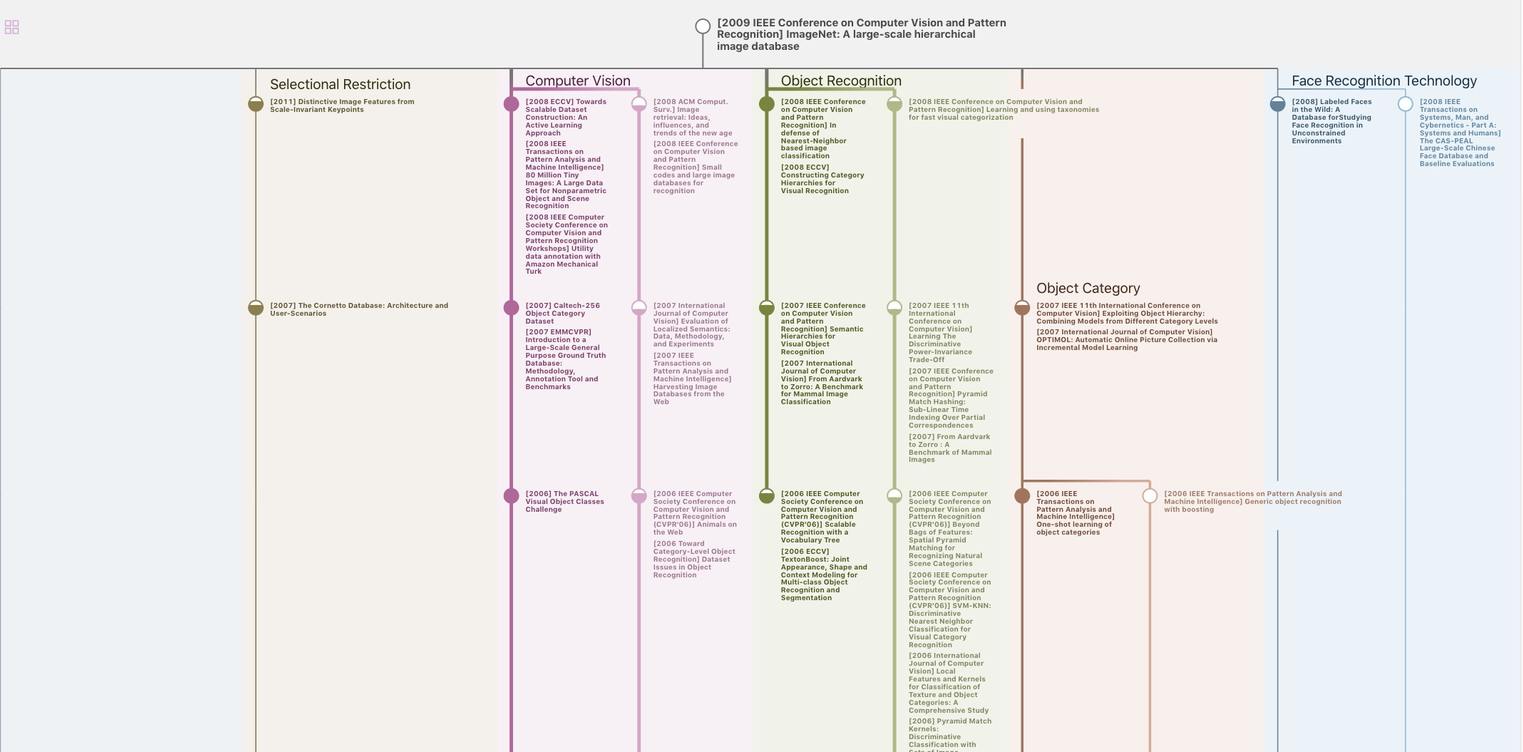
生成溯源树,研究论文发展脉络
Chat Paper
正在生成论文摘要