Improving Search Relevance For Short Queries In Community Question Answering
WSDM(2014)
摘要
Relevant question retrieval and ranking is a typical task in community question answering (CQA). Existing methods mainly focus on long and syntactically structured queries. However, when an input query is short, the task becomes challenging, due to a lack information regarding user intent. In this paper, we mine different types of user intent from various sources for short queries. With these intent signals, we propose a new intent-based language model. The model takes advantage of both state-of-the-art relevance models and the extra intent information mined from multiple sources. We further employ a state-of-the-art learning-to-rank approach to estimate parameters in the model from training data. Experiments show that by leveraging user intent prediction, our model significantly outperforms the state-of-the-art relevance models in question search.
更多查看译文
关键词
community question answering,question search,short query,user intent
AI 理解论文
溯源树
样例
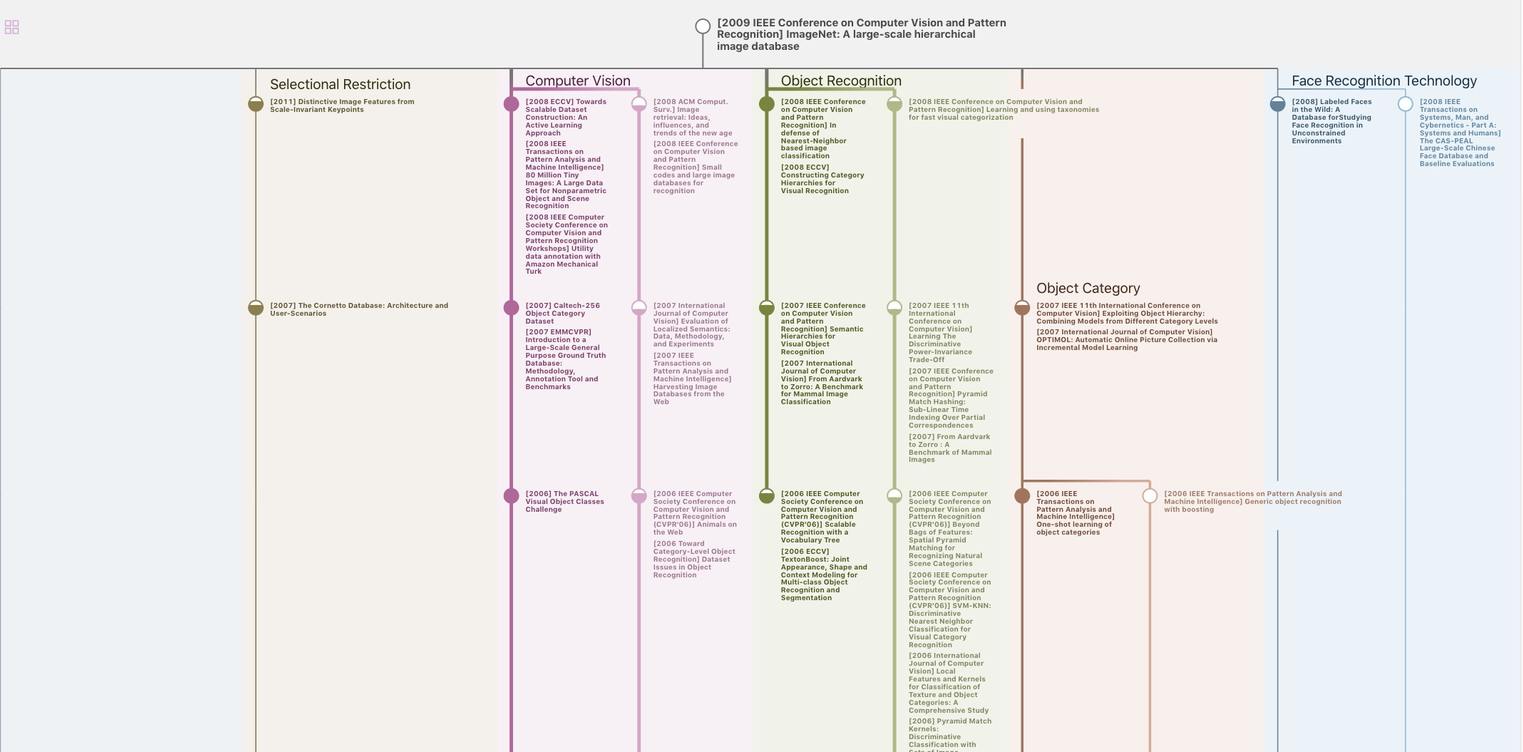
生成溯源树,研究论文发展脉络
Chat Paper
正在生成论文摘要