A graph-cut approach to image segmentation using an affinity graph based on l0–sparse representation of features
ICIP(2019)
摘要
We propose a graph-cut based image segmentation method by constructing an affinity graph using l0 sparse representation. Computing first oversegmented images, we associate with all segments, that we call superpixels, a collection of features. We find the sparse representation of each set of features over the dictionary of all features by solving a l0-minimization problem. Then, the connection information between superpixels is encoded as the non-zero representation coefficients, and the affinity of connected superpixels is derived by the corresponding representation error. This provides a l0 affinity graph that has interesting properties of long range and sparsity, and a suitable graph cut yields a segmentation. Experimental results on the BSD database demonstrate that our method provides perfectly semantic regions even with a constant segmentation number, but also that very competitive quantitative results are achieved.
更多查看译文
关键词
Image segmentation, sparse representation, l(0) affinity graph, spectral clustering
AI 理解论文
溯源树
样例
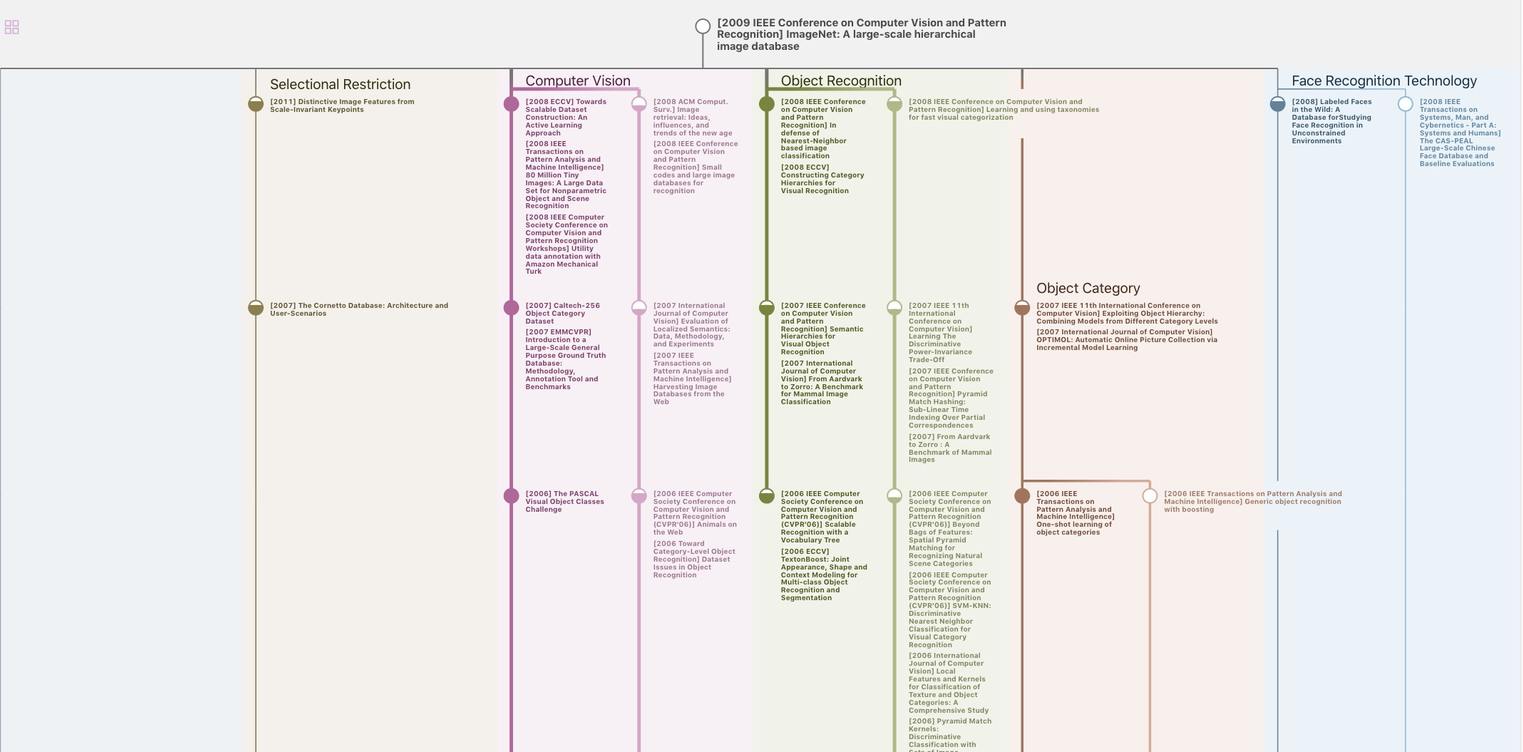
生成溯源树,研究论文发展脉络
Chat Paper
正在生成论文摘要