Feature Selection Via L(1)-Penalized Squared-Loss Mutual Information
IEICE TRANSACTIONS ON INFORMATION AND SYSTEMS(2013)
摘要
Feature selection is a technique to screen out less important features. Many existing supervised feature selection algorithms use redundancy and relevancy as the main criteria to select features. However, feature interaction, potentially a key characteristic in real-world problems, has not received much attention. As an attempt to take feature interaction into account, we propose l(1)-LSMI, an l(1)-regularization based algorithm that maximizes a squared-loss variant of mutual information between selected features and outputs. Numerical results show that l(1)-LSMI performs well in handling redundancy, detecting non-linear dependency, and considering feature interaction.
更多查看译文
关键词
feature selection, l(1)-regularization, squared-loss mutual information, density-ratio estimation, dimensionality reduction
AI 理解论文
溯源树
样例
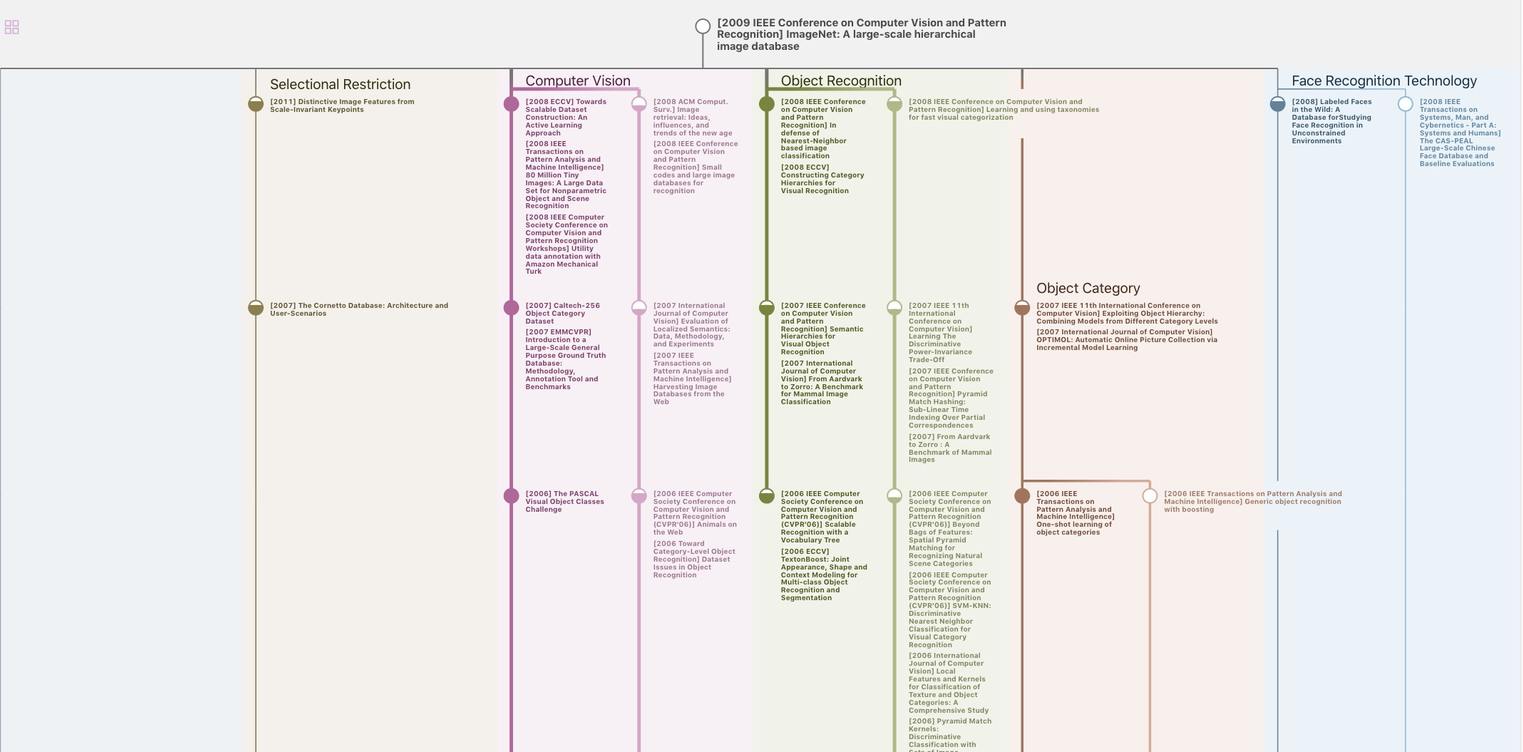
生成溯源树,研究论文发展脉络
Chat Paper
正在生成论文摘要