Phase Space Reconstruction from Accelerator Beam Measurements Using Neural Networks and Differentiable Simulations
arxiv(2023)
摘要
Characterizing the phase space distribution of particle beams in accelerators is a central part of accelerator understanding and performance optimization. However, conventional reconstruction-based techniques either use simplifying assumptions or require specialized diagnostics to infer high-dimensional ($>$ 2D) beam properties. In this Letter, we introduce a general-purpose algorithm that combines neural networks with differentiable particle tracking to efficiently reconstruct high-dimensional phase space distributions without using specialized beam diagnostics or beam manipulations. We demonstrate that our algorithm accurately reconstructs detailed 4D phase space distributions with corresponding confidence intervals in both simulation and experiment using a single focusing quadrupole and diagnostic screen. This technique allows for the measurement of multiple correlated phase spaces simultaneously, which will enable simplified 6D phase space distribution reconstructions in the future.
更多查看译文
关键词
accelerator beam measurements,phase space reconstruction,neural networks
AI 理解论文
溯源树
样例
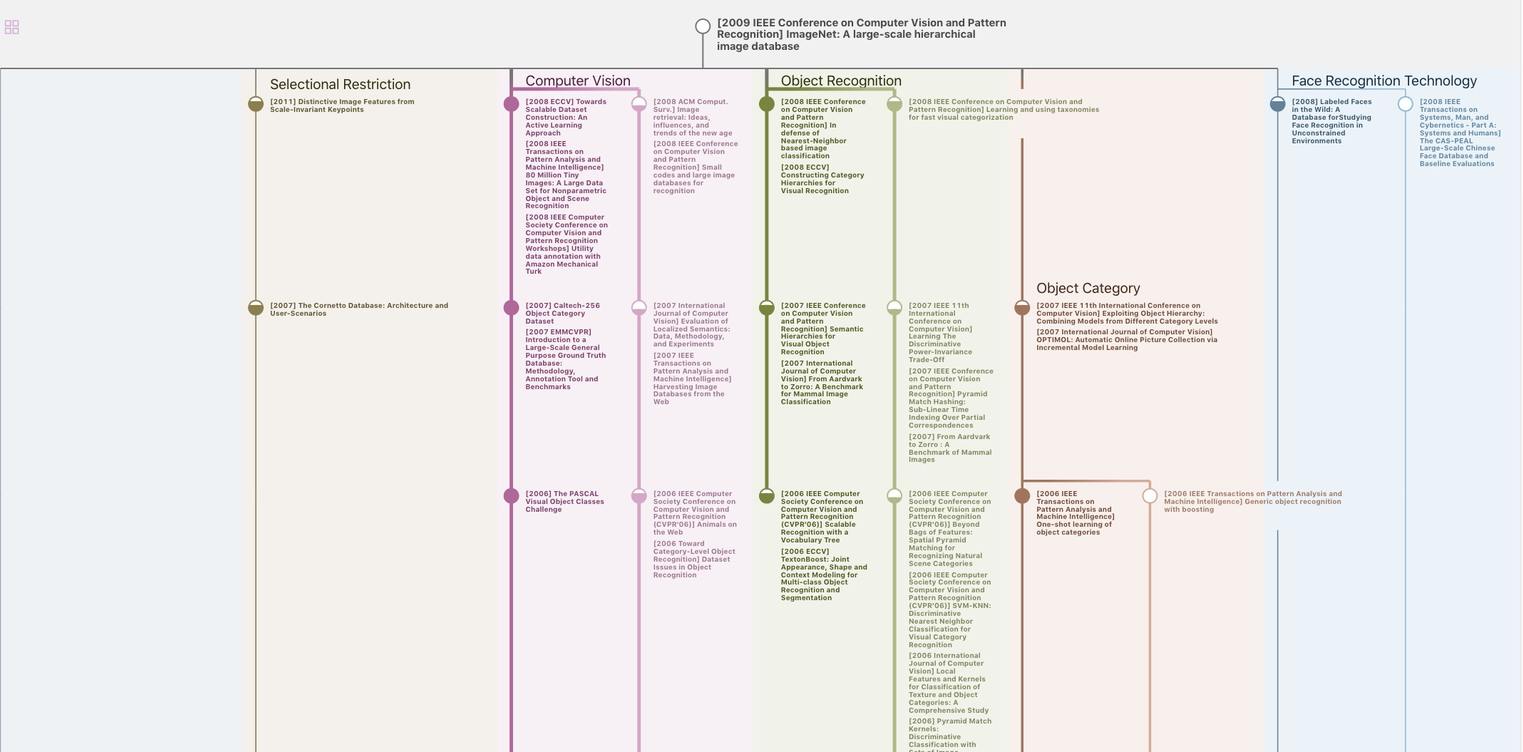
生成溯源树,研究论文发展脉络
Chat Paper
正在生成论文摘要