TH-D-213A-04: Application of Supervised Spectral Clustering for PET Tumor Delineation: A Phantom Study
MEDICAL PHYSICS(2009)
摘要
Purpose:Spectralclustering is a powerful technique for image segmentation. This study investigated the feasibility of using the supervised spectralclustering technique for PETtumor delineation. Method and Materials: A phantom was constructed with six spheres filled with 11 C featuring simulated tumors of varying size ranged from 0.5ml to 20ml situated in a cylindrical container filled with 18 F ‐FDG. The phantom was scanned for 120 minutes in a PET/CT scanner. For every 2 minute scan, 6 images were reconstructed by using the iterative OSEM algorithm with 5mm‐FWHM Gaussian smoothing filter and followed with an anisotropic filtering. The supervised spectralclustering algorithm using normalized cut was then applied to segment the filtered images.Spectralclustering uses the eigenvectors and eigenvalues of a similarity matrix (based on both proximity and intensity) to partition pixels into clusters. Its segmentation performance was evaluated with two metrics: area detection error and area overlap metric. Results: The supervised spectralclustering technique partitions the image to a designated number of regions according the measure of the pair‐wise affinities between pixels. The spectralclustering has a rather steady segmentation performance of greater than 85% area overlap for images with S/B ratios ⩾ 5. The detected area is enclosed in the true area until the S/B ratio drops to the lowest level of 2. Conclusion: The preliminary results demonstrate the potential of the supervised spectralclustering method for PETtumor segmentation. Compared with other PETtumor delineation techniques, it segments the PETimage with minimal human intervention and is instrument independent.
更多查看译文
关键词
spectral clustering
AI 理解论文
溯源树
样例
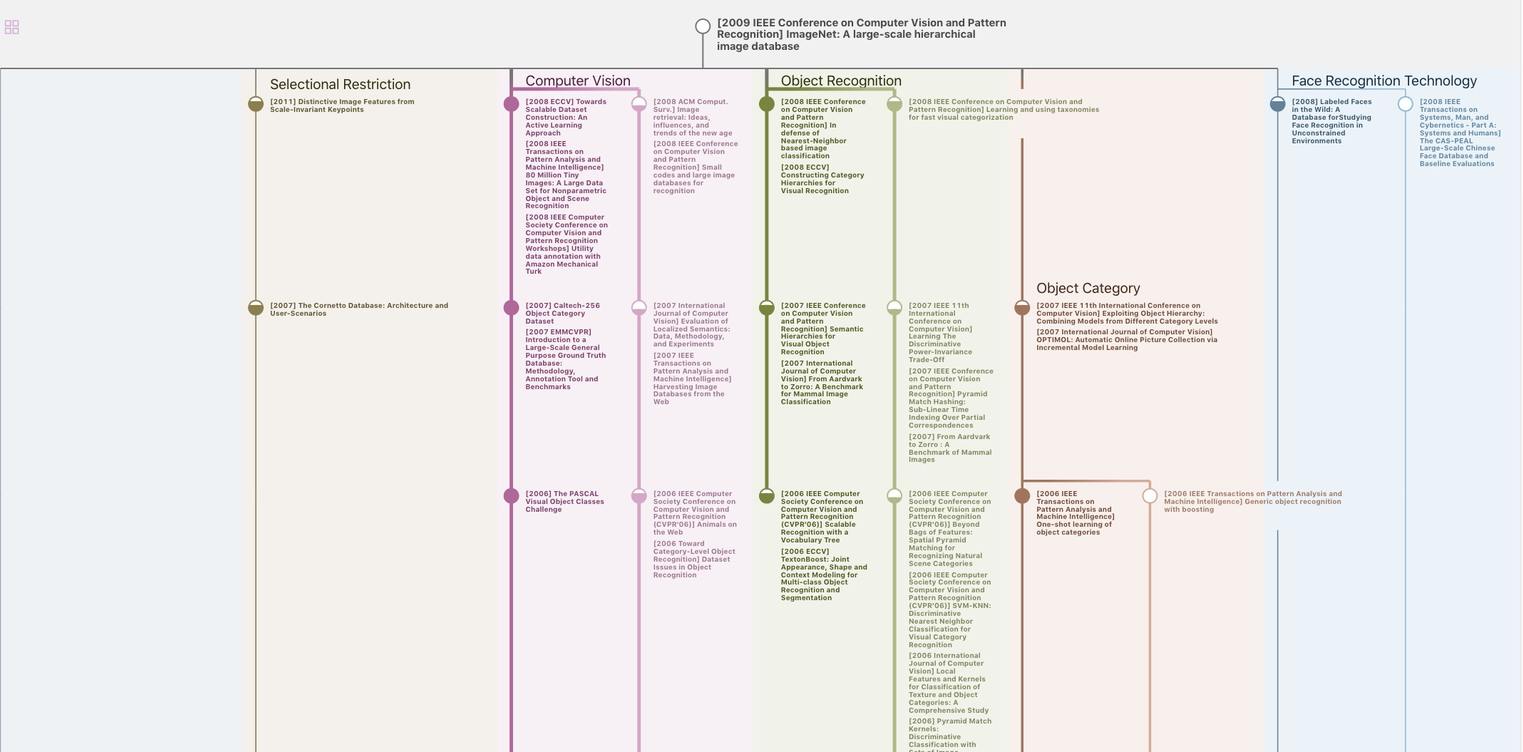
生成溯源树,研究论文发展脉络
Chat Paper
正在生成论文摘要