Discovering personally meaningful places: An interactive clustering approach
ACM Trans. Inf. Syst.(2007)
摘要
The discovery of a person's meaningful places involves obtaining the physical locations and their labels for a person's places that matter to his daily life and routines. This problem is driven by the requirements from emerging location-aware applications, which allow a user to pose queries and obtain information in reference to places, for example, “home”, “work” or “Northwest Health Club”. It is a challenge to map from physical locations to personally meaningful places due to a lack of understanding of what constitutes the real users' personally meaningful places. Previous work has explored algorithms to discover personal places from location data. However, we know of no systematic empirical evaluations of these algorithms, leaving designers of location-aware applications in the dark about their choices. Our work remedies this situation. We extended a clustering algorithm to discover places. We also defined a set of essential evaluation metrics and an interactive evaluation framework. We then conducted a large-scale experiment that collected real users' location data and personally meaningful places, and illustrated the utility of our evaluation framework. Our results establish a baseline that future work can measure itself against. They also demonstrate that that our algorithm discovers places with reasonable accuracy and outperforms the well-known K-Means clustering algorithm for place discovery. Finally, we provide evidence that shapes more complex than “points” are required to represent the full range of people's everyday places.
更多查看译文
关键词
place discovery,real user,location-aware applications,essential evaluation metrics,location data,ubiquitous computing,clustering algorithms,physical location,previous work,interactive clustering approach,meaningful place,location-aware application,future work,work remedy,clustering algorithm,field studies,k means clustering,field study
AI 理解论文
溯源树
样例
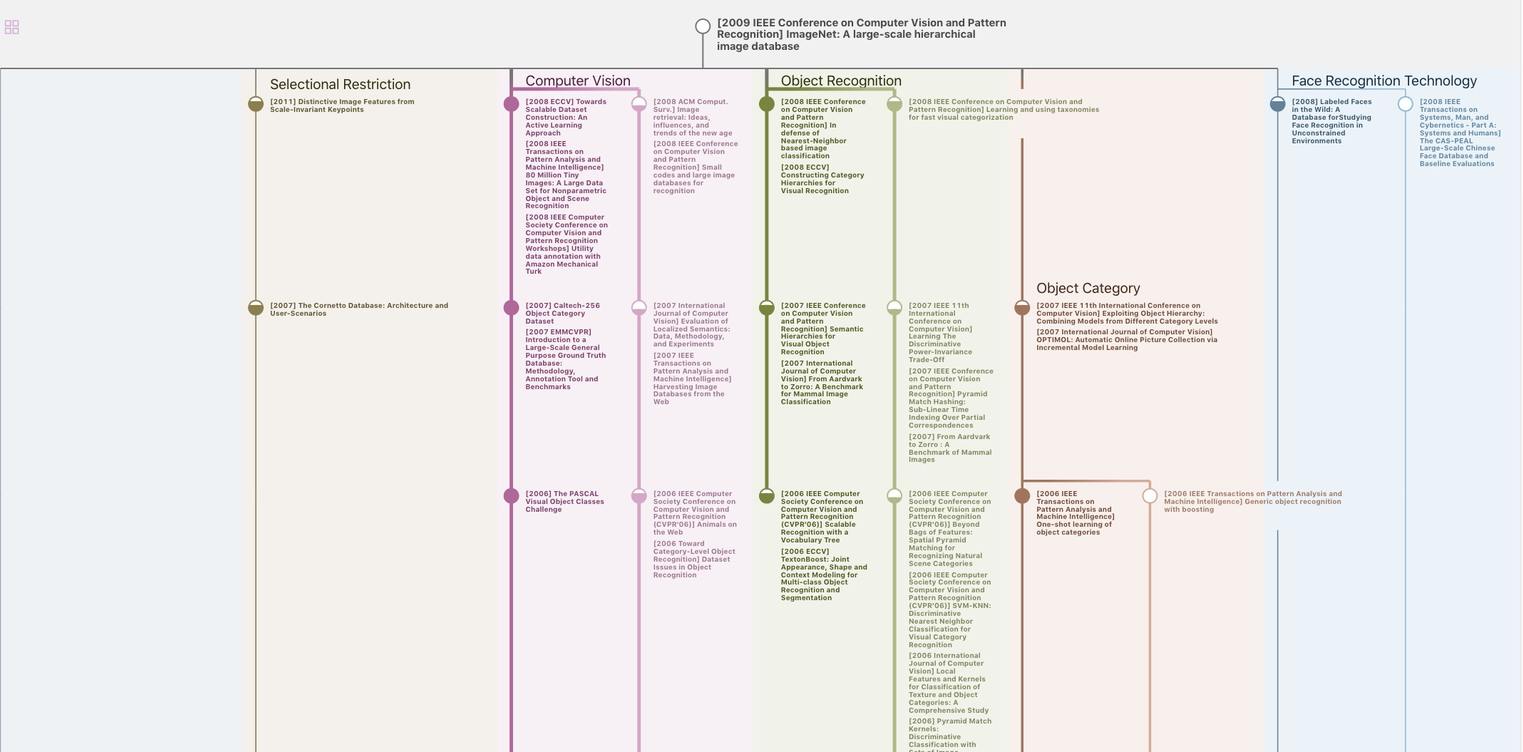
生成溯源树,研究论文发展脉络
Chat Paper
正在生成论文摘要