Blind Source Separation Using Multinode Sparse Representation
2001 INTERNATIONAL CONFERENCE ON IMAGE PROCESSING, VOL III, PROCEEDINGS(2001)
摘要
The blind source separation problem is concerned with extraction of the underlying source signals from a set of their linear mixtures, where the mixing matrix is unknown. It was discovered recently, that exploiting the sparsity of sources in their representation according to some signal dictionary, dramatically improves the quality of separation. It is especially useful in image processing problems, wherein signals possess strong spatial sparsity In this work we use the multiscale transforms, such as wavelet or wavelet packets, to decompose signals into sets of local features with various degrees of sparsity We use this intrinsic property for selecting the best (most sparse) subsets of features for further separation. Experiments with ID signals and images demonstrate significant improvement of separation quality.
更多查看译文
关键词
wavelet packets,sparse representation,surface waves,dictionaries,sparse matrices,crosstalk,feature extraction,signal generators,feature selection,independent component analysis,wavelet transforms,blind source separation,computer science,image processing,signal processing
AI 理解论文
溯源树
样例
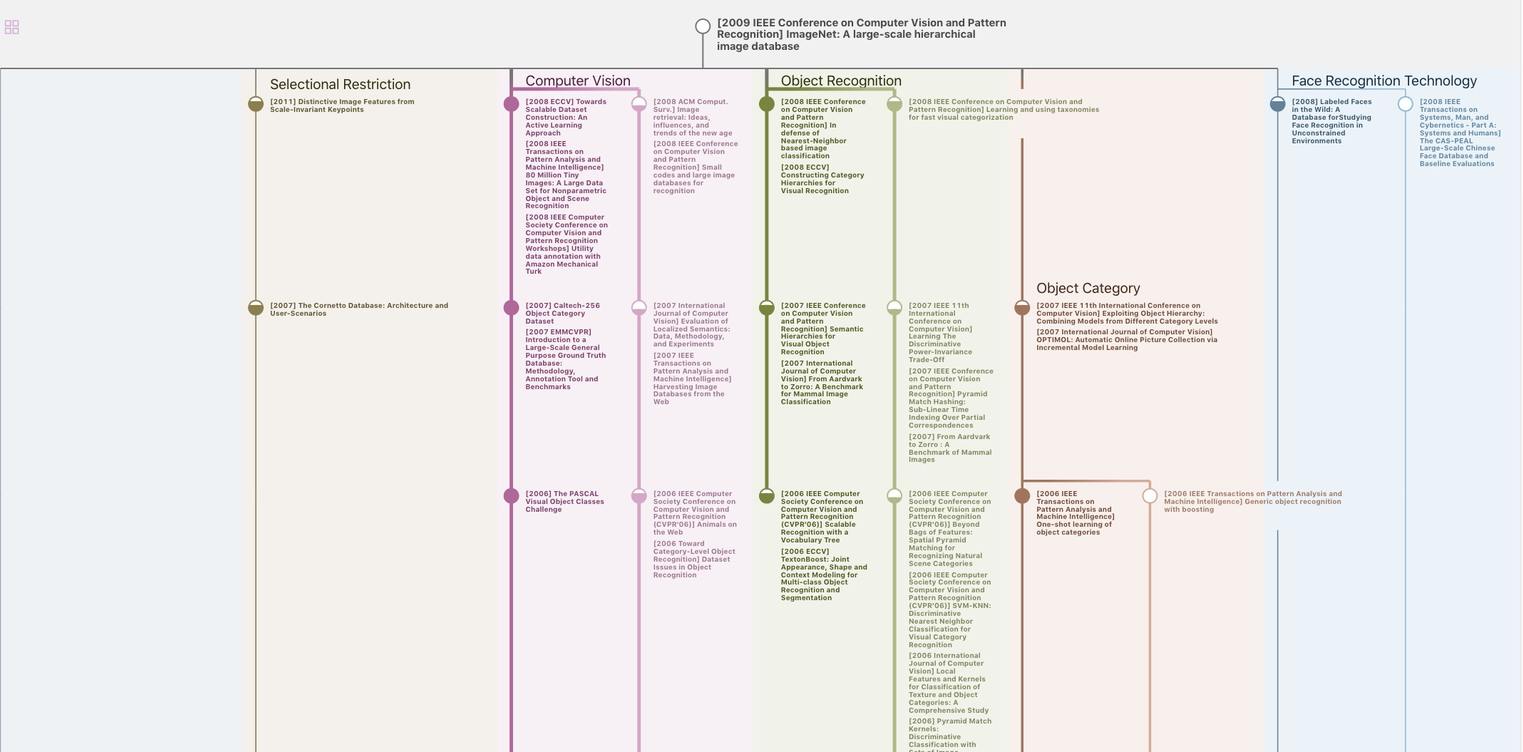
生成溯源树,研究论文发展脉络
Chat Paper
正在生成论文摘要