Incremental Structure Learning in Factored MDPs with Continuous States and Actions
msra(2009)
摘要
Learning factored transition models of structured environments has been shown to provide significant leverage when computing optimal policies for tasks within those environments. Previous work has focused on learning the structure of fac- tored Markov Decision Processes (MDPs) with finite sets of states and actions. In this work we present an algorithm for online incremental learning of transition models of factored MDPs that have continuous, multi-dimensional state and ac- tion spaces. We use incremental density estimation techniques and information- theoretic principles to learn a factored model of the transition dynamics of an FMDP online from a single, continuing trajectory of experience.
更多查看译文
AI 理解论文
溯源树
样例
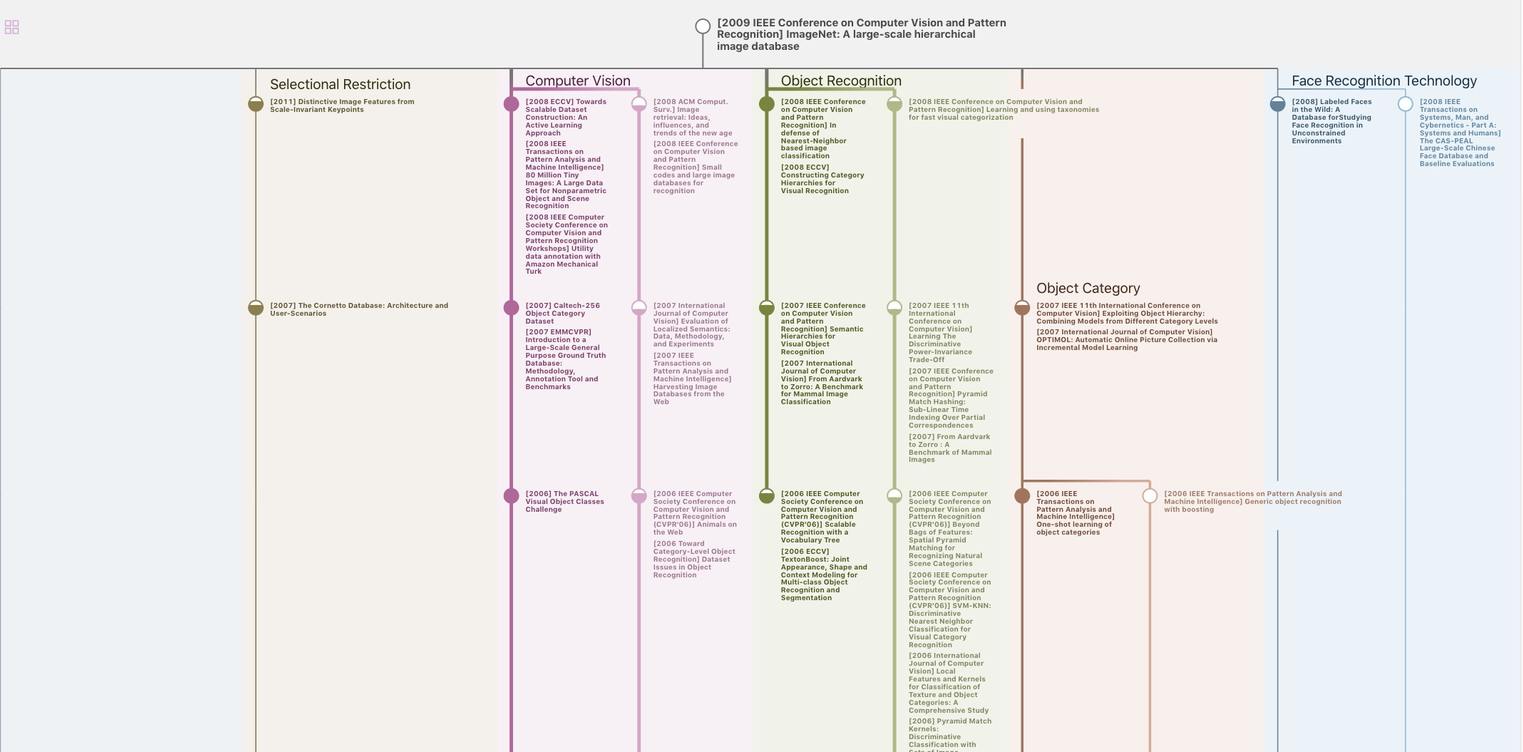
生成溯源树,研究论文发展脉络
Chat Paper
正在生成论文摘要