Tool Condition Monitoring in Drilling Using Artificial Neural Networks
AI 2003: ADVANCES IN ARTIFICIAL INTELLIGENCE(2003)
摘要
A reliable and sensitive technique for monitoring tool condition in drilling is essential help for practising engineers. It is commonly known that the unattended use of a drill bit until it reaches the ultimate failure can potentially damage to machine tool and work-piece resulting in considerable down time and productivity loss. Thus there is a need for such tools to save high maintenance costs in case of the catastrophic failure. A system in drilling that can estimate tool life in terms of the number of hole to failure as condition monitoring techniques in the form of a digital display is significantly beneficial. In this paper, a tailor-made novel feed forward network is proposed to predict tool life in terms of the number of holes to failure. These involved the development of predictive model, test rig design and a digital display to assist engineers with on-line tool life. To entitle the network to cater for various cutting conditions, a knowledge base as training and testing data have to be generated on the experimental data in a comprehensive working range of drilling. Consequently, the experiments were performed in thirty-two cutting conditions based on the combination of three basic cutting parameters, which are feed rate, spindle speed and drill diameter. The neural networks were trained and the architecture of networks was appropriately selected by benchmarking the Root Mean Square error (RMS). The results of the novel network, Optimisation layer by layer (OLL), have shown the ability to accurately predict the number of holes to failure with a 100% success rate at both training and testing stages. To highlight OLL predictive capability, a brief comparison with Backpropagation Neural Network (BPNN) is carried out.
更多查看译文
关键词
prediction model,artificial neural network,machine tool,feed forward,knowledge base,root mean square error,neural network,layer by layer
AI 理解论文
溯源树
样例
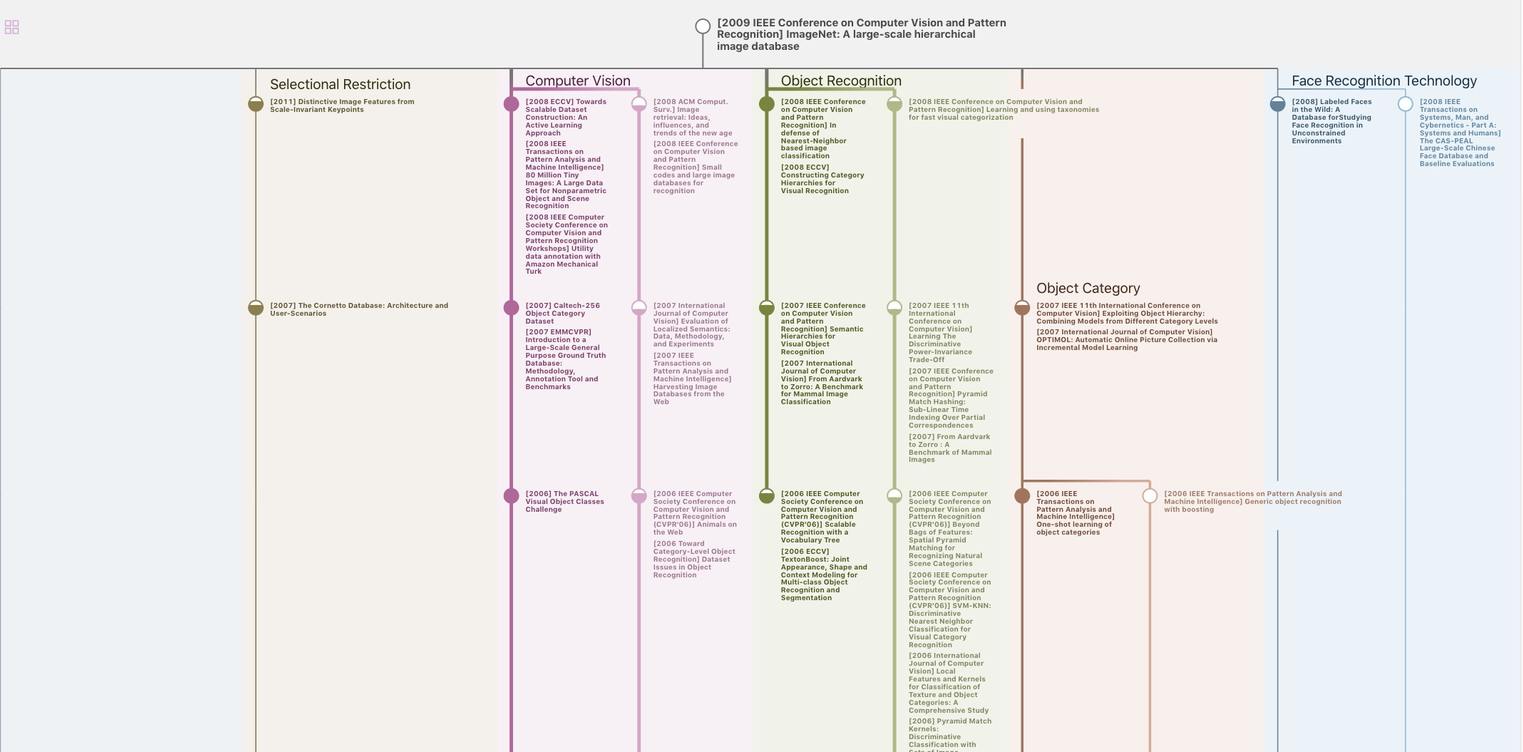
生成溯源树,研究论文发展脉络
Chat Paper
正在生成论文摘要