Reduction Of Truncation Artifact In Stationary Inverse-Geometry Digital Tomosynthesis Using Convolutional Neural Network
MEDICAL IMAGING 2020: PHYSICS OF MEDICAL IMAGING(2021)
摘要
Stationary inverse-geometry digital tomosynthesis (s-IGDT) has advantages in terms of motion artifact reduction and diagnostic efficiency improvement. However, truncation artifacts are caused in reconstructed images owing to the geometric characteristics of s-IGDT systems, and this drawback degrades the diagnostic accuracy. In order to overcome this limitation, we proposed a convolutional neural network (CNN)-based truncation artifact reduction method. We simulated a s-IGDT system with stationary X-ray source array and small detector. Also, we acquired s-IGDT images using 70 volumetric phantoms based on the SPIE-AAPM lung CT challenge dataset. The U-Net was used as the CNN architecture, and we trained the network through 207 s-IGDT images. We confirmed that the truncation artifacts with various patterns included in the prior images were clearly removed in the prediction images obtained by the trained network. Moreover, the quantitative evaluation showed that both of the structural similarity index measure (SSIM) and peak signal-to-noise ratio (PSNR) improved when using the proposed method. The averaged SSIM and PSNR of the prediction images were approximately 6% and 25% higher than those of the prior images, respectively. In conclusion, the proposed model based on the CNN has superior performance in removing the truncation artifacts of s-IGDT images.
更多查看译文
关键词
Stationary inverse-geometry digital tomosynthesis, convolutional neural network, truncation artifact, artifact reduction
AI 理解论文
溯源树
样例
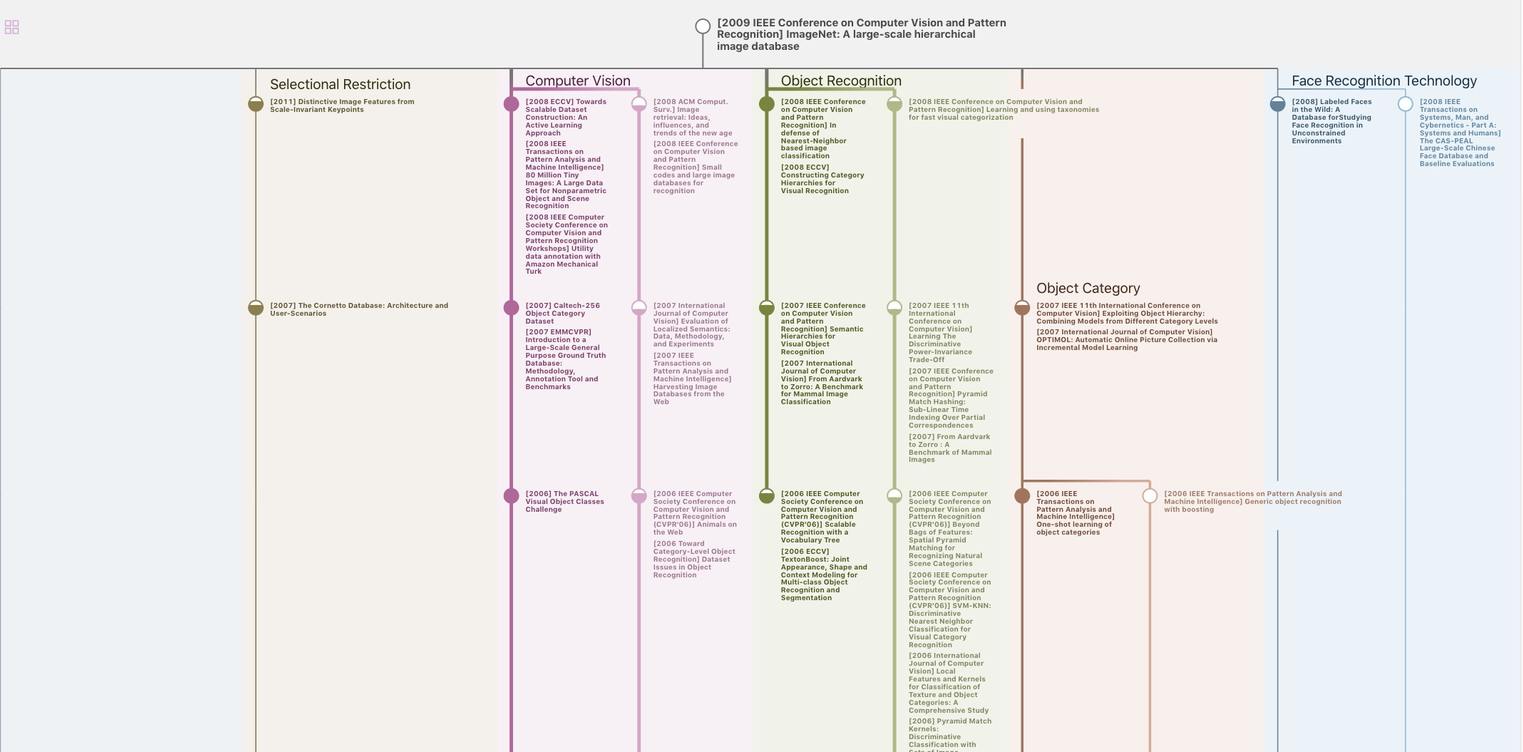
生成溯源树,研究论文发展脉络
Chat Paper
正在生成论文摘要