Bayesian Nonexhaustive Learning for Online Discovery and Modeling of Emerging Classes
ICML(2012)
摘要
We present a framework for online inference in the presence of a nonexhaustively defined set of classes that incorporates supervised classification with class discovery and modeling. A Dirichlet process prior (DPP) model defined over class distributions ensures that both known and unknown class distributions originate according to a common base distribution. In an attempt to automatically discover potentially interesting class formations, the prior model is coupled with a suitably chosen data model, and sequential Monte Carlo sampling is used to perform online inference. Our research is driven by a biodetection application, where a new class of pathogen may suddenly appear, and the rapid increase in the number of samples originating from this class indicates the onset of an outbreak.
更多查看译文
AI 理解论文
溯源树
样例
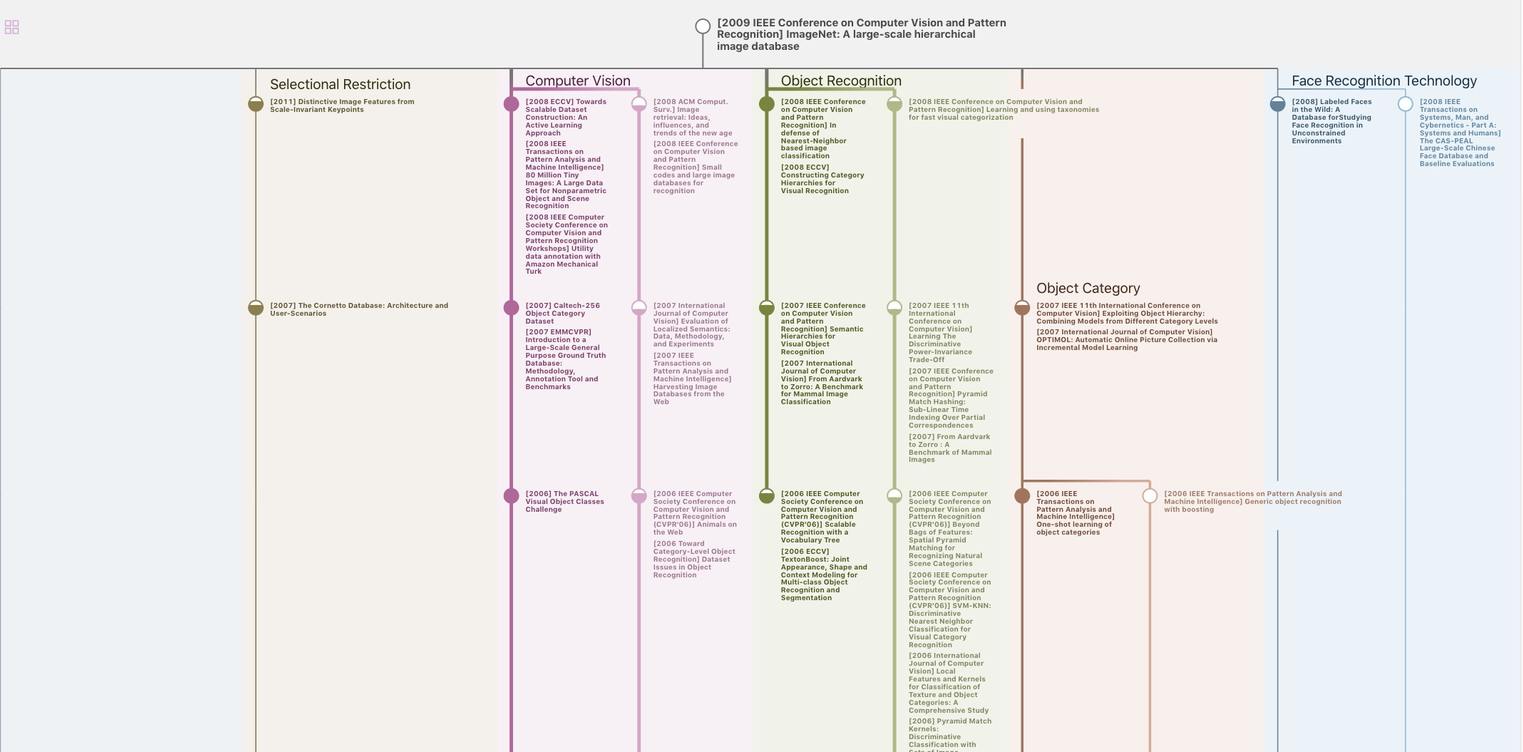
生成溯源树,研究论文发展脉络
Chat Paper
正在生成论文摘要