Evolutionary based feature extraction with dynamic mutation
IEEE Congress on Evolutionary Computation(2011)
摘要
Determining a good feature set is critical to the performance of learning algorithms such as classifiers. Recently, researchers have proposed evolutionary-based feature extraction methods that aim to find a good feature set by combining the original features with new features generated by mathematical transformations of the original features. In this paper, we propose dynamically collecting past performance information on promising features and operators to use in our mutation method. We consider how to make our evolutionary algorithm more efficient and reliable by reducing overfitting. Preliminary results using UCI data show that our dynamic mutation method only slightly enhances the classification accuracy but it produces more reliable results.
更多查看译文
关键词
overfitting,evolutionary algorithm,evolutionary computation,mathematical transformation,pattern classification,dynamic mutation method,evolutionary based feature extraction,feature extraction,classifiers,uci data,classification accuracy,classification,dynamic mutation,learning algorithm,accuracy,testing,history,classification algorithms,evolutionary computing
AI 理解论文
溯源树
样例
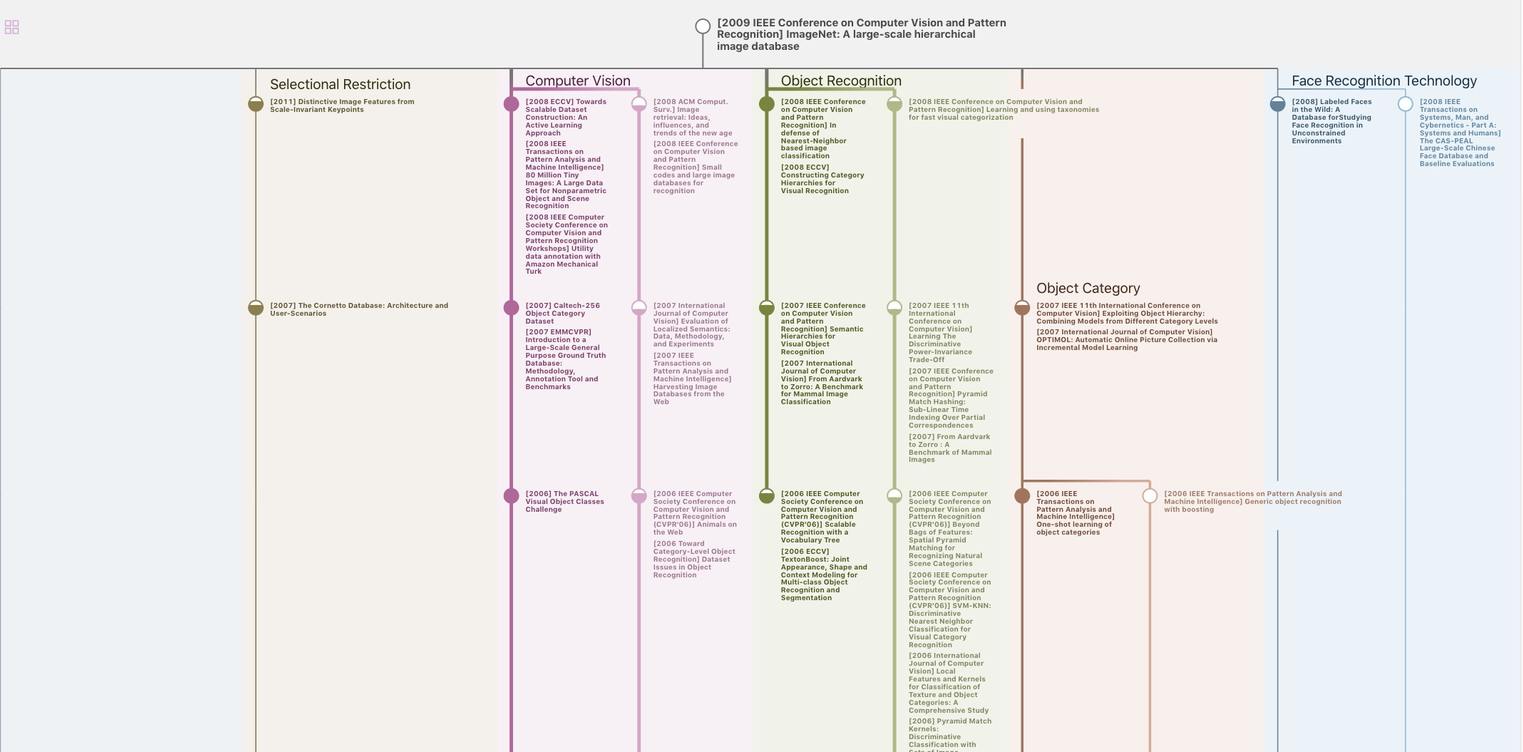
生成溯源树,研究论文发展脉络
Chat Paper
正在生成论文摘要