Machine Learning and the Spatial Structure of House Prices and Housing Returns
Social Science Research Network(2008)
摘要
Economists do not have reliable measures of current house values, let alone housing re- turns. This ignorance underlies the illiquidity of mortgage-backed securities, which in turn feeds back to deepen the sub-prime crisis. Using a massive new data tape of housing transactions in L.A., we demonstrate systematic patterns in the error associated with using the ubiquitous repeat sales methodology to understand house values. In all periods, the resulting indices under-predict sales prices of less expensive homes, and over-predict prices of more expen- sive homes. The recent period has produced errors that are not only unprecedentedly large in absolute value, but highly systematic: after a few years in which the indices under-predicted prices, they now significantly over-predict them. We introduce new machine learning tech- niques from computer science to correct for prediction errors that have geographic origins. The results are striking. Accounting for geography significantly reduces the extent of the prediction error, removes many of the systematic patterns, and results in far less deterioration in model performance in the recent period.
更多查看译文
关键词
and ed wike for their help. we thank new york university for its,sub-prime crisis we thank leslie greengard,default,damien weldon,machine learning,house prices
AI 理解论文
溯源树
样例
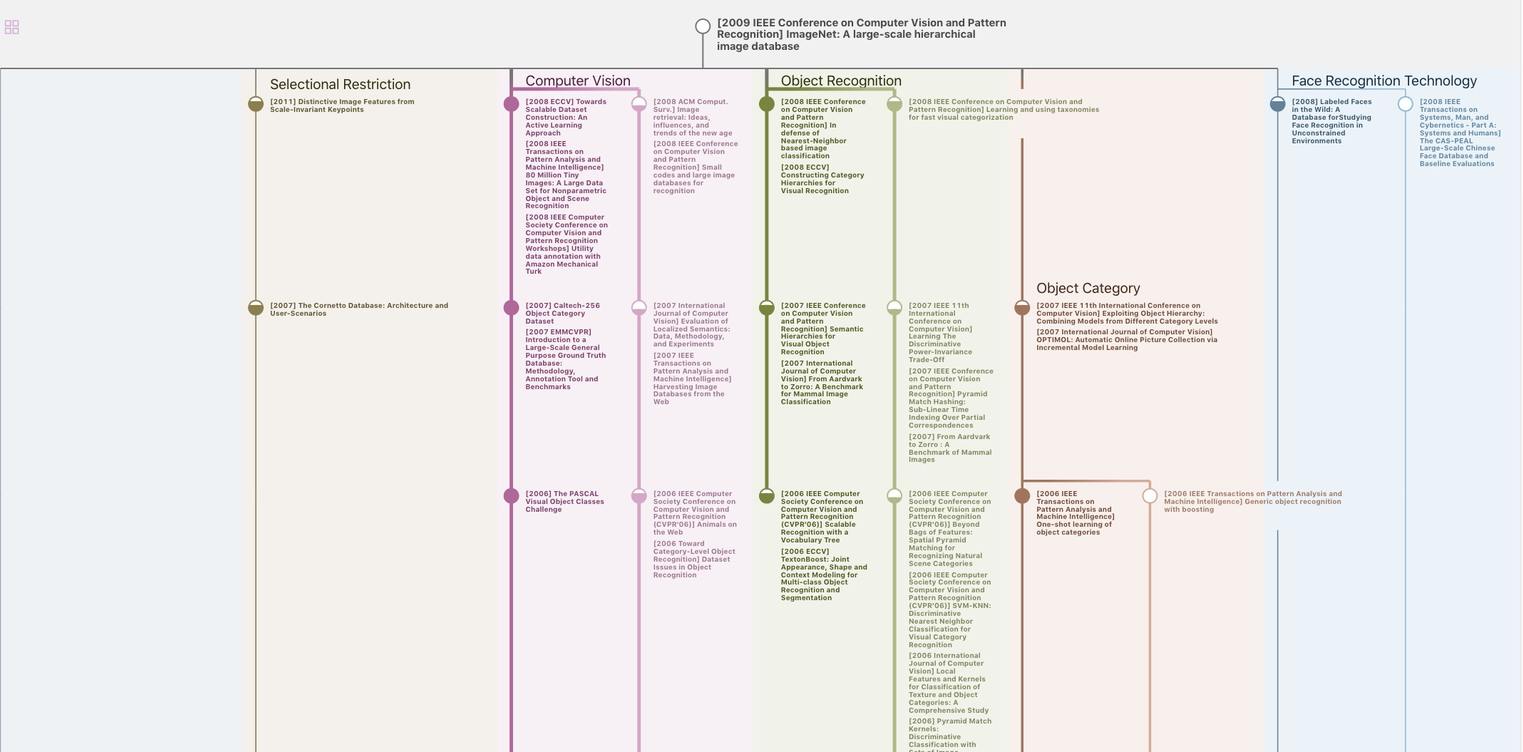
生成溯源树,研究论文发展脉络
Chat Paper
正在生成论文摘要