A Combination of Support Vector Machines and Bidirectional Recurrent Neural Networks for Protein Secondary Structure Prediction
AI(ASTERISK)IA 2003: ADVANCES IN ARTIFICIAL INTELLIGENCE, PROCEEDINGS(2003)
摘要
Predicting the secondary structure of a protein is a main topic in bioinformatics. A reliable predictor is needed by threading methods to improve the prediction of tertiary structure. Moreover, the predicted secondary structure content of a protein can be used to assign the protein to a specific folding class and thus estimate its function. We discuss here the use of support vector machines (SVMs) for the prediction of secondary structure. We show the results of a comparative experiment with a previously presented work. We measure the performances of SVMs on a significant non-redundant set of proteins. We present for the first time a direct comparison between SVMs and feed forward neural netwoks (NNs) for the task of secondary structure prediction. We exploit the use of bidirectional recurrent neural networks (BRNNs) as a filtering method to refine the predictions of the SVM classifier. Finally, we introduce a simple but effective idea to enforce constraints into secondary structure prediction based on finite-state automata (FSA) and Viterbi algorithm.
更多查看译文
关键词
recurrent neural network,secondary structure,viterbi algorithm,support vector machine
AI 理解论文
溯源树
样例
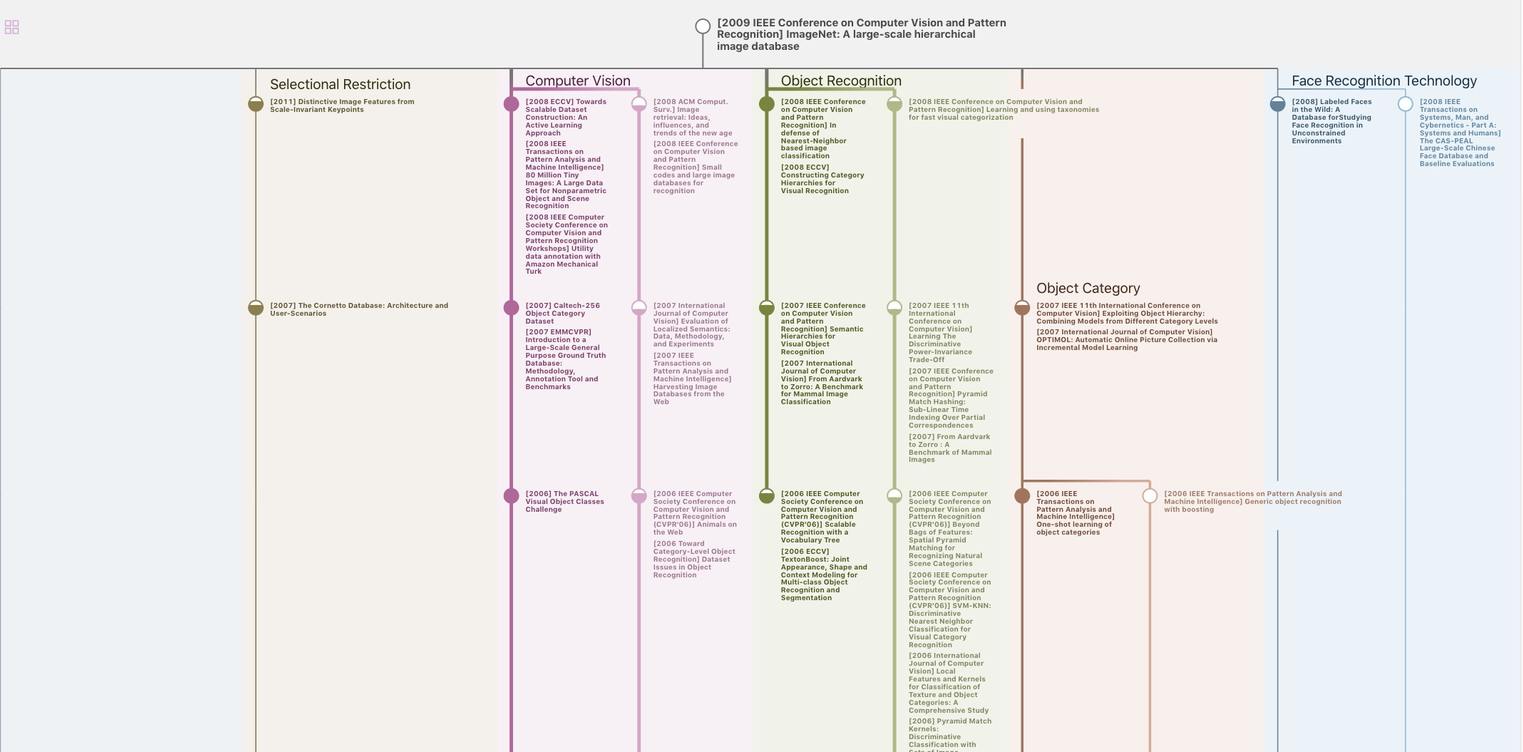
生成溯源树,研究论文发展脉络
Chat Paper
正在生成论文摘要