Two connectionist models for graph processing: an experimental comparison on relational data
mining and learning with graphs(2006)
摘要
In this paper, two recently developed connectionist models for lear- ning from relational or graph-structured data, i.e. Relational Neural Networks (RelNNs) and Graph Neural Networks (GNNs), are compared. We first introduce a general paradigm for connectionist learning from graphs that covers both ap- proaches, and situate the approaches in this general paradigm. This gives a first view on how they relate to each other. As RelNNs have been developed with learning aggregate functions in mind, we compare them to GNNs for this spe- cific task. Next, we compare both with other relational learners on a number of benchmark problems (mutagenesis, biodegradability). The results are promising and suggest that RelNNs and GNNs can be a viable approach for learning on relational data. They also point out a number of differences in behavior between both approaches that deserve further study.
更多查看译文
AI 理解论文
溯源树
样例
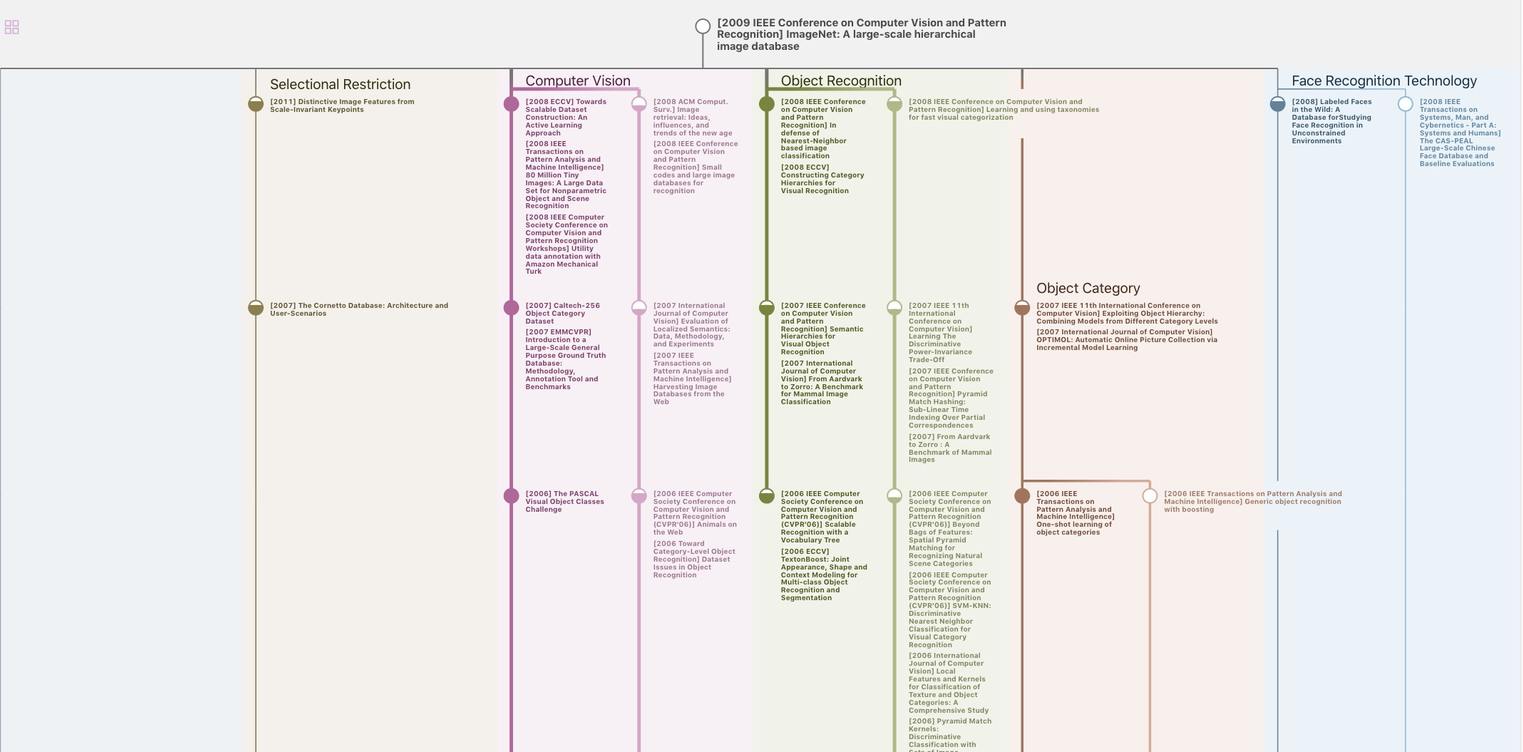
生成溯源树,研究论文发展脉络
Chat Paper
正在生成论文摘要