Semi-supervised learning for scalable and robust visual search
ACM SIGMultimedia Records(2011)
摘要
AbstractUnlike textual document retrieval, searching of visual data is still far from satisfactory. There exist major gaps between the available solutions and practical needs in both accuracy and computational cost. This thesis aims at the development of robust and scalable solutions for visual search and retrieval. Specifically, we investigate two classes of approaches: graph-based semi-supervised learning and hashing techniques. The graph-based approaches are used to improve accuracy, while hashing approaches are used to improve efficiency and cope with large-scale applications. A common theme shared between these two subareas of our work is the focus on semi-supervised learning paradigm, in which a small set of labeled data is complemented with large unlabeled datasets.
更多查看译文
关键词
semi-supervised learning,bivariate graph transduction,hash function,efficient solution,semi-supervised hash function,graph-based approach,general semi-supervised task,large-scale application,available solution,visual data,rigorous semi-supervised paradigm,robust visual search,large unlabeled datasets,visual search,textual document retrieval,computational cost,semi-supervised learning paradigm,common theme,graph-based semi-supervised learning,document retrieval,semi supervised learning,information science,computer science
AI 理解论文
溯源树
样例
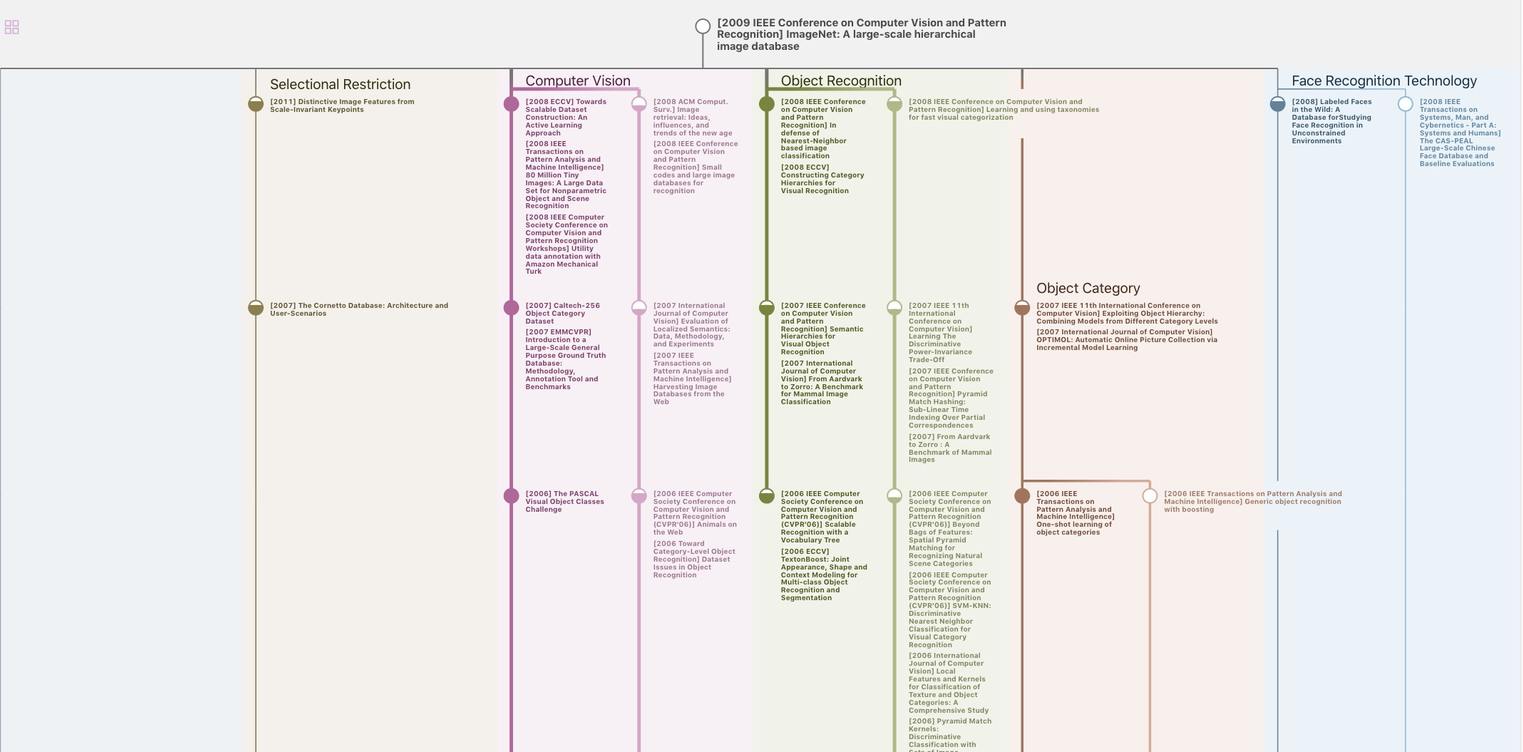
生成溯源树,研究论文发展脉络
Chat Paper
正在生成论文摘要