EC-GCN: A encrypted traffic classification framework based on multi-scale graph convolution networks.
Comput. Networks(2023)
摘要
The sharp increase in encrypted traffic brings a huge challenge to traditional traffic classification methods. Combining deep learning with time series analysis techniques is a recent trend in solving this problem. Most of these approaches only capture the temporal correlation within a flow. The accuracy and robustness are unsatisfactory, especially in an unstable network environment with high packet loss and reordering. How to learn a representation with a strong generalization ability for each encrypted traffic flow remains a key challenge. Our detailed analysis indicates that there is a graph with particular local structures corresponding to each type of encrypted traffic flow. Inspired by this observation, we propose a novel deep learning framework called EC-GCN to classify encrypted traffic flows based on multi-scale graph convolutional neural networks. We first provide a novel lightweight layer that only relies on the metadata and encodes each encrypted traffic flow into graph representations. So that our framework can be independent of different encryption protocols. Then we design a novel graph pooling and structure learning layer to dynamically extract the multi-graph representations and improve the capabilities to adapt to complex network environments. EC-GCN is an end-to-end classification model that learns representative spatial-temporal traffic features hidden in a traffic time series and then classifies them in a unified framework. Our comprehensive experiments on three real-world datasets indicate that EC-GCN can achieve up to 5%-20% accuracy improvement and outperforms state-of-the-art methods.
更多查看译文
关键词
Encrypted traffic classification,Graph neural networks,Packet sequence learning
AI 理解论文
溯源树
样例
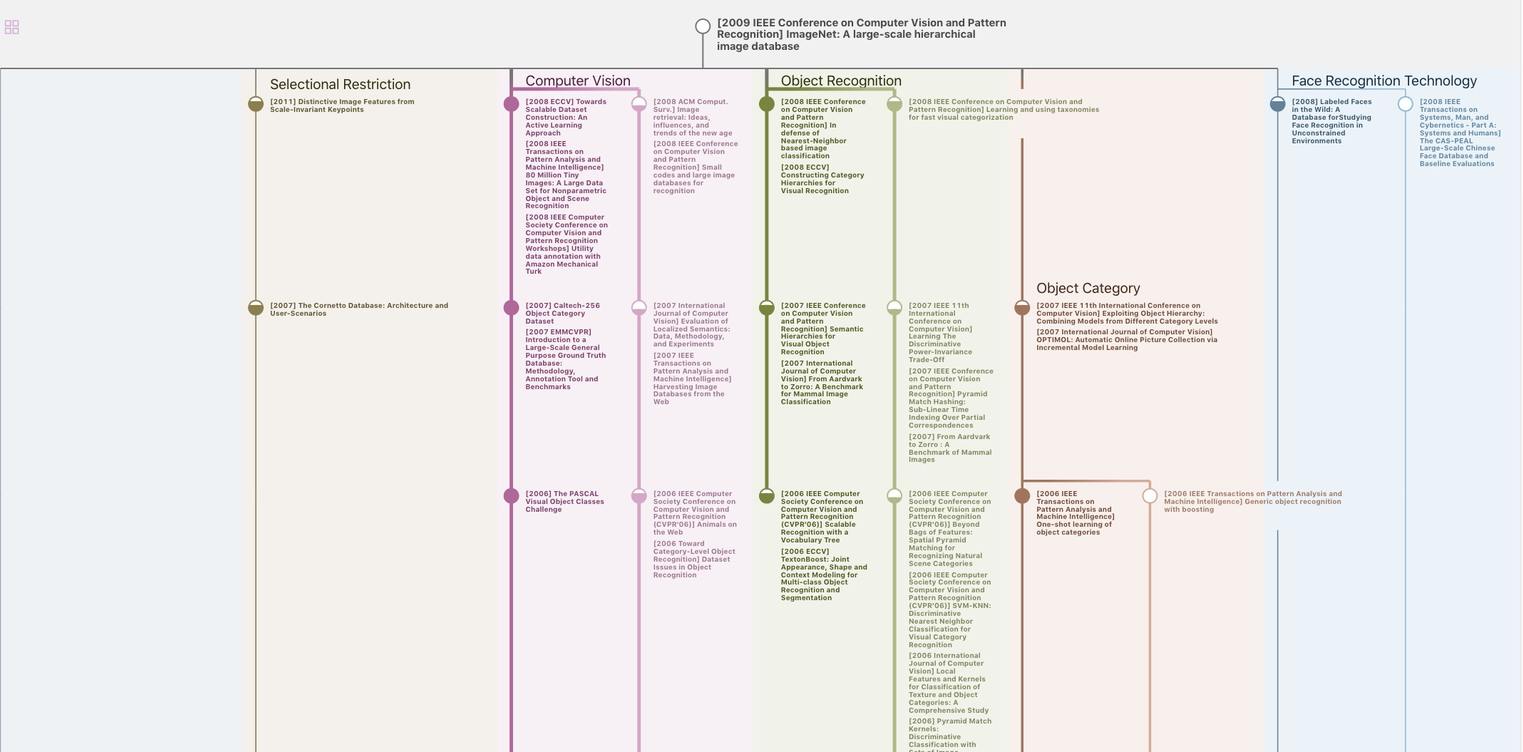
生成溯源树,研究论文发展脉络
Chat Paper
正在生成论文摘要