Classification of discrete emotions in speech using prosodic and spectral features: intra and cross-lingual studies in five native languages of assam
Classification of discrete emotions in speech using prosodic and spectral features: intra and cross-lingual studies in five native languages of assam(2012)
摘要
The thesis proposes new sets of features for discrete vocal emotion recognition in five native languages of Assam, a north-eastern state of India. The proposed feature sets have been extensively compared with some of the existing features available in the literature. The overall objective of the present work is to investigate whether vocal expressions of discrete emotion can be distinguished (i) from no-emotion (i.e. neutral), (ii) from another, and (iii) from surprise which is a cognitive component could be present with any emotion. All these studies have been carried out in intra-lingual and cross-lingual cases. This study will enable us to get more information regarding nature and function of emotion. Furthermore, this work will help in developing a generalized vocal discrete emotion recognition system, which will increase the efficiency of human-machine interaction systems. A vocal portrayed emotion database of six full-blown discrete emotions (Anger, Disgust, Fear, Happiness, Sadness, and Surprise) and ‘No-emotion’ (i.e. Neutral) has been created with 140 utterances per speaker (20 per emotion) consisting of short sentences of five native languages of Assam. The total number of speakers in each language is 6 (3 Males and 3 Females). This database is validated by a Listening Test (i.e. Subjective test). Eight different types of feature sets are extracted from the utterances. These are based on Prosodic features, Mel Frequency Cepstral Coefficients (MFCC); Log Frequency Power Coefficients (LFPC), Wavelet Packet Cepstral Coefficients (WPCC), Linear Prediction Cepstral Coefficients (LPCC), Line Spectral Frequencies (LSF), and Eigen Values of Autocorrelation Matrix (EVAM). The Gaussian Mixture Model (GMM) is used as the classifier. The comparative performances of all these feature sets are evaluated with respect to the accuracy of classification in two cases: (i) text-and-speaker independent vocal emotion recognition in each language, and (ii) cross-lingual vocal emotion recognition. Two feature sets are proposed in this thesis based on (1) WPCC2 and (2) EVAM. Key words: Vocal Emotion Recognition; Gaussian Mixture Model (GMM) Classifier; Prosodic Features; Mel Frequency Cepstral Coefficients (MFCC); Log Frequency Power Coefficients (LFPC); Wavelet Packet Cepstral Coefficients (WPCC); Prediction Cepstral Coefficients (LPCC); Line Spectral Frequencies (LSF); Eigen Values of Autocorrelation Matrix (EVAM); Multilingual Emotional Speech Database of North-East India (MESDNEI)
更多查看译文
关键词
native language,discrete vocal emotion recognition,Wavelet Packet Cepstral Coefficients,feature set,discrete emotion,spectral feature,cross-lingual study,full-blown discrete emotion,cross-lingual vocal emotion recognition,generalized vocal discrete emotion,Mel Frequency Cepstral Coefficients,Log Frequency Power Coefficients,emotion database
AI 理解论文
溯源树
样例
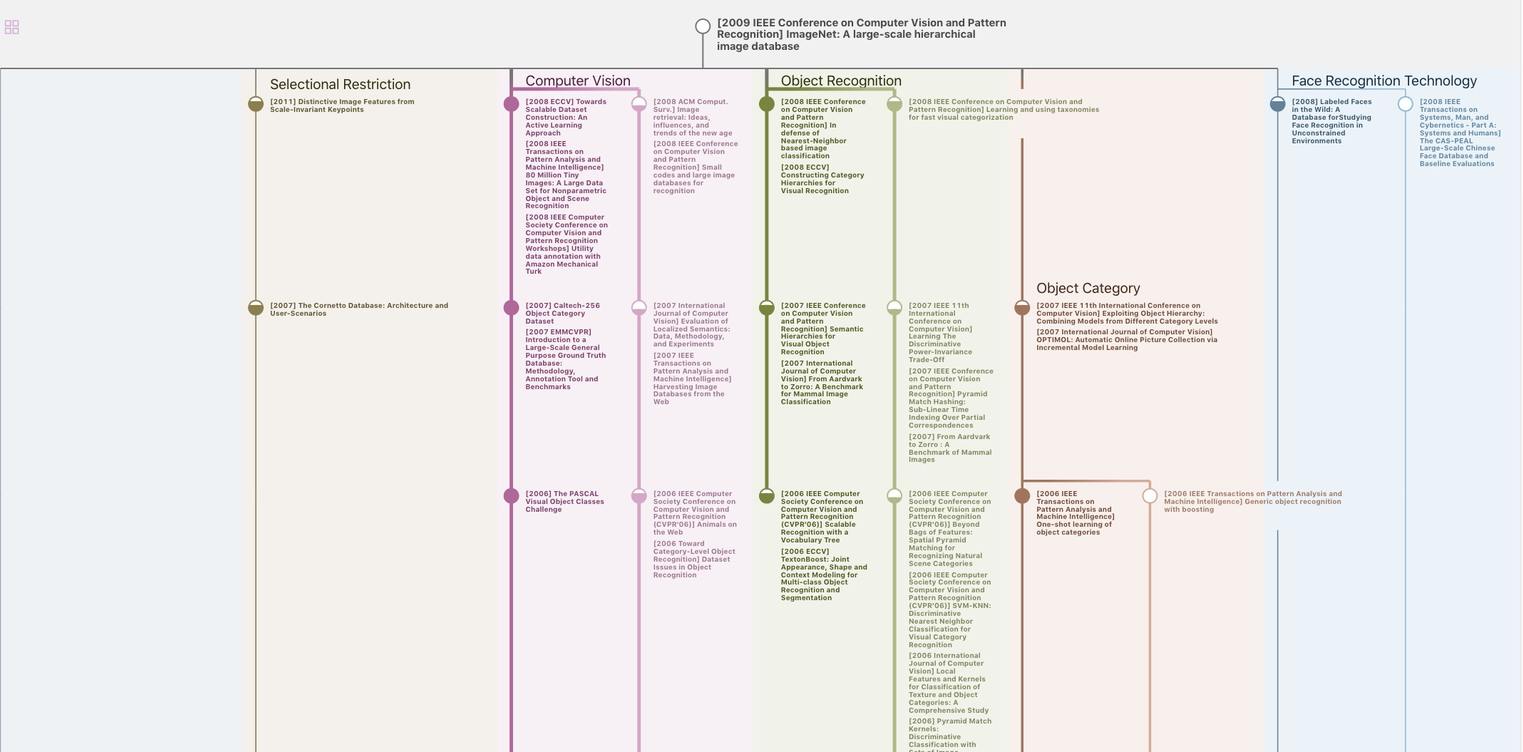
生成溯源树,研究论文发展脉络
Chat Paper
正在生成论文摘要