Addressing the metabolic activation potential of new leads in drug discovery: a case study using ion trap mass spectrometry and tritium labeling techniques.
JOURNAL OF MASS SPECTROMETRY(2003)
摘要
Metabolic activation of drug candidates to electrophilic reactive metabolites that can covalently modify cellular macromolecules may result in acute and/or idiosyncratic immune system-mediated toxicities in humans. This presents a significant potential liability for the future development of these compounds as safe therapeutic agents. We present here an example of an approach where sites of metabolic activation within a new drug candidate series were rapidly identified using online liquid chromatography/multistage mass spectrometry on an ion trap mass spectrometer. This was accomplished by trapping the reactive intermediates formed upon incubation of compounds with rat and human liver microsomes as their corresponding glutathione conjugates and mass spectral characterization of these thiol adducts. Based on the structures of the GSH adducts identified, potential sites and mechanisms of bioactivation within the chemical structure were proposed. These metabolism studies were interfaced with iterative structural modifications of the chemical series in order to block these bioactivation sites within the molecule. This strategy led to a significant reduction in the propensity of the compounds to undergo metabolic activation as evidenced by reductions in the irreversible binding of radioactivity to liver microsomal material upon incubation of tritium-labeled compounds with this in vitro system. With the efficiency and throughput achievable with such an approach, it appears feasible to identify and address the metabolic activation potential of new drug leads during routine metabolite identification studies in an early drug discovery setting. Copyright (C) 2003 John Wiley Sons, Ltd.
更多查看译文
关键词
reactive metabolites,bioactivation,glutathione conjugates,covalent binding,microsomes,ion trap mass spectrometry,tritium labeling
AI 理解论文
溯源树
样例
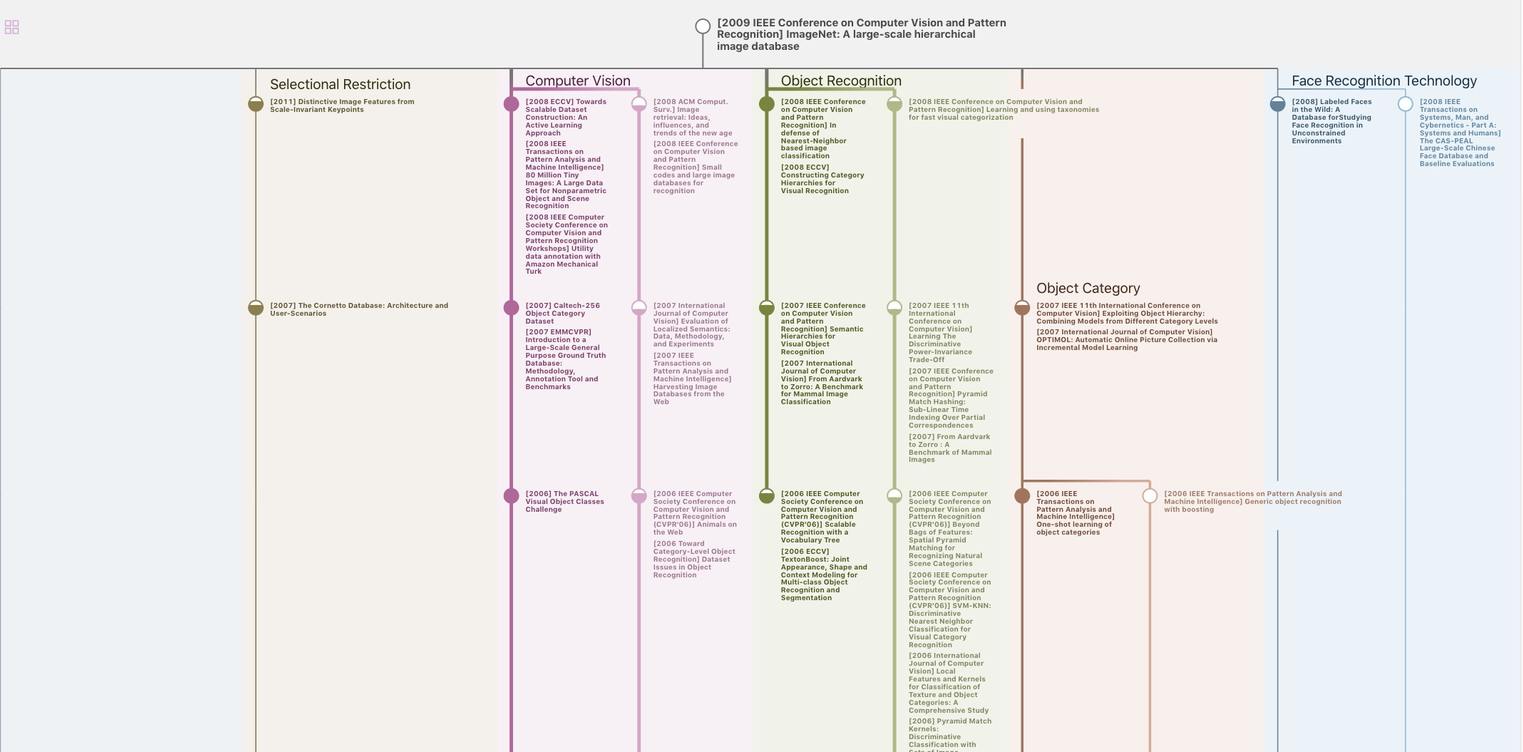
生成溯源树,研究论文发展脉络
Chat Paper
正在生成论文摘要