ResDTA: Predicting Drug-Target Binding Affinity Using Residual Skip Connections
arxiv(2023)
摘要
The discovery of novel drug target (DT) interactions is an important step in the drug development process. The majority of computer techniques for predicting DT interactions have focused on binary classification, with the goal of determining whether or not a DT pair interacts. Protein ligand interactions, on the other hand, assume a continuous range of binding strength values, also known as binding affinity, and forecasting this value remains a difficulty. As the amount of affinity data in DT knowledge-bases grows, advanced learning techniques such as deep learning architectures can be used to predict binding affinities. In this paper, we present a deep-learning-based methodology for predicting DT binding affinities using just sequencing information from both targets and drugs. The results show that the proposed deep learning-based model that uses the 1D representations of targets and drugs is an effective approach for drug target binding affinity prediction and it does not require additional chemical domain knowledge to work with. The model in which high-level representations of a drug and a target are constructed via CNNs that uses residual skip connections and also with an additional stream to create a high-level combined representation of the drug-target pair achieved the best Concordance Index (CI) performance in one of the largest benchmark datasets, outperforming the recent state-of-the-art method AttentionDTA and many other machine-learning and deep-learning based baseline methods for DT binding affinity prediction that uses the 1D representations of targets and drugs.
更多查看译文
AI 理解论文
溯源树
样例
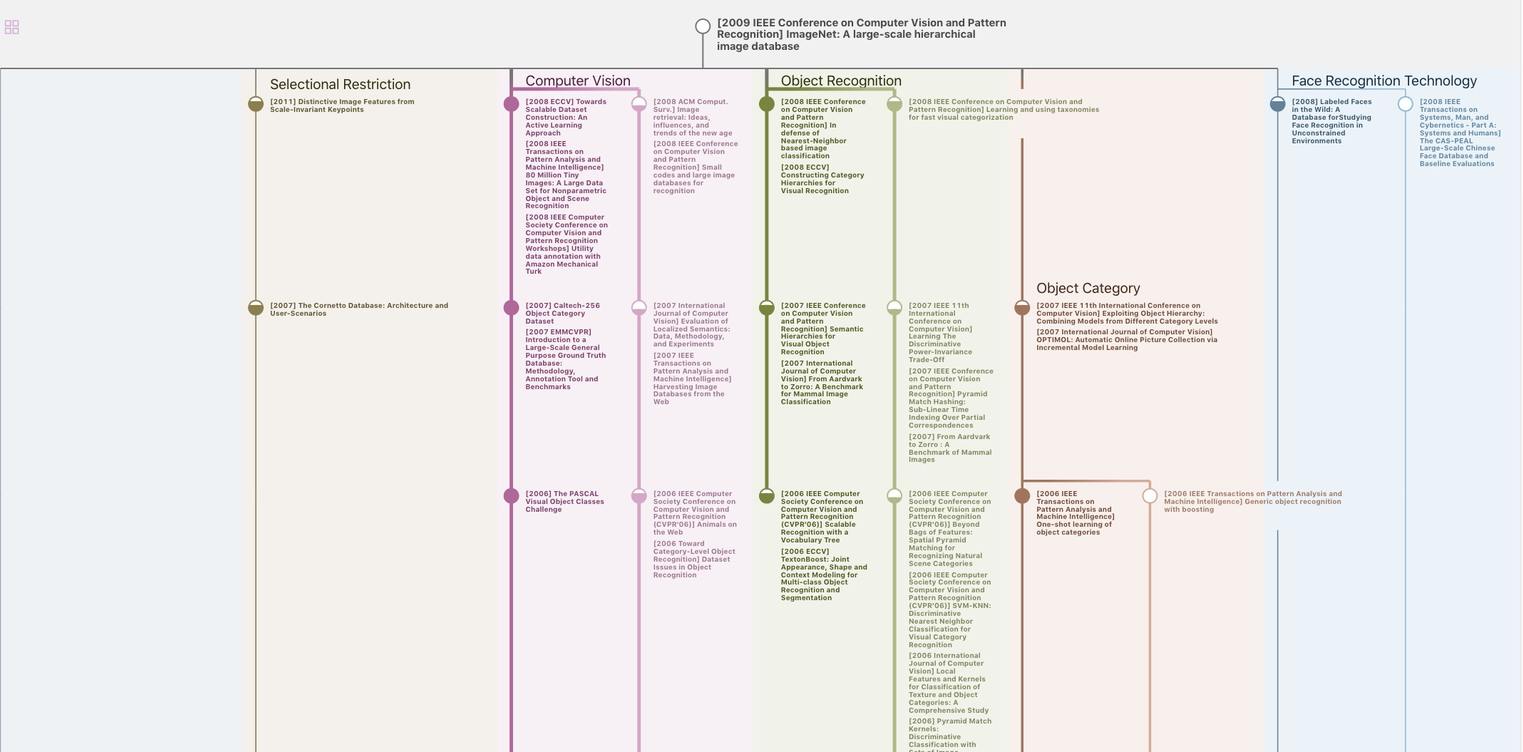
生成溯源树,研究论文发展脉络
Chat Paper
正在生成论文摘要