3D segmentation in CT imagery with conditional random fields and histograms of oriented gradients
MLMI(2011)
摘要
In this paper we focus on the problem of 3D segmention in volumetric computed tomography imagery to identify organs in the abdomen. We propose and evaluate different models and modeling strategies for 3D segmentation based on traditionalMarkov Random Fields (MRFs) and their discriminative counterparts known as Conditional Random Fields (CRFs). We also evaluate the utility of features based on histograms of oriented gradients or HOG features. CRFs and HOG features have independently produced state of the art performance in many other problem domains and we believe our work is the first to combine them and use them for medical image segmentation. We construct 3D lattice MRFs and CRFs, use variational message passing (VMP) for learning and max-product (MP) inference for prediction in the models. These inference and learning approaches allow us to learn pairwise terms in random fields that are non-submodular and are thus very flexible. We focus our experiments on abdominal organ and region segmentation, but our general approach should be useful in other settings. We evaluate our approach on a larger set of anatomical structures found within a publicly available liver database and we provide these labels for the dataset to the community for future research.
更多查看译文
关键词
hog feature,oriented gradient,anatomical structure,abdominal organ,general approach,conditional random fields,region segmentation,problem domain,conditional random field,medical image segmentation,traditionalmarkov random fields,ct imagery,lattice mrfs,mrf,generative,discriminative
AI 理解论文
溯源树
样例
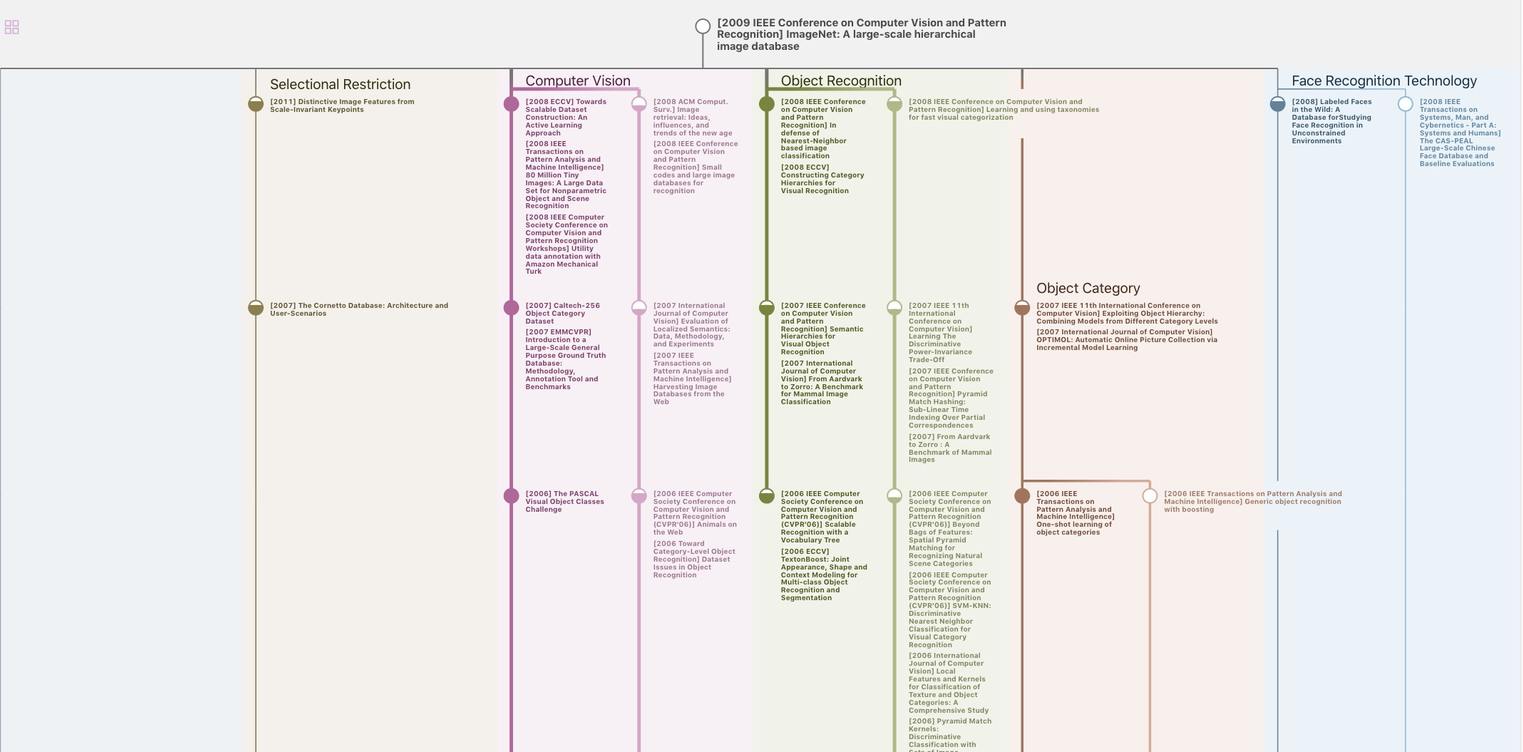
生成溯源树,研究论文发展脉络
Chat Paper
正在生成论文摘要