Coupling detection and data association for multiple object tracking
CVPR(2012)
摘要
We present a novel framework for multiple object tracking in which the problems of object detection and data association are expressed by a single objective function. The framework follows the Lagrange dual decomposition strategy, taking advantage of the often complementary nature of the two subproblems. Our coupling formulation avoids the problem of error propagation from which traditional “detection-tracking approaches” to multiple object tracking suffer. We also eschew common heuristics such as “nonmaximum suppression” of hypotheses by modeling the joint image likelihood as opposed to applying independent likelihood assumptions. Our coupling algorithm is guaranteed to converge and can handle partial or even complete occlusions. Furthermore, our method does not have any severe scalability issues but can process hundreds of frames at the same time. Our experiments involve challenging, notably distinct datasets and demonstrate that our method can achieve results comparable to those of state-of-art approaches, even without a heavily trained object detector.
更多查看译文
关键词
Lagrange dual decomposition strategy,coupling algorithm,coupling formulation,novel framework,object detection,coupling detection,joint image likelihood,independent likelihood assumption,data association,object detector,multiple object tracking,complementary nature
AI 理解论文
溯源树
样例
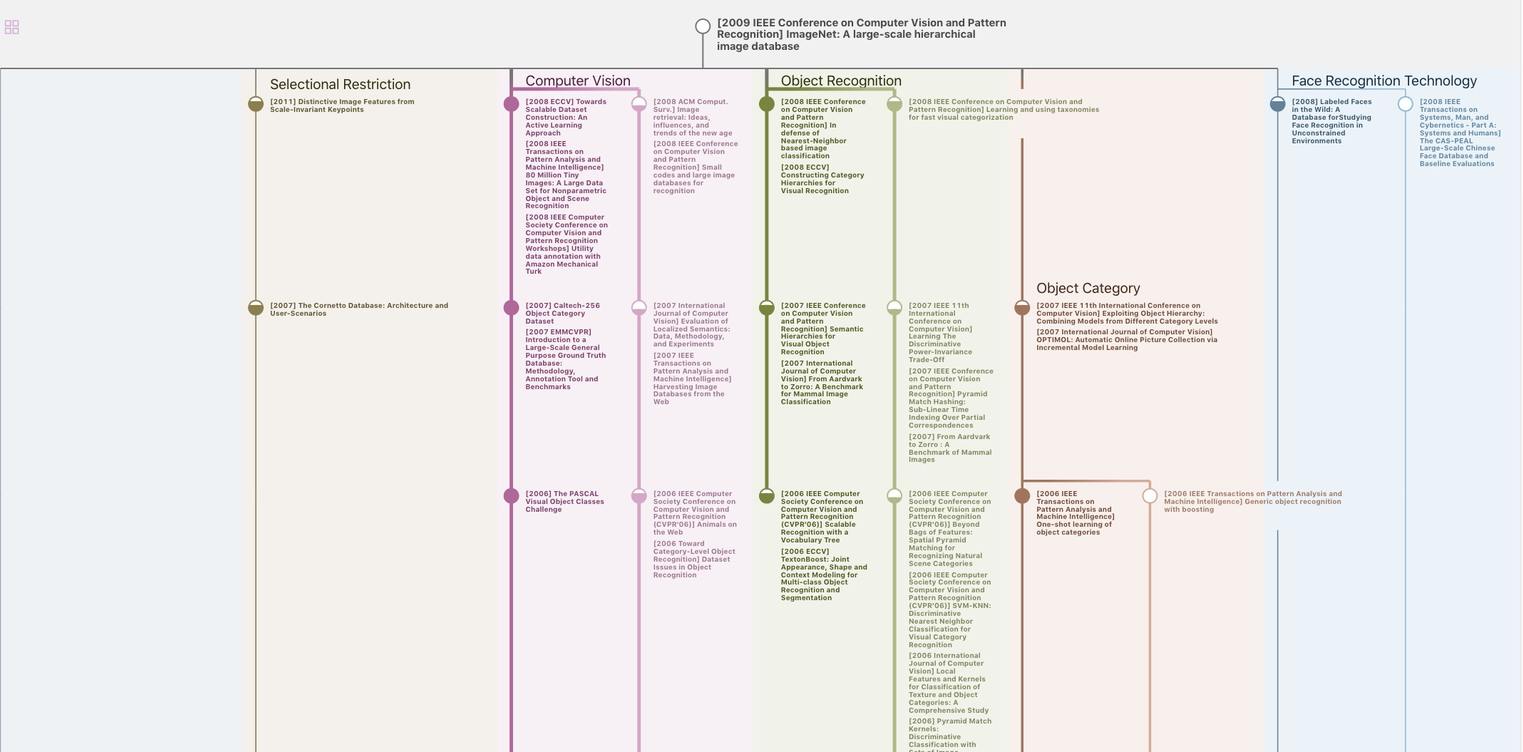
生成溯源树,研究论文发展脉络
Chat Paper
正在生成论文摘要