Learning Bayesian Network Models from Incomplete Data using Importance Sampling.
AISTATS(2005)
摘要
We propose a Bayesian approach to learning Bayesian network models from incomplete data. The objective is to obtain the posterior distribution of models, given the observed part of the data. We describe a new algo- rithm, called eMC4, to simulate draws from this posterior distribution. One of the new ideas in our algorithm is to use importance sampling to approximate the posterior distri- bution of models given the observed data and the current imputation model. The impor- tance sampler is constructed by dening an approximate predictive distribution for the unobserved part of the data. In this way existing (heuristic) imputation methods can be used that don't require exact inference for generating imputations. We illustrate eMC4 by its application to mod- eling the risk factors of coronary heart dis- ease. In the experiments we consider dieren t missing data mechanisms and dieren t frac- tions of missing data.
更多查看译文
AI 理解论文
溯源树
样例
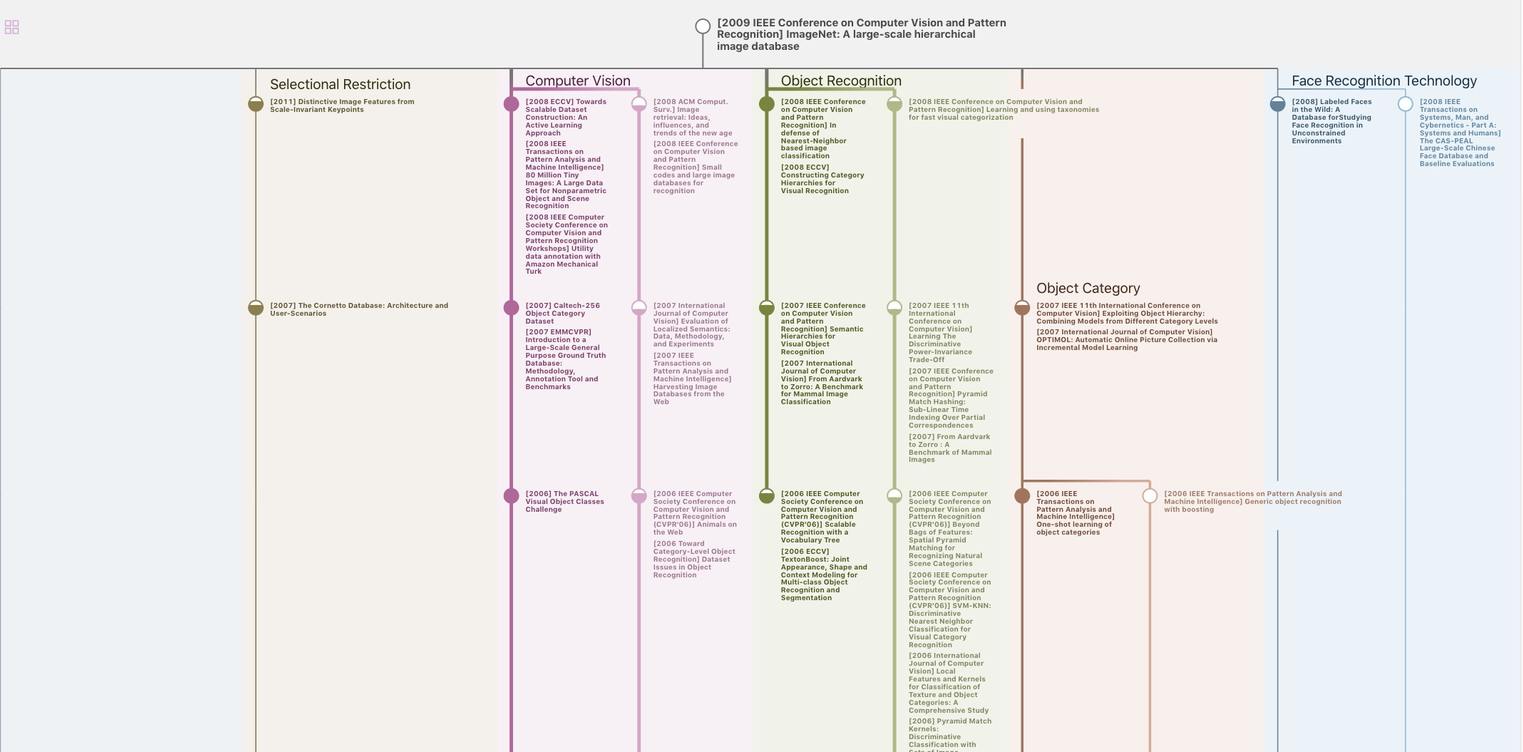
生成溯源树,研究论文发展脉络
Chat Paper
正在生成论文摘要