On the Ensemble of Collision Perception Neuron Models Towards Ultra-Selectivity
2023 INTERNATIONAL JOINT CONFERENCE ON NEURAL NETWORKS, IJCNN(2023)
摘要
This paper investigates the coordination of multiple looming sensitive neuronal models to get closer to the ultra-selectivity represented by organisms, i.e., responding to merely approaching objects. In locust's brain, a group of lobula giant movement detectors (LGMDs) cooperate very well in collision perception. Although the existing single neuron computation can portray individual LGMDs relatively well, it seems to be insufficient to handle all complex situations as stimuli like receding and translating still greatly stimulate the models. Compounding is a method of biological problem-solving, therefore, this paper conjectures that there is signal deepening between LGMDs. We propose a composite model by deepening the forward dendritic circuitry of existing LGMD models. Two types of ensemble herein are investigated with which the LGMD1 cascaded with LGMD2 is named LGMD1-LGMD2, and the LGMD2 cascaded with LGMD1 is named LGMD2-LGMD1. We carry out systematic experiments to test our hypothesis. The results demonstrate that compared with the single neuron models, the proposed composite model features extreme looming selectivity to only approaching objects over other categories of movements. Specifically, the selectivity of either LGMD1 or LGMD2 has been mutually enhanced built upon their original selectivity, i.e., the LGMD1LGMD2 ensemble is sensitive to approaching white and dark objects, not to any receding or translating stimuli; while the LGMD2-LGMD1 ensemble only responds to approaching dark objects. Accordingly, this research provides a novel case study on the ensemble of motion perception neuronal models to achieve stronger selectivity that a single neuron model cannot accomplish.
更多查看译文
关键词
Looming perception,Ultra-selectivity,Neural ensemble,Mutual enhancement,LGMD
AI 理解论文
溯源树
样例
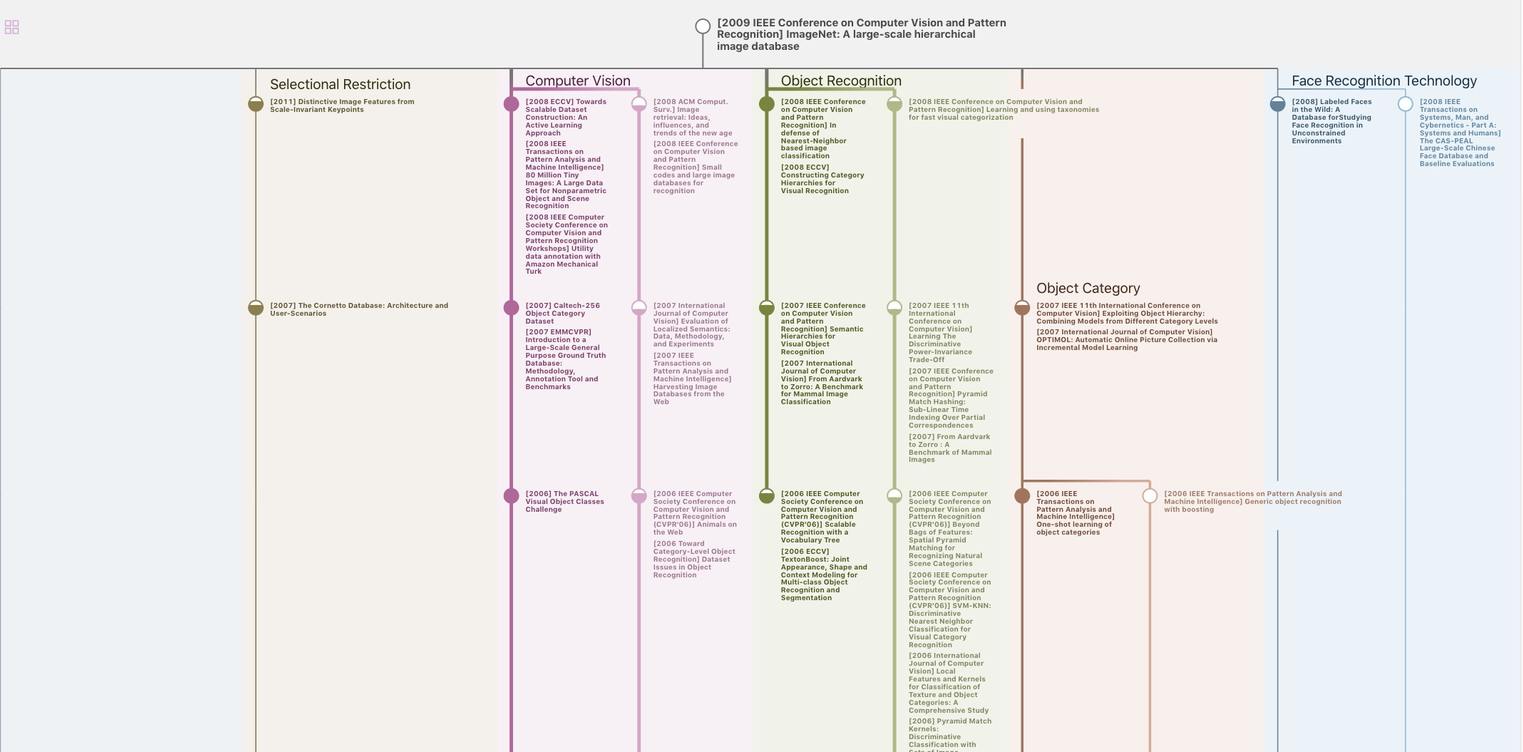
生成溯源树,研究论文发展脉络
Chat Paper
正在生成论文摘要