Knowledge-augmented Few-shot Visual Relation Detection
arxiv(2023)
摘要
Visual Relation Detection (VRD) aims to detect relationships between objects for image understanding. Most existing VRD methods rely on thousands of training samples of each relationship to achieve satisfactory performance. Some recent papers tackle this problem by few-shot learning with elaborately designed pipelines and pre-trained word vectors. However, the performance of existing few-shot VRD models is severely hampered by the poor generalization capability, as they struggle to handle the vast semantic diversity of visual relationships. Nonetheless, humans have the ability to learn new relationships with just few examples based on their knowledge. Inspired by this, we devise a knowledge-augmented, few-shot VRD framework leveraging both textual knowledge and visual relation knowledge to improve the generalization ability of few-shot VRD. The textual knowledge and visual relation knowledge are acquired from a pre-trained language model and an automatically constructed visual relation knowledge graph, respectively. We extensively validate the effectiveness of our framework. Experiments conducted on three benchmarks from the commonly used Visual Genome dataset show that our performance surpasses existing state-of-the-art models with a large improvement.
更多查看译文
关键词
relation,knowledge-augmented,few-shot
AI 理解论文
溯源树
样例
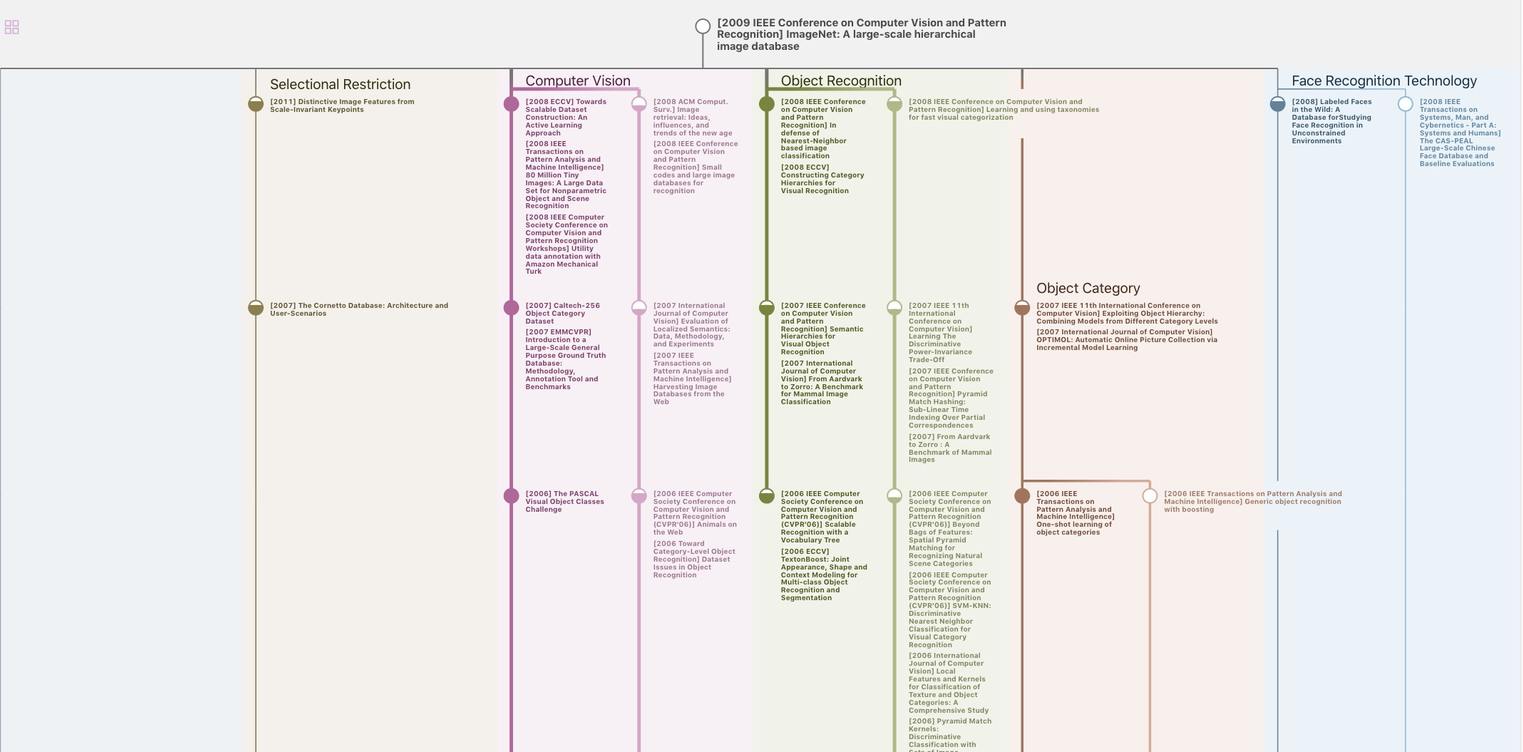
生成溯源树,研究论文发展脉络
Chat Paper
正在生成论文摘要