K-Means-Based Consensus Clustering: A Unified View
IEEE Trans. Knowl. Data Eng.(2015)
摘要
The objective of consensus clustering is to find a single partitioning which agrees as much as possible with existing basic partitionings. Consensus clustering emerges as a promising solution to find cluster structures from heterogeneous data. As an efficient approach for consensus clustering, the K-means based method has garnered attention in the literature, however the existing research efforts are still preliminary and fragmented. To that end, in this paper, we provide a systematic study of K-means-based consensus clustering (KCC). Specifically, we first reveal a necessary and sufficient condition for utility functions which work for KCC. This helps to establish a unified framework for KCC on both complete and incomplete data sets. Also, we investigate some important factors, such as the quality and diversity of basic partitionings, which may affect the performances of KCC. Experimental results on various realworld data sets demonstrate that KCC is highly efficient and is comparable to the state-of-the-art methods in terms of clustering quality. In addition, KCC shows high robustness to incomplete basic partitionings with many missing values.
更多查看译文
关键词
heterogeneous data,k-means,pattern clustering,consensus clustering,utility function,partitioning diversity,incomplete data sets,kcc,utility functions,k-means-based consensus clustering,cluster structures,complete data sets,partitioning quality,vectors,linear programming,convex functions,robustness,clustering algorithms,k means
AI 理解论文
溯源树
样例
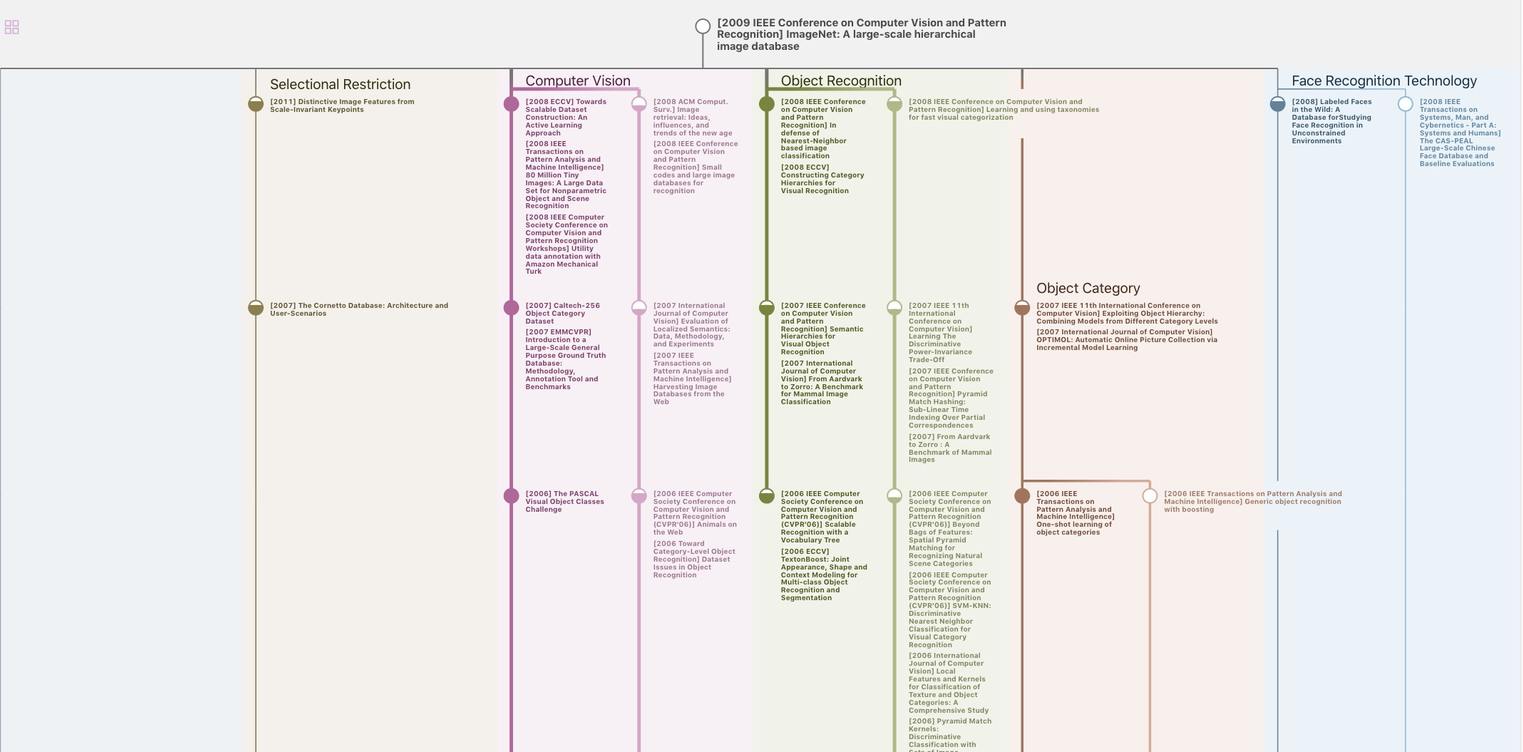
生成溯源树,研究论文发展脉络
Chat Paper
正在生成论文摘要