Federated learning for heart segmentation
2022 IEEE 14th Image, Video, and Multidimensional Signal Processing Workshop (IVMSP)(2022)
摘要
Training deep learning models for medical imaging requires access to large volumes of sensitive patient data. To this end, the models are generally trained on centralized, de-identified databases that are hard to collect because of privacy requirements. Federated learning proposes an alternative approach, in which a coalition of hospitals collaboratively trains a central model without exchanging any clinical data. This paper explores the combination of federated learning with U-Net models, and applies it to the task of image segmentation of the heart. A variant of federated learning referred to as federated equal-chances that improves segmentation performance on unbalanced datasets is introduced as well.
更多查看译文
关键词
federated learning,U-net,image segmentation,federated equal-chances,heart segmentation,deep learning,sensitive patient data,privacy requirements,medical imaging,hospitals
AI 理解论文
溯源树
样例
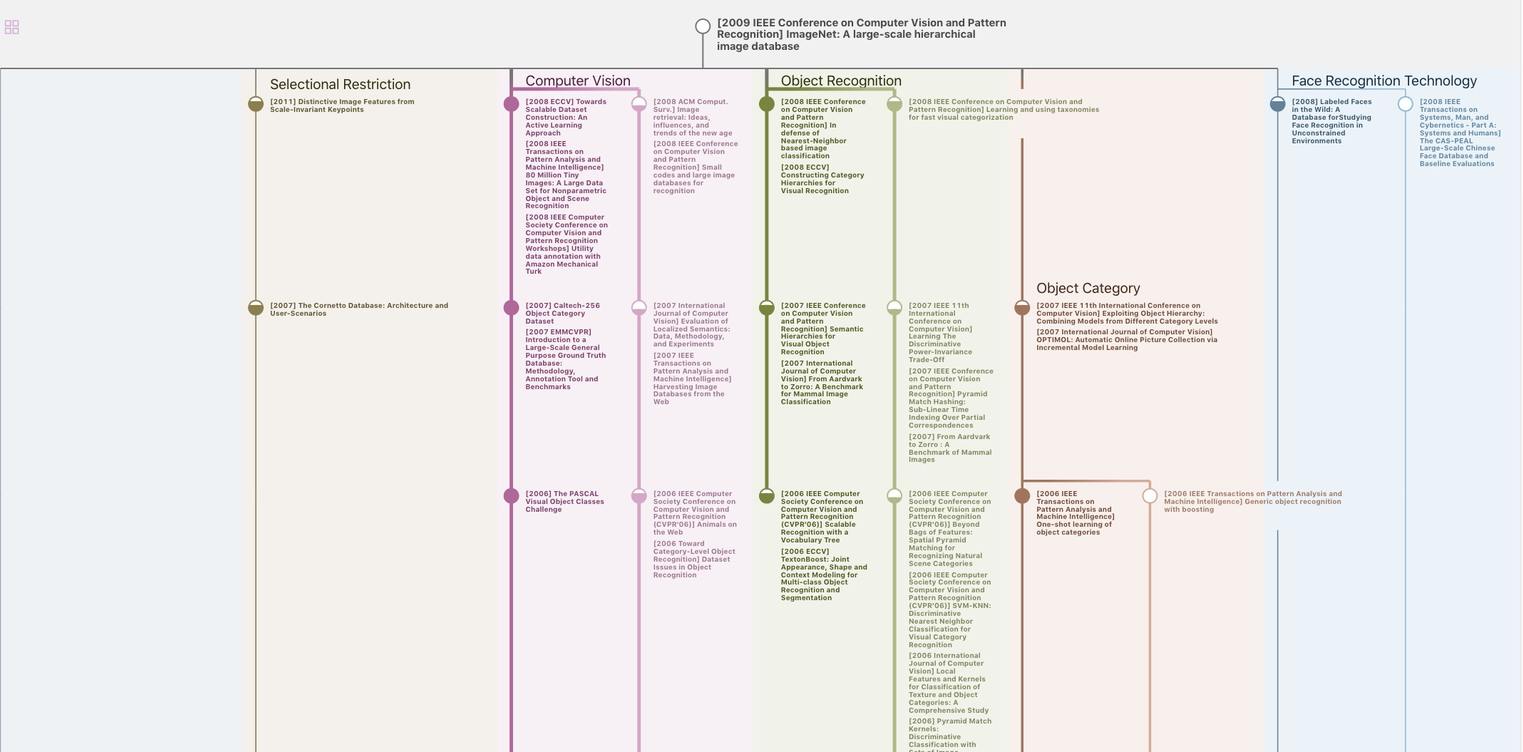
生成溯源树,研究论文发展脉络
Chat Paper
正在生成论文摘要