Optimal Sampling In State Space Models With Applications To Network Monitoring
SIGMETRICS08: ACM SIGMETRICS International Conference on Measurement and Modeling of Computer Systems Annapolis MD USA June, 2008(2008)
摘要
Advances in networking technology have enabled network engineers to use sampled data from routers to estimate network flow volumes and track them over time. However, low sampling rates result in large noise in traffic volume estimates. We propose to combine data on individual flows obtained front sampling with highly aggregate data obtained front SNMP measurements (similar to those used in network tomography) for the tracking problem at hand. Specifically, we introduce a linearized state space model for the estimation of network traffic flow volumes from combined SNMP and sampled data. Further, we formulate the problem of obtaining optimal sampling rates under router resource constraints as all experiment design problem. Theoretically it corresponds to the problem of optimal design for estimation of conditional means for state space models and we present the associated convex programs for a simple approach to it. The usefulness of the approach in the context of network monitoring is illustrated through all extensive numerical study.
更多查看译文
关键词
Internet Traffic Matrix Estimation,Kalman Filtering,State Space Models,Optimal Design of Experiments
AI 理解论文
溯源树
样例
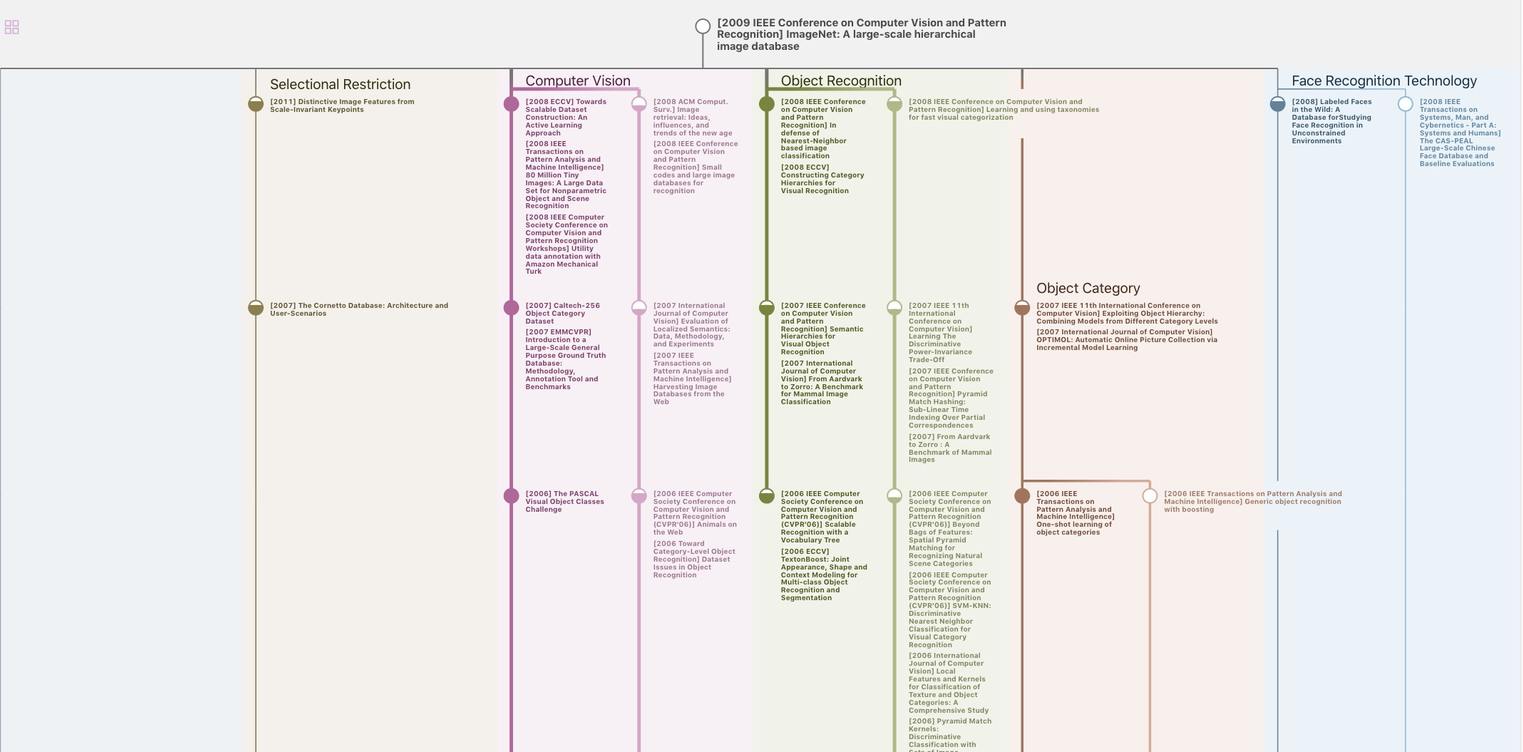
生成溯源树,研究论文发展脉络
Chat Paper
正在生成论文摘要