Biglog: Unsupervised Large-scale Pre-training for a Unified Log Representation.
IWQoS(2023)
摘要
Automated log analysis has been widely applied in modern data-center network, performing critical tasks such as log parsing, log anomaly detection and log-based failure prediction. However, existing approaches rely on hand-crafted features or domain-specific vectors to represent logs, which are either laborious in manual efforts or ineffective facing multiple domains in a system. Furthermore, general-purpose word embeddings are not optimized for log data, thus are data-inefficient in handling complex log analysis tasks. In this paper, we present a pre-training phase for language models to understand both in-sentence and cross-sentence features of logs, resulting in a unified representation of logs that is well-suited for various downstream analysis tasks. The pre-training phase is unsupervised, utilizing 0.45 billion logs from 16 diverse domains. Experiments on 12 publicly available evaluation datasets across 3 tasks indicate superiority of our approach against existing approaches, especially in online scenarios with limited historical logs. Our approach also exhibits remarkable few-shot learning ability and domain-adaptiveness, which not only outperforms existing approaches using only 0.0025% of their required training data, but also adapts into new domains via only a few in-domain logs. We release our code and pre-trained model.
更多查看译文
关键词
log analysis,language model,log pre-training,domain adaption
AI 理解论文
溯源树
样例
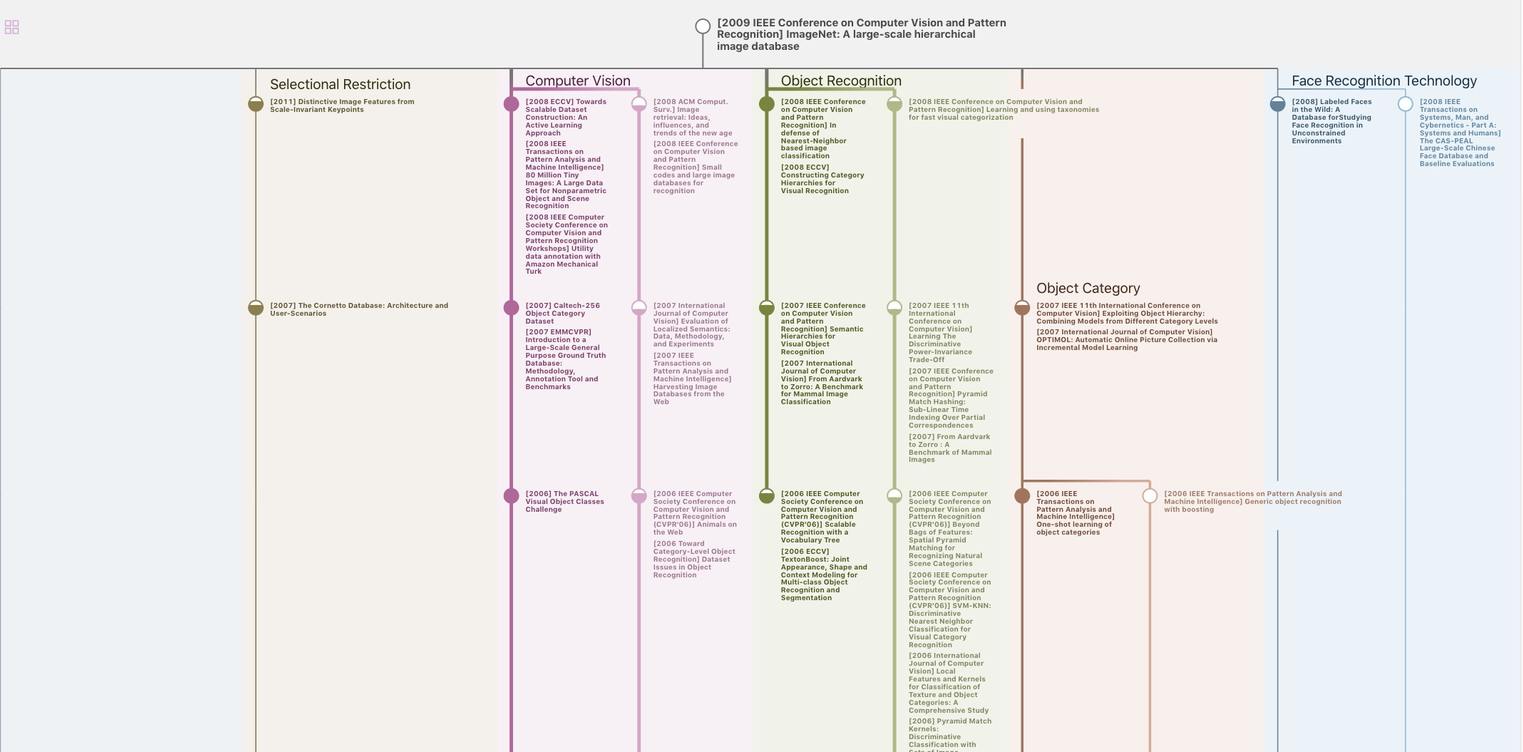
生成溯源树,研究论文发展脉络
Chat Paper
正在生成论文摘要