Fashion Outfit Composition Combining Sequential Learning And Deep Aesthetic Network
2019 INTERNATIONAL JOINT CONFERENCE ON NEURAL NETWORKS (IJCNN)(2019)
摘要
A proper outfit should consist of different categories of items that are visually compatible and share a similar style. Besides, personal aesthetic preference is also an important criterion when creating an overall outfit. Nevertheless, only few studies deal with aesthetic information in previous work on outfit composition. In this paper, we exploit both sequential learning and deep aesthetic network to train an end-to-end model for composing aesthetic outfits automatically. In detail, we firstly introduce a bidirectional long short-term memory (Bi-LSTM) model to discover the concept of compatibility among fashion items in an outfit. Then, an aesthetic-based model is proposed to parallel supervise the Bi-LSTM model so that the trained model can capture the aesthetic features from outfits. Meanwhile, we leverage visual-semantic descriptions to guarantee the uniqueness of each fashion item in an outfit. Moreover, to evaluate the effectiveness and practicability of the proposed model, we also design two representative tasks. One is evaluating the aesthetic scores of existing outfits. The other is composing aesthetic outfits conditioned on the given fashion item. Considering that aesthetic is highly correlated with personal preference, we additionally conduct the experiment that we can create proper outfits according to personal aesthetic preferences. Extensive experiments indicate that our method can generate aesthetic outfits that meet personal preferences and outperform the state-of-the-art.
更多查看译文
关键词
deep aesthetic network,personal aesthetic preference,aesthetic information,end-to-end model,aesthetic outfits,Bi-LSTM model,trained model,aesthetic features,aesthetic scores,personal preference,fashion outfit composition,fashion item,sequential learning
AI 理解论文
溯源树
样例
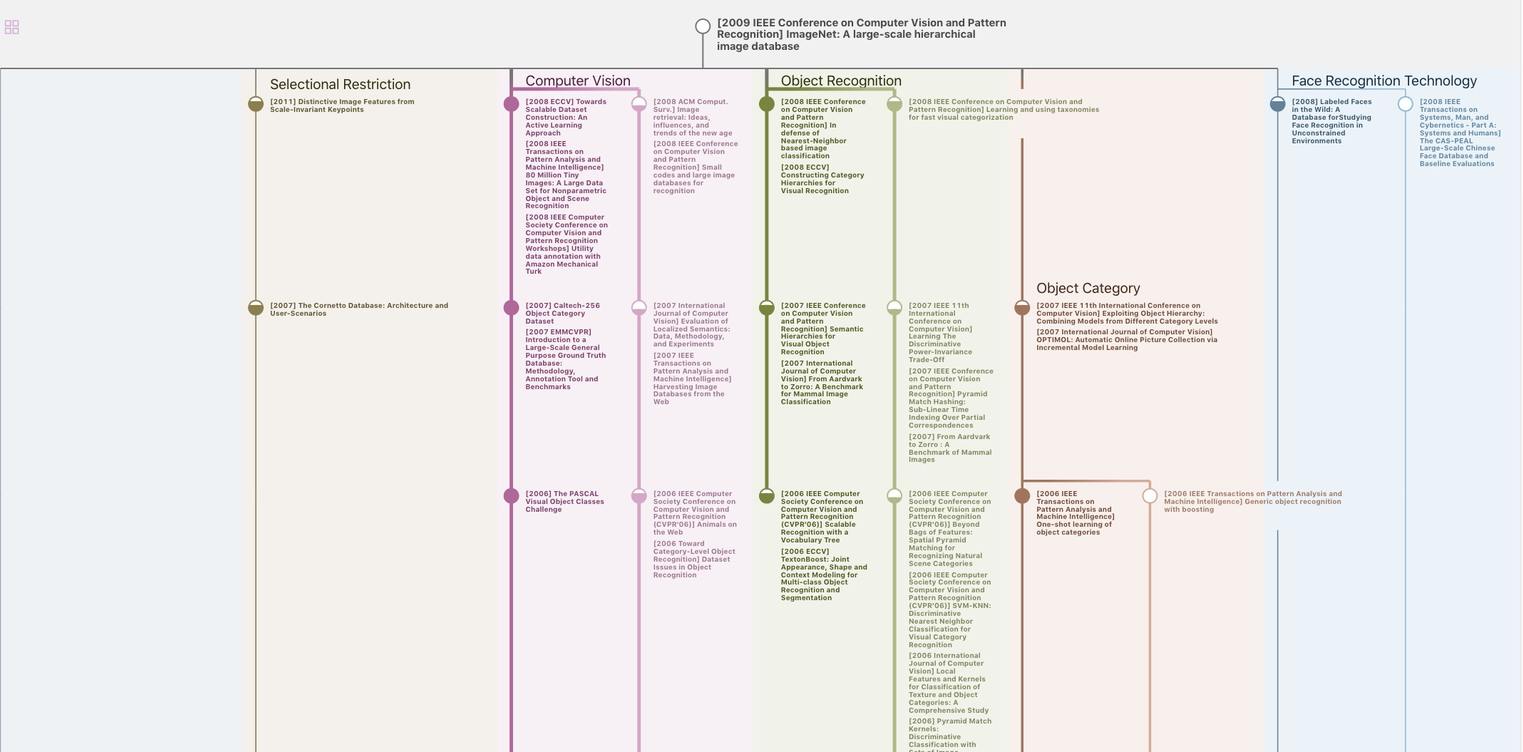
生成溯源树,研究论文发展脉络
Chat Paper
正在生成论文摘要