Unsupervised Discovery Of Object Classes In 3d Outdoor Scenarios
2011 IEEE INTERNATIONAL CONFERENCE ON COMPUTER VISION WORKSHOPS (ICCV WORKSHOPS)(2011)
摘要
Designing object models for a robot's detection-system can be very time-consuming since many object classes exist. This paper presents an approach that automatically infers object classes from recorded 3D data and collects training examples. A special focus is put on difficult unstructured outdoor scenarios with object classes ranging from cars over trees to buildings. In contrast to many existing works, it is not assumed that perfect segmentation of the scene is possible. Instead, a novel hierarchical segmentation method is proposed that works together with a novel inference strategy to infer object classes.
更多查看译文
关键词
unsupervised learning,geometry,vectors,nickel,three dimensional,principal component analysis,clustering algorithms,image segmentation,object model
AI 理解论文
溯源树
样例
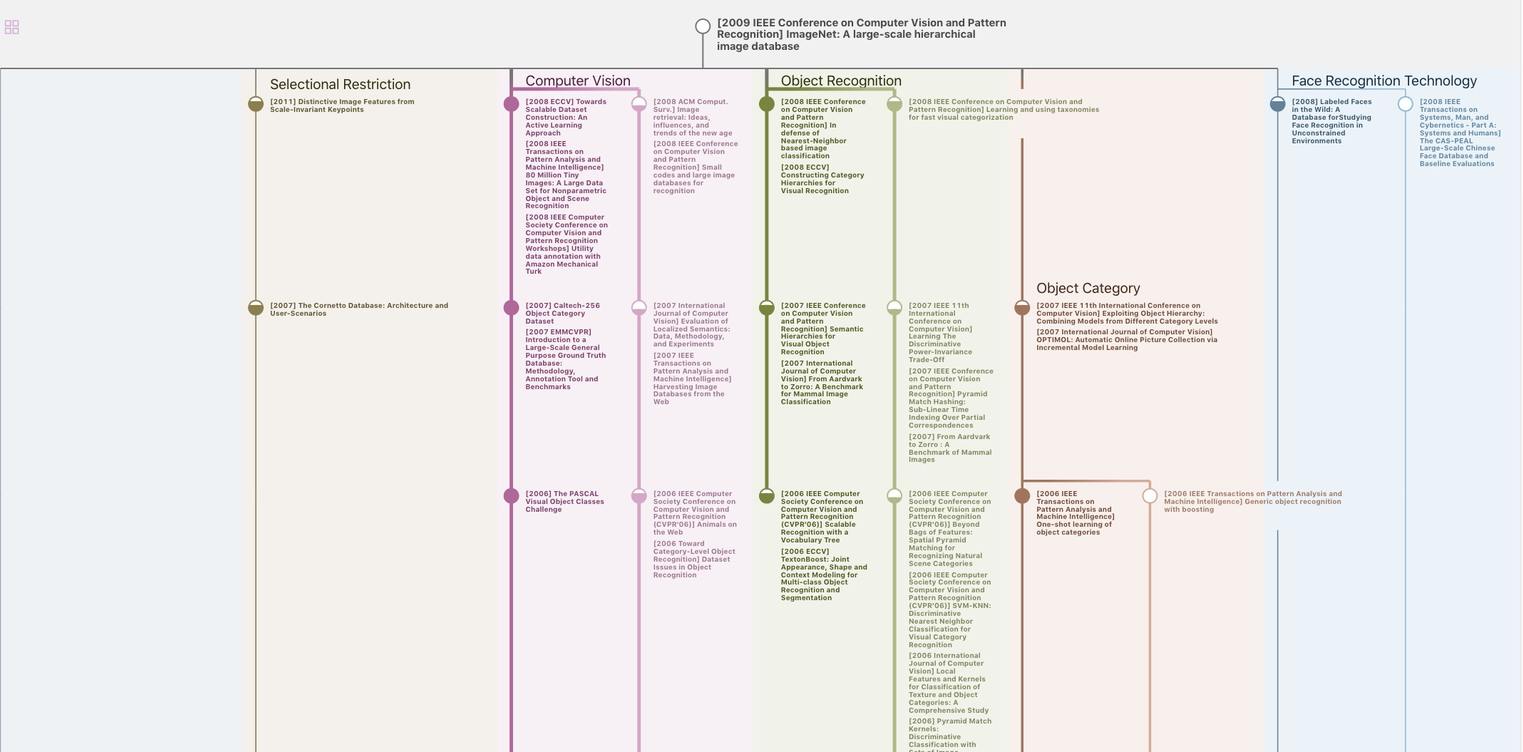
生成溯源树,研究论文发展脉络
Chat Paper
正在生成论文摘要