Exploiting interactions among polymorphisms contributing to complex disease traits with boosted generative modeling.
JOURNAL OF COMPUTATIONAL BIOLOGY(2007)
摘要
Although there has been great success in identifying disease genes for simple, monogenic Mendelian traits, deciphering the genetic mechanisms involved in complex diseases remains challenging. One major approach is to identify configurations of interacting factors such as single nucleotide polymorphisms (SNPs) that confer susceptibility to disease. Traditional methods, such as the multiple dimensional reduction method and the combinatorial partitioning method, provide good tools to decipher such interactions amid a disease population with a single genetic cause. However, these traditional methods have not managed to resolve the issue of genetic heterogeneity, which is believed to be a very common phenomenon in complex diseases. There is rarely prior knowledge of the genetic heterogeneity of a disease, and traditional methods based on estimation over the entire population are unlikely to succeed in the presence of heterogeneity. We present a novel Boosted Generative Modeling (BGM) approach for structure-model the interactions leading to diseases in the context of genetic heterogeneity. Our BGM method bridges the ensemble and generative modeling approaches to genetic association studies under a case-control design. Generative modeling is employed to model the interaction network configuration and the causal relationships, while boosting is used to address the genetic heterogeneity problem. We perform our method on simulation data of complex diseases. The results indicate that our method is capable of modeling the structure of interaction networks among disease-susceptible loci and of addressing genetic heterogeneity issues where the traditional methods, such as multiple dimensional reduction method, fail to apply. Our BGM method provides an exploratory tool that identifies the variables (e.g., disease-susceptible loci) that are likely to correlate and contribute to the disease.
更多查看译文
关键词
genotype,complex traits,genetic heterogeneity,boosting,generative model
AI 理解论文
溯源树
样例
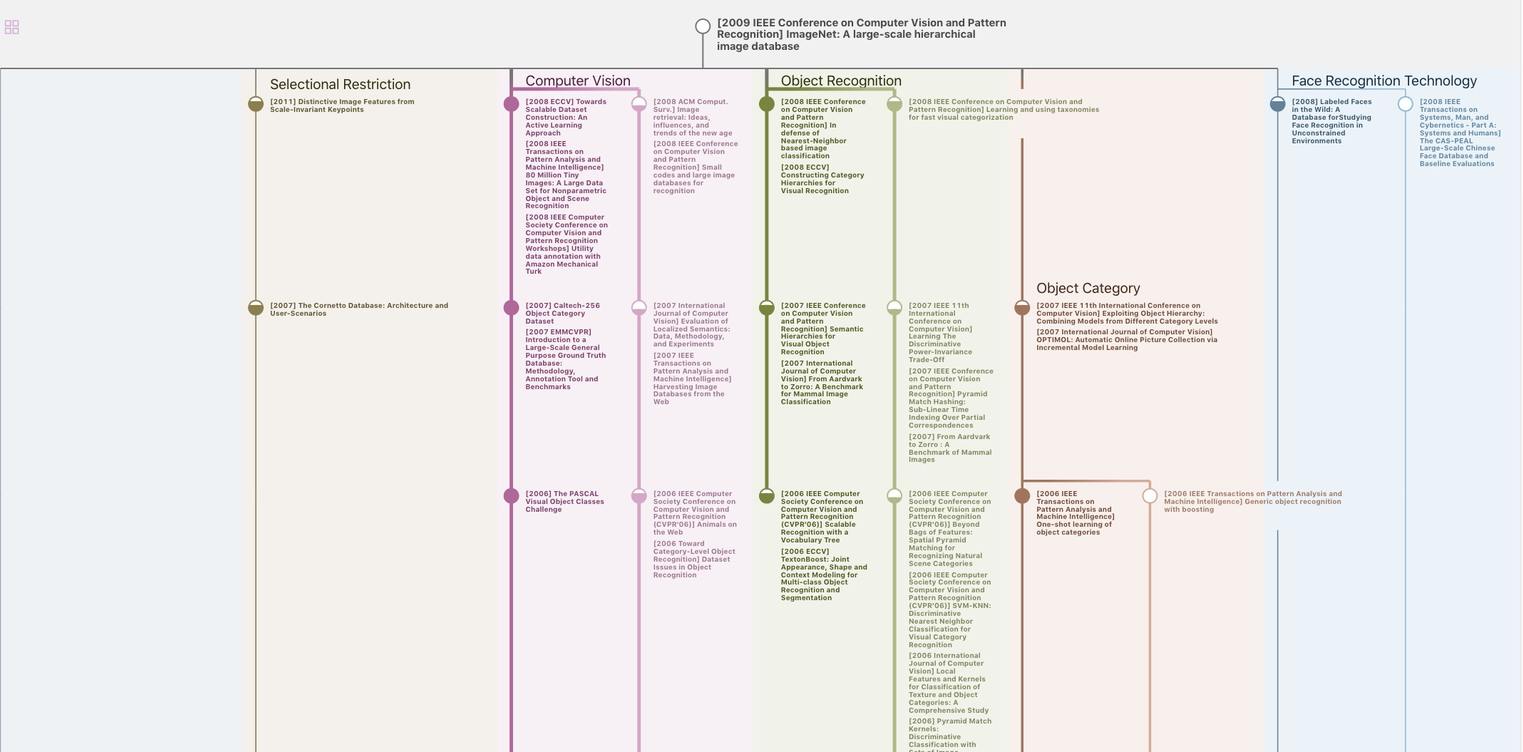
生成溯源树,研究论文发展脉络
Chat Paper
正在生成论文摘要