Learning Alpha-Integration With Partially-Labeled Data
2010 IEEE INTERNATIONAL CONFERENCE ON ACOUSTICS, SPEECH, AND SIGNAL PROCESSING(2010)
摘要
Sensory data integration is an important task in human brain for multimodal processing as well as in machine learning for multisensor processing. alpha-integration was proposed by Amari as a principled way of blending multiple positive measures (e.g., stochastic models in the form of probability distributions), providing an optimal integration in the sense of minimizing the alpha-divergence. It also encompasses existing integration methods as its special case, e. g., weighted average and exponential mixture. In alpha-integration, the value of a determines the characteristics of the integration and the weight vector w assigns the degree of importance to each measure. In most of the existing work, however, a and w are given in advance rather than learned. In this paper we present two algorithms, for learning a and w from data when only a few integrated target values are available. Numerical experiments on synthetic as well as real-world data confirm the proposed method's effectiveness.
更多查看译文
关键词
alpha-integration, parameter estimation
AI 理解论文
溯源树
样例
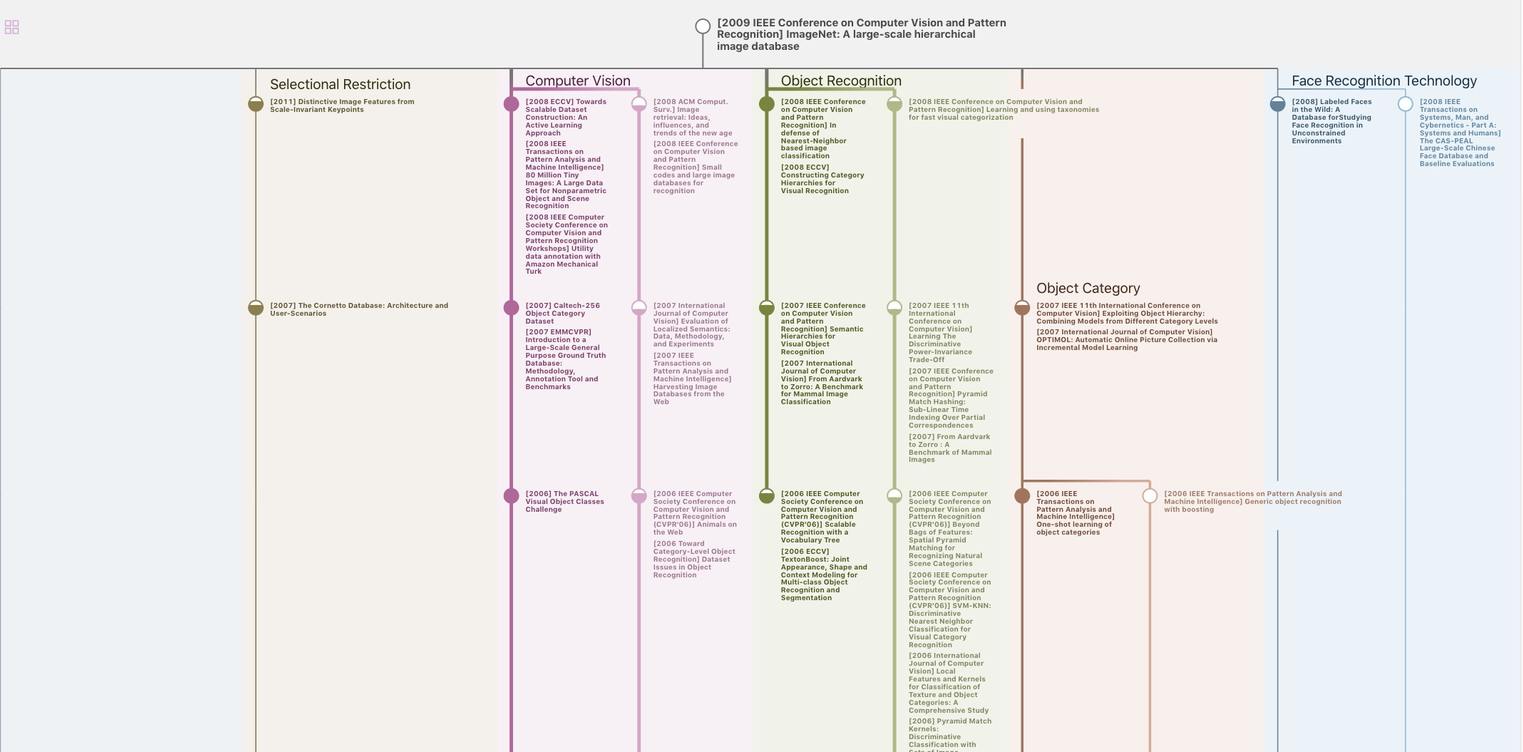
生成溯源树,研究论文发展脉络
Chat Paper
正在生成论文摘要