Online learning of large margin hidden markov models for automatic speech recognition
MLSLP(2011)
摘要
Over the last two decades, large margin methods have yielded excellent performance on many tasks. The theoretical properties of large margin methods have been intensively studied and are especially well-established for support vector machines (SVMs). However, the scalability of large margin methods remains an issue due to the amount of computation they require. This is especially true for applications involving sequential data.In this thesis we are motivated by the problem of automatic speech recognition (ASR) whose large-scale applications involve training and testing on extremely large data sets. The acoustic models used in ASR are based on continuous-density hidden Markov models (CD-HMMs). Researchers in ASR have focused on discriminative training of HMMs, which leads to models with significantly lower error rates. More recently, building on the successes of SVMs and various extensions thereof in the machine learning community, a number of researchers in ASR have also explored large margin methods for discriminative training of HMMs.This dissertation aims to apply various large margin methods developed in the machine learning community to the challenging large-scale problems that arise in ASR. Specifically, we explore the use of sequential, mistake-driven updates for online learning and acoustic feature adaptation in large margin HMMs. The updates are applied to the parameters of acoustic models after the decoding of individual training utterances. For large margin training, the updates attempt to separate the log-likelihoods of correct and incorrect transcriptions by an amount proportional to their Hamming distance. For acoustic feature adaptation, the updates attempt to improve recognition by linearly transforming the features computed by the front end. We evaluate acoustic models trained in this way on the TIMIT speech database. We find that online updates for large margin training not only converge faster than analogous batch optimizations, but also yield lower phone error rates than approaches that do not attempt to enforce a large margin.We conclude this thesis with a discussion of future research directions, highlighting in particular the challenges of scaling our approach to the most difficult problems in large-vocabulary continuous speech recognition.
更多查看译文
关键词
large margin training,automatic speech recognition,large margin HMMs,large margin method,large data set,various large margin method,individual training utterance,acoustic feature adaptation,markov model,large margin,discriminative training,acoustic model
AI 理解论文
溯源树
样例
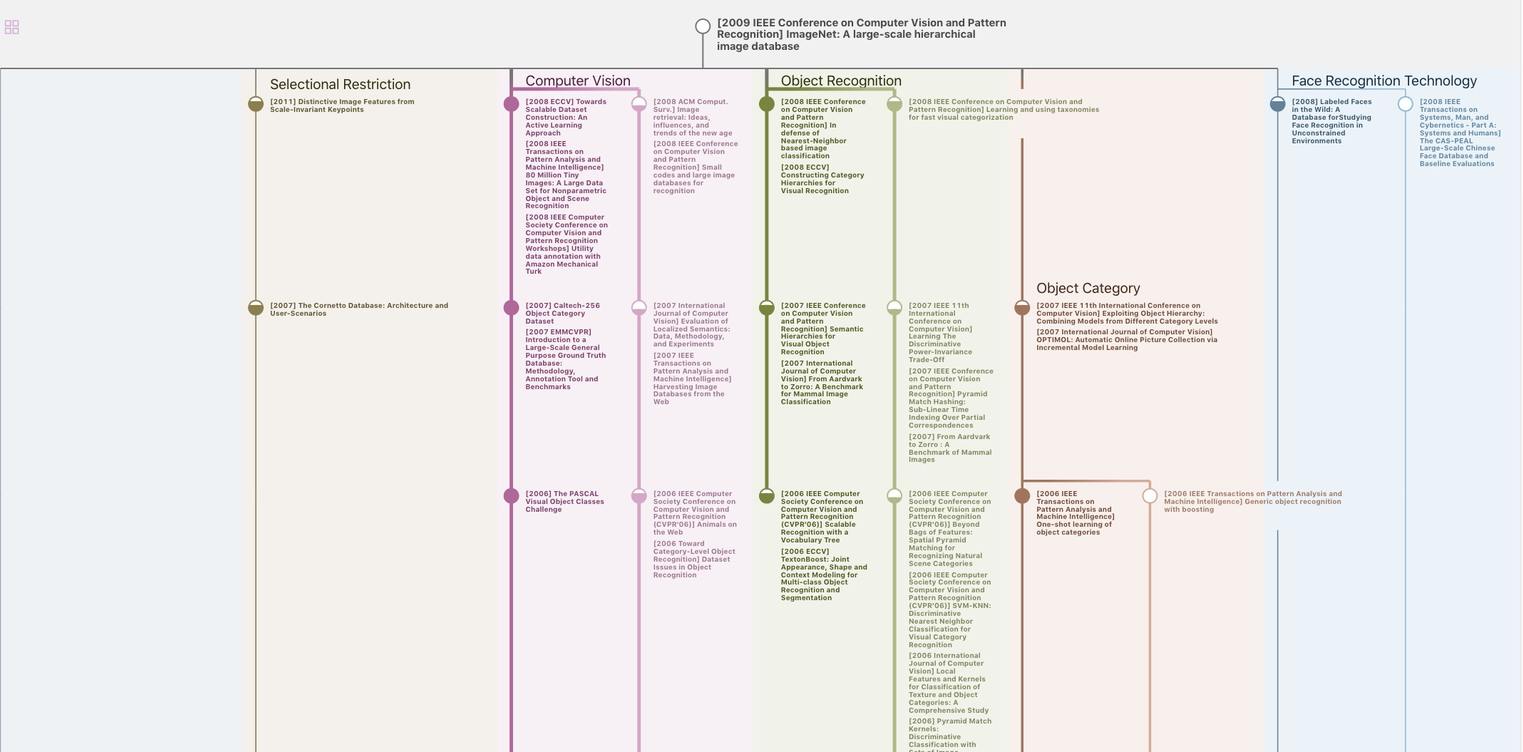
生成溯源树,研究论文发展脉络
Chat Paper
正在生成论文摘要